Using machine learning AI(Artificial intelligence) model on images of everyday items improved the accuracy and speed of detecting COVID-19, reducing the need for specialist medical expertise.
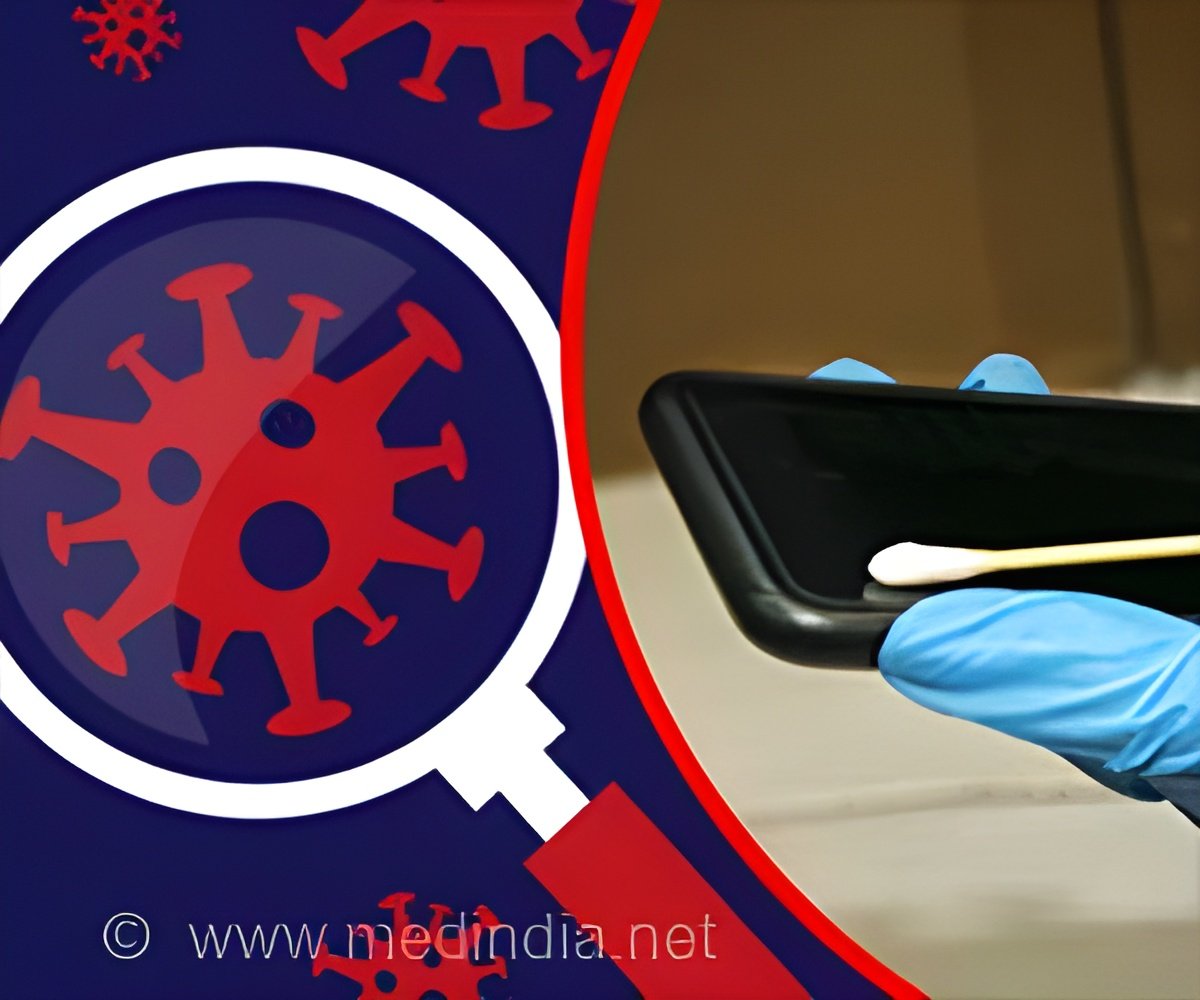
‘Researchers are using photos of toasters and fridges to train AI (Artificial intelligence) algorithms to detect COVID-19.’
