Artificial intelligence (AI) module has been found to analyze patients with intermittent atrial fibrillation using a quick and non-invasive 10-second test, unlike current tests which consume weeks to years.
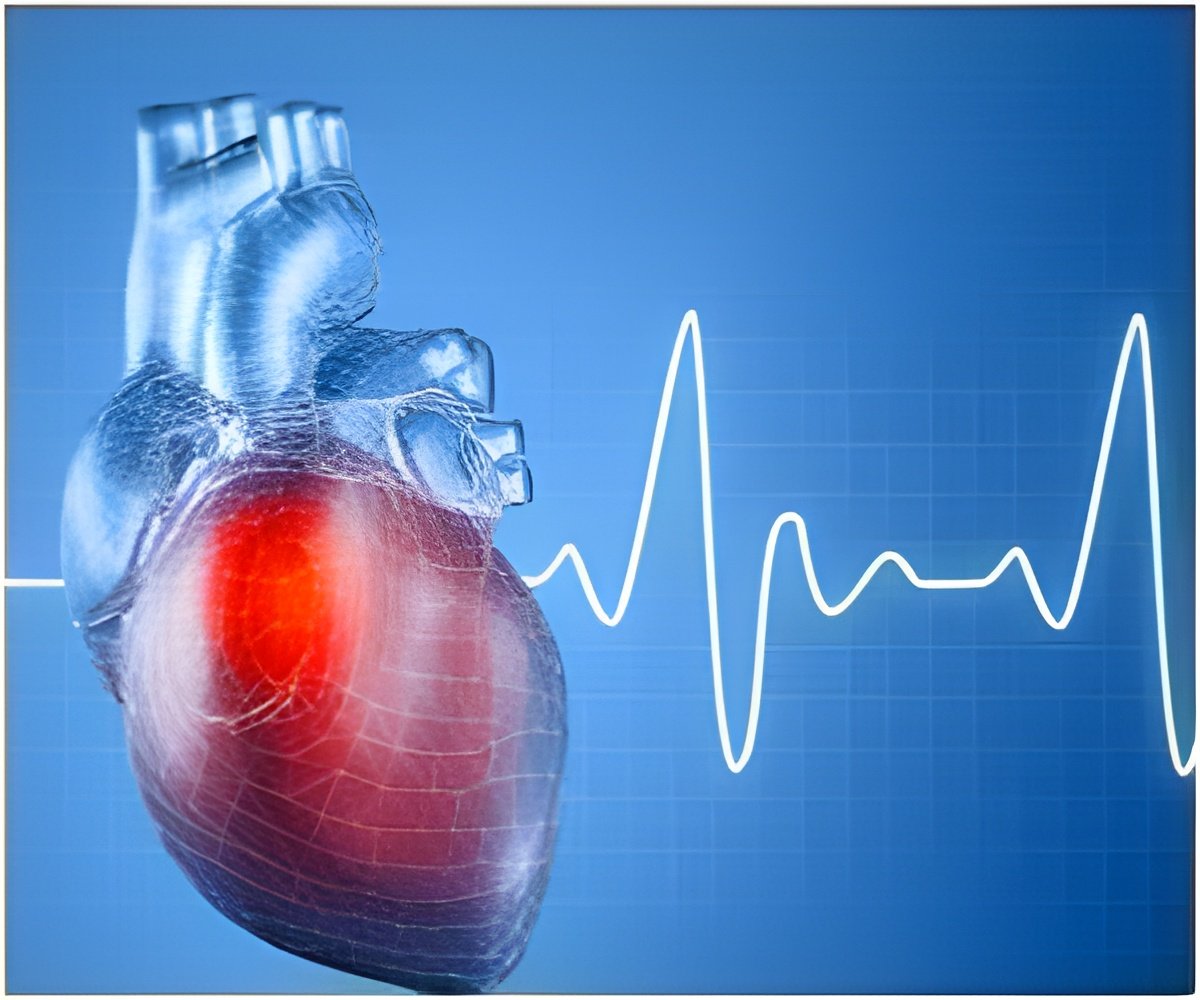
‘The Artifical intelligence has been trained using ECGs in people who needed clinical investigations, but not people with unexplained stroke nor the overall population, and so its not certain how it would perform at assessing these groups.’
Read More..
