Study shows that machine learning model is superior to traditional behavioral diagnosis for ADHD.
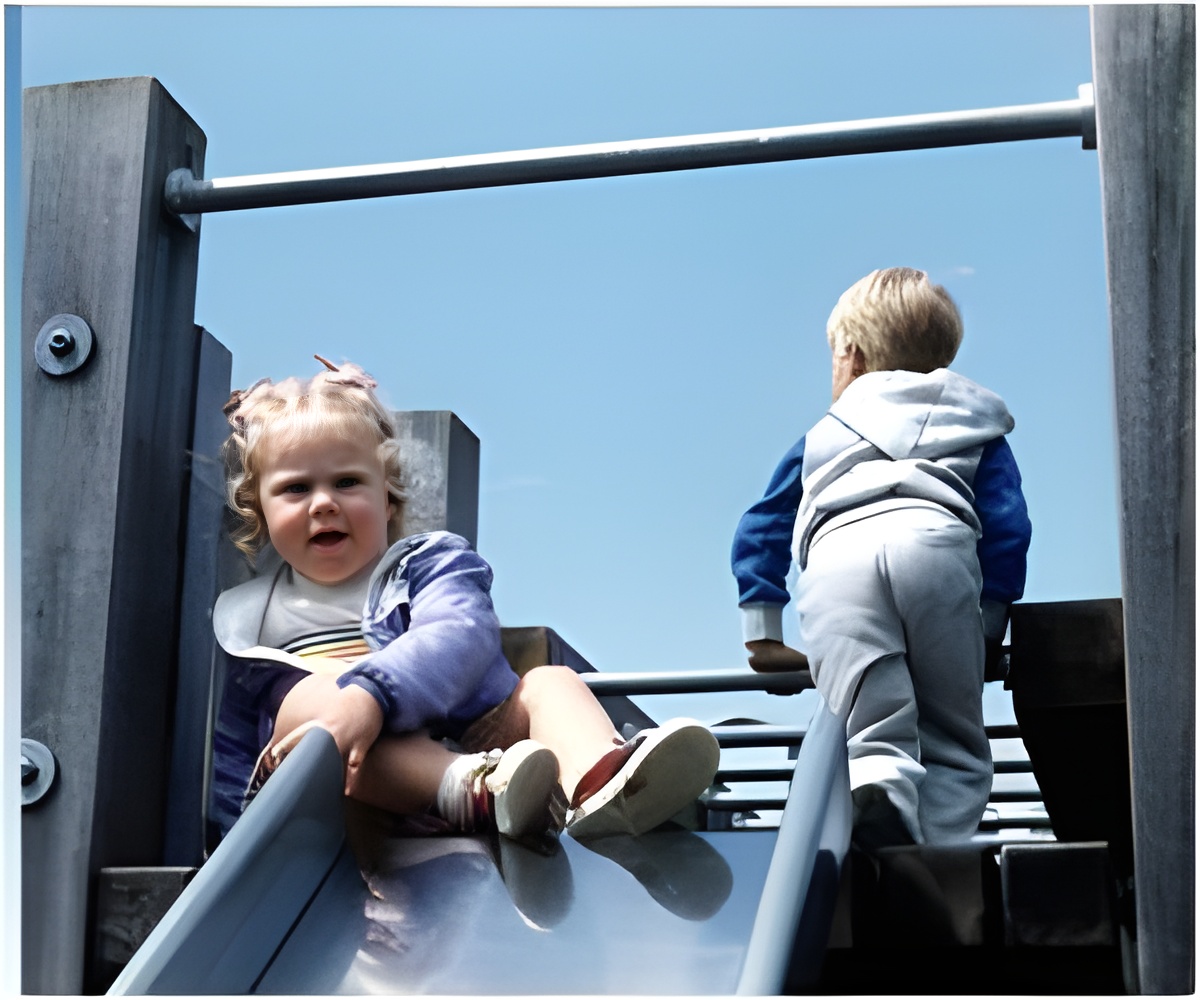
The research used machine-learning classifiers to identify adults who had received a childhood diagnosis of ADHD with 99% accuracy.
Lead author of the study Chris McNorgan explains that the results suggest that brain connectivity is a stable biomarker for ADHD, at least into childhood. This is true even when an individual's behavior becomes more typical sometimes by adapting different coping strategies.
Since patients sit on a broad-spanning continuum, findings can also help tailor-make treatment plans for the patients. McNorgan also adds that as certain medical drugs react with certain pathways, understanding the different types of ADHD can help make informed decisions about one medication versus others.
ADHD has multiple subtypes, which complicate a clinical definition. The diagnosis of ADHD in a patient can change when that same patient returns for a follow-up evaluation.
"A patient may be exhibiting behavioral symptoms consistent with ADHD one day, but even days later, might not present those symptoms, or to the same degree," said McNorgan. "It could just be the difference between a good day and a bad day. But the brain connectivity signature of ADHD appears to be more stable. We don't see the diagnostic flip-flop."
The research team used fMRI data from 80 adult participants who were diagnosed with ADHD during childhood.
During a task designed to test the subject's ability to inhibit an automatic response, machine learning classifiers were then applied to four snapshots taken during the activity.
ADHD was predicted from the patterns of communication between groups of brain areas, for example, A, B, and C. If regions A and B were highly connected, that could be predictive of ADHD, but not if these regions were also highly connected to the region C. These relationships are problematic for commonly-used techniques, but not for deep-learning classifiers.
This study also differentiates individuals with ADHD with typical or atypical performance on the Iowa Gambling Task (IGT) - a behavioral paradigm that presents both high and low-risk options, commonly used to study and diagnose ADHD.
This method also gives room for developing therapies that focus on specific brain networks, he concluded.
Source-Medindia