Artificial Intelligence tool can now detect normal chest X-rays more accurately than human radiologists with minimal errors.
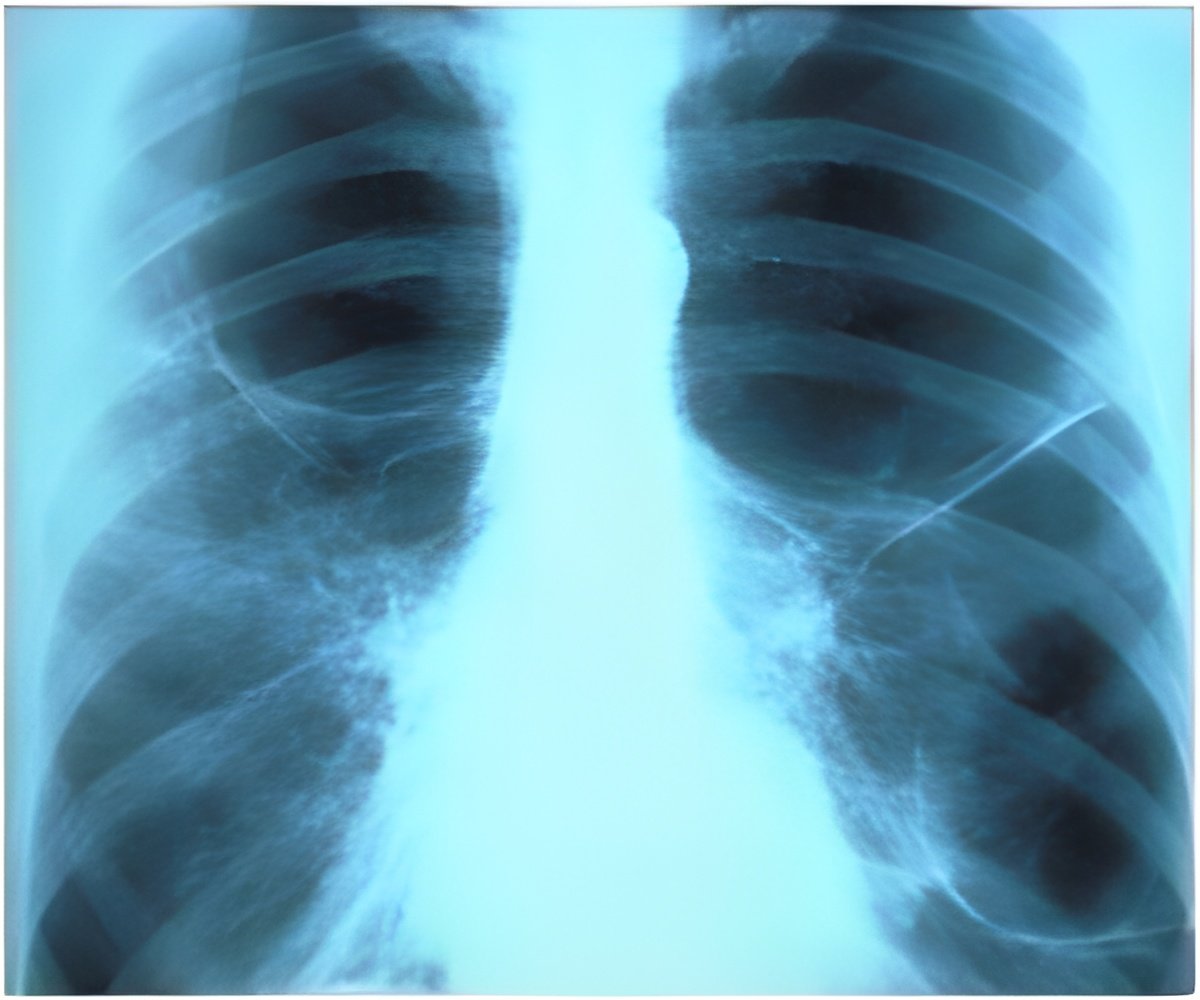
Using AI to Identify Unremarkable Chest Radiographs for Automatic Reporting
Go to source).