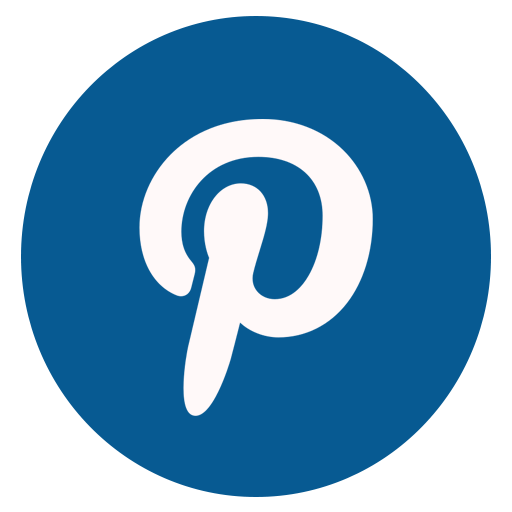
Atrial fibrillation is estimated to affect 2.7-6.1 million people in the United States [1] and is associated with increased risk of stroke, heart failure, and mortality. It is difficult to detect on a single ECG because patients' hearts can go in and out of this abnormal rhythm, so atrial fibrillation often goes undiagnosed.Read More..
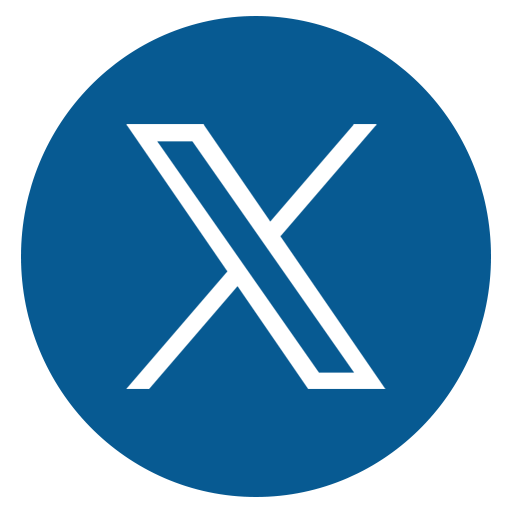
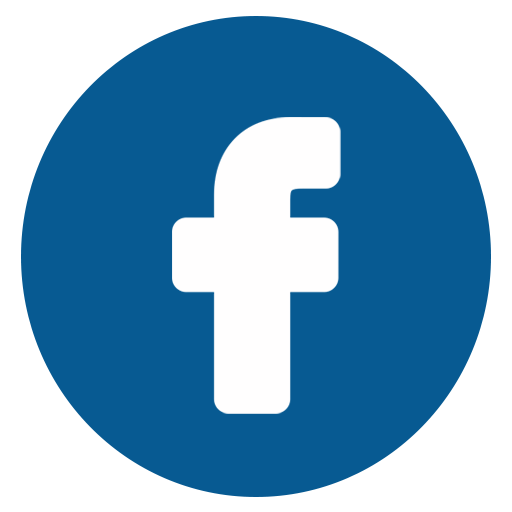
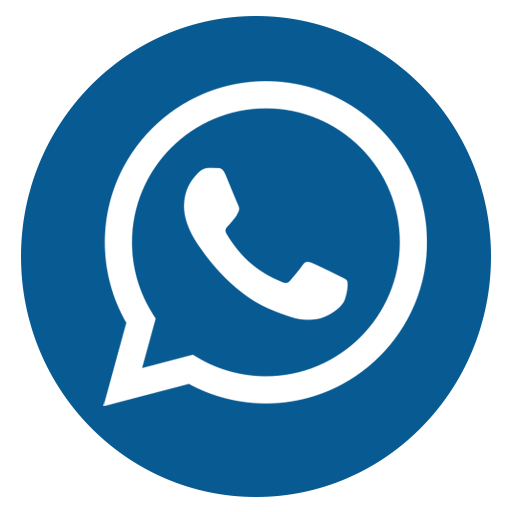
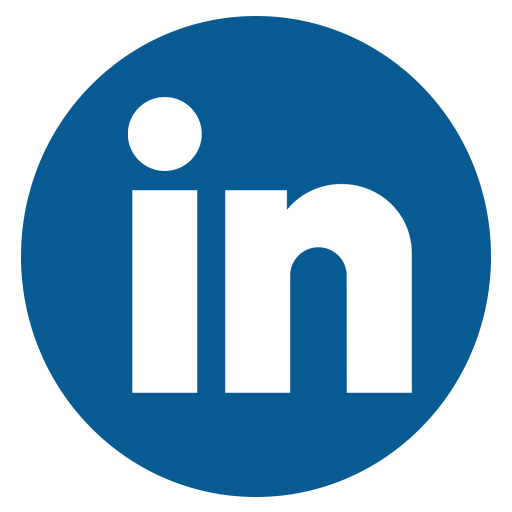
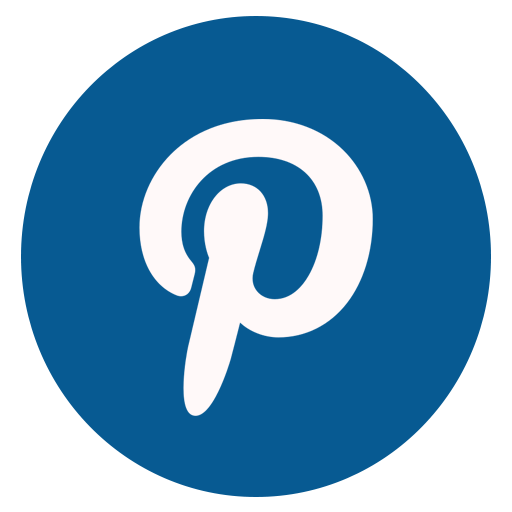
Dr. Paul Friedman, Chair of the Department of Cardiovascular Medicine, Mayo Clinic, USA says: "Applying an AI model to the ECG permits detection of atrial fibrillation even if not present at the time the ECG is recorded. It is like looking at the ocean now and being able to tell that there were big waves yesterday."