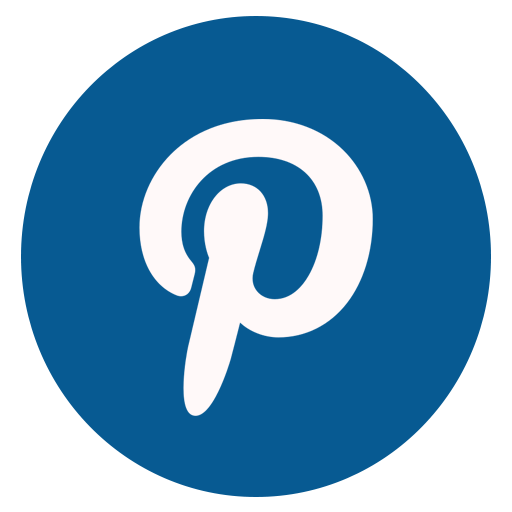
"Our technique has the capacity to not only detect COVID-19 in chest x-rays, but also other chest diseases such as pneumonia. We have tested it on 10 different chest diseases, achieving highly accurate results," said ECU School of Science researcher Dr Shams Islam.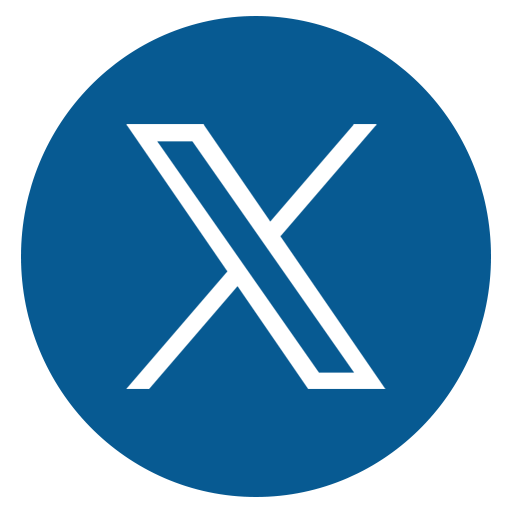
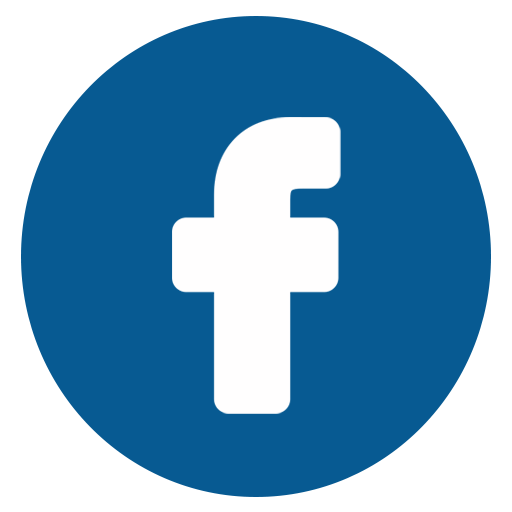
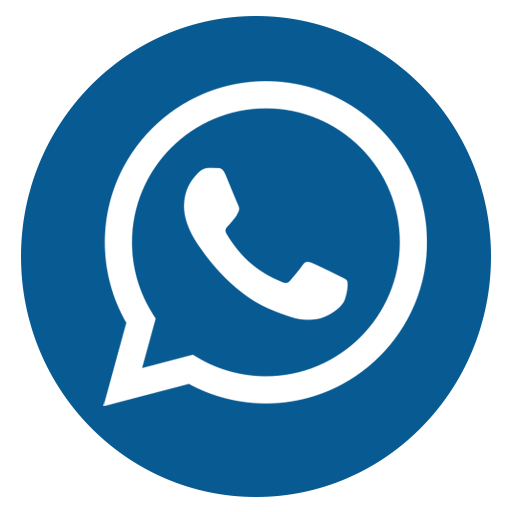
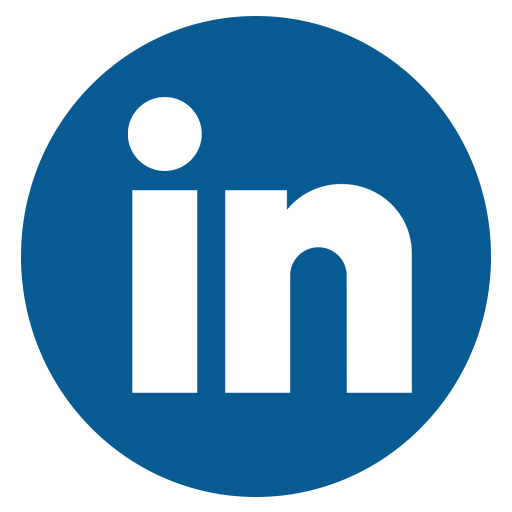
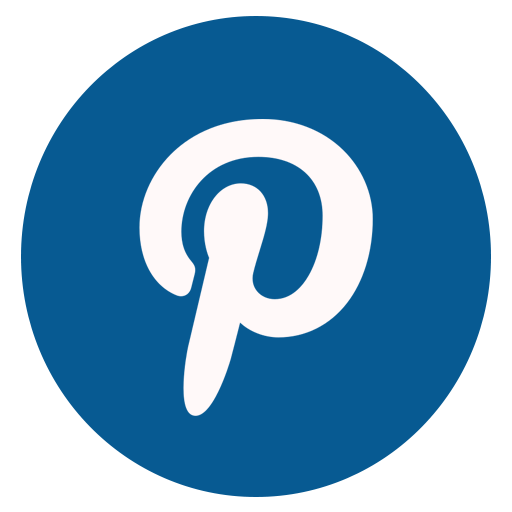
It is difficult for AI-based methods to perform detection of chest diseases accurately because the AI models need a very large amount of training data to understand the characteristic signatures of the diseases.
The data acquired needs to be carefully annotated by medical experts, it is not only a cumbersome process but also entails a significant cost.
The new technique published in Neural Computing and Applications bypasses this requirement and learns accurate models with a very limited amount of annotated data and can be used for image recognition in other medical diagnoses.
ImageNet is a database of more than 1 million images of regular household items classified by people without medical expertise just like chest x-rays diagnosed by medical professionals.
Advertisement
Researchers hope this technique can be refined in future research to increase accuracy and further reduce training time.
Advertisement
Source-Medindia