‘Artificial Intelligence can now handle over half of all normal chest X-rays on its own! #AI #x-ray #radiology #medindia’
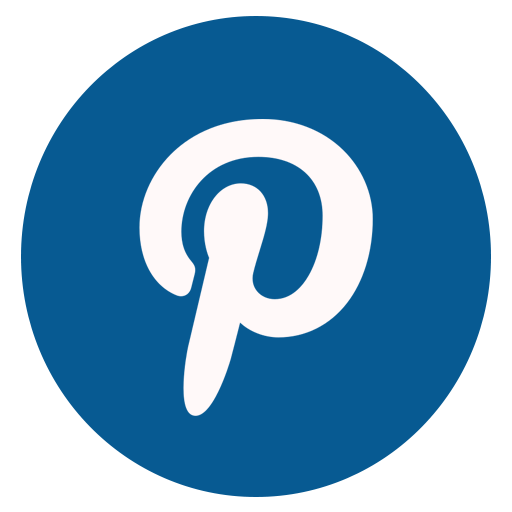
Researchers in Denmark set out to estimate the proportion of unremarkable chest X-rays where AI could correctly exclude pathology without increasing diagnostic errors. The study included radiology reports and data from 1,961 patients (median age, 72 years; 993 female), with one chest X-ray per patient, obtained from four Danish hospitals. 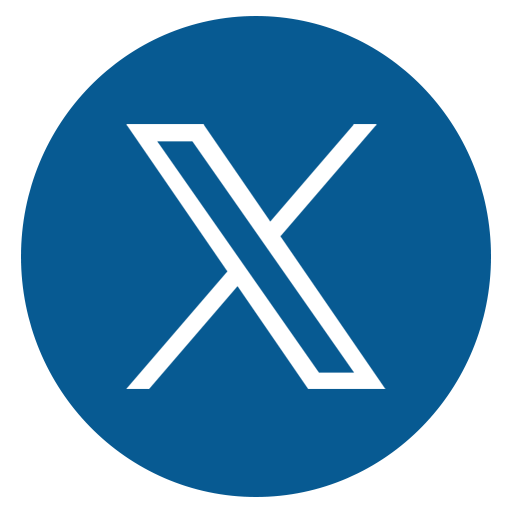
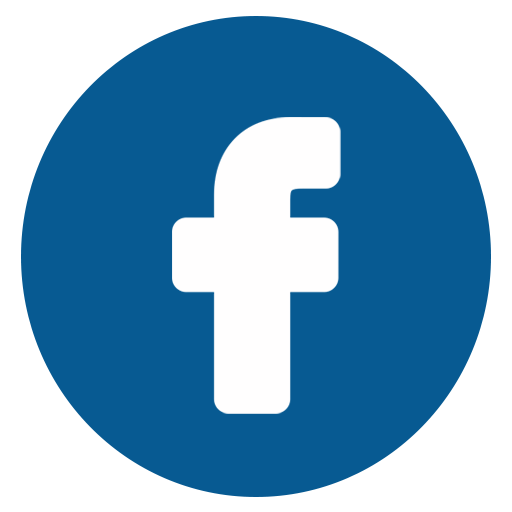
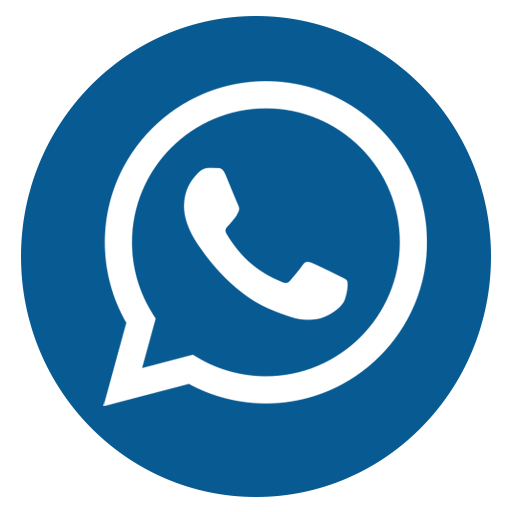
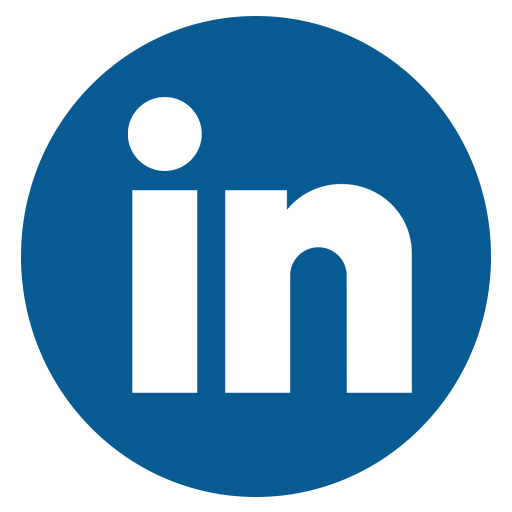
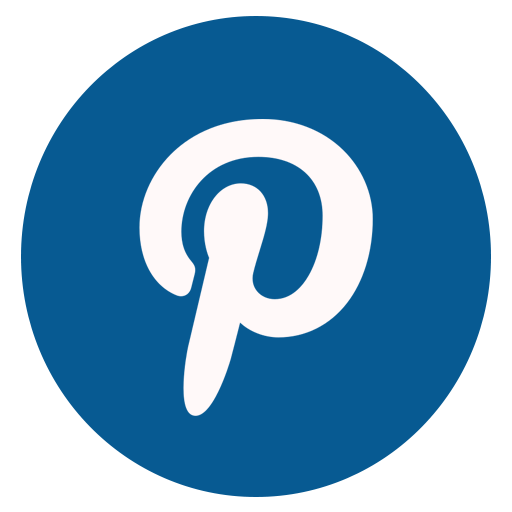
Comparison of AI and Radiologist Errors
“Our group and others have previously shown that AI tools are capable of excluding pathology in chest X-rays with high confidence and thereby provide an autonomous normal report without a human in-the-loop,” said lead author Louis Lind Plesner, M.D., from the Department of Radiology at Herlev and Gentofte Hospital in Copenhagen, Denmark. “Such AI algorithms miss very few abnormal chest radiographs. However, before our current study, we didn’t know what the appropriate threshold was for these models.”The research team wanted to know whether the quality of mistakes made by AI and radiologists was different and if AI mistakes, on average, are objectively worse than human mistakes. The AI tool was adapted to generate a chest X-ray “remarkableness” probability, which was used to calculate specificity (a measure of a medical test’s ability to correctly identify people who do not have a disease) at different AI sensitivities.
Two chest radiologists, who were blinded to the AI output, labeled the chest X-rays as “remarkable” or “unremarkable” based on predefined unremarkable findings. Chest X-rays with missed findings by AI and/or the radiology report were graded by one chest radiologist—blinded to whether the mistake was made by AI or radiologist—as critical, clinically significant, or clinically insignificant.