He notes: "Currently, the AI has been trained using ECGs in people who needed clinical investigations, but not people with unexplained stroke nor the overall population, and so we are not yet sure how it would perform at diagnosing these groups. However, the ability to test quickly and inexpensively with a non-invasive and widely available test might one day help identify undiagnosed atrial fibrillation and guide important treatment, preventing stroke and other serious illness." [2]
After an unexplained stroke, it is important to accurately detect atrial fibrillation so that patients with it are given anticoagulation treatment to reduce the risk of recurring stroke, and other patients (who may be harmed by this treatment) are not. Currently, detection in this situation requires monitoring for weeks to years, sometimes with an implanted device, potentially leaving patients at risk of recurrent stroke as current methods do not always accurately detect atrial fibrillation, or take too long.
Hearts with atrial fibrillation develop structural changes, such as chamber enlargement. Before those changes are visible to standard imaging techniques such as echocardiograms, there is likely fibrosis (scarring) of the heart associated with atrial fibrillation. Additionally, the presence of atrial fibrillation may temporarily modify the electrical properties of the heart muscle, even after it has ended.
Advertisement
ECG data was assigned into three groups: training, internal validation and testing datasets with 70% in the training group, 10% invalidation and optimisation, and 20% in the testing group (454,789 ECGs from 126,526 patients in the training dataset, 64,340 ECGs from 18,116 patients in the validation dataset and 130,802 ECGs from 36,280 patients in the testing dataset).
Advertisement
The authors of the study also speculate that it may one day be possible to use this technology as a point-of-care diagnostic test in the doctor's surgery to screen high-risk groups. Screening people with hypertension, diabetes, or age over 65 years for atrial fibrillation could help avoid ill health. However, current detection methods are costly and identify a few patients. In addition, this screening currently requires wearing a large and uncomfortable heart monitor for days or weeks.
Dr. Xiaoxi Yao, a study co-investigator from Mayo Clinic, USA, says: "It is possible that our algorithm could be used on low-cost, widely available technologies, including smartphones, however, this will require more research before widespread application." [2]
The authors note several limitations and further research before their work reaches clinics. The population studied may have a higher prevalence of atrial fibrillation compared to the general population. The AI has therefore been trained to retrospectively classify clinically indicated ECGs more than for prediction in healthy patients, or those with unexplained stroke, and may need calibration before widespread application to screening of a broader, healthy population.
Patients were considered negative for atrial fibrillation if they did not have verified the diagnosis, but there were likely some patients who had been undiagnosed and labeled erroneously, so the AI may have identified what the previous testing had not. On the other hand, some of the false-positive patients identified by the AI as having a history of atrial fibrillation (despite being classified as negative by a human) might actually have had undiagnosed atrial fibrillation. Since the AI is only as good as the data, it is trained against there could be errors in the interpretation when the test is applied to other populations, such as an individual without an indicated ECG.
In a linked Comment, Dr. Jeroen Hendriks of the University of Adelaide and Royal Adelaide Hospital, Adelaide, Australia, says: "In summary, Attia and colleagues are to be congratulated for their innovative approach and the thorough development and local validation of the AI-enabled ECG. Given that AI algorithms have recently reached cardiologist level in diagnostic performance, this AI-ECG interpretation is ground-breaking in creating an algorithm to reveal the likelihood of atrial fibrillation in ECGs showing sinus rhythm."
Source-Eurekalert