The reference standard labeled 1,231 of 1,961 chest X-rays (62.8%) as remarkable and 730 of 1,961 (37.2%) as unremarkable. The AI tool correctly excluded pathology in 24.5% to 52.7% of unremarkable chest X-rays at greater than or equal to 98% sensitivity, with lower rates of critical misses than found in the radiology reports associated with the images.
Advertisement
AI’s Role in Autonomous X-Ray Report
Dr. Plesner notes that the mistakes made by AI were, on average, more clinically severe for the patient than mistakes made by radiologists. “This is likely because radiologists interpret findings based on the clinical scenario, which AI does not,” he said. “Therefore, when AI is intended to provide an automated normal report, it has to be more sensitive than the radiologist to avoid decreasing the standard of care during implementation. This finding is also generally interesting in this era of AI capabilities covering multiple high-stakes environments not only limited to health care.”AI could autonomously report more than half of all normal chest X-rays, according to Dr. Plesner. “In our hospital-based study population, this meant that more than 20% of all chest X-rays could have been potentially autonomously reported using this methodology while keeping a lower rate of clinically relevant errors than the current standard,” he said. Dr. Plesner noted that a prospective implementation of the model using one of the thresholds suggested in the study is needed before widespread deployment can be recommended.
Advertisement
- Using AI to Identify Unremarkable Chest Radiographs for Automatic Reporting - (https:pubs.rsna.org/doi/10.1148/radiol.240272#:~:text=A%20commercial%20AI%20tool%20used,than%20or%20equal%20to%2095.4%25.)
Source-Eurekalert