A team of scientists has developed a computer model that applies techniques used to analyse social networks to identify new ways of treating cancer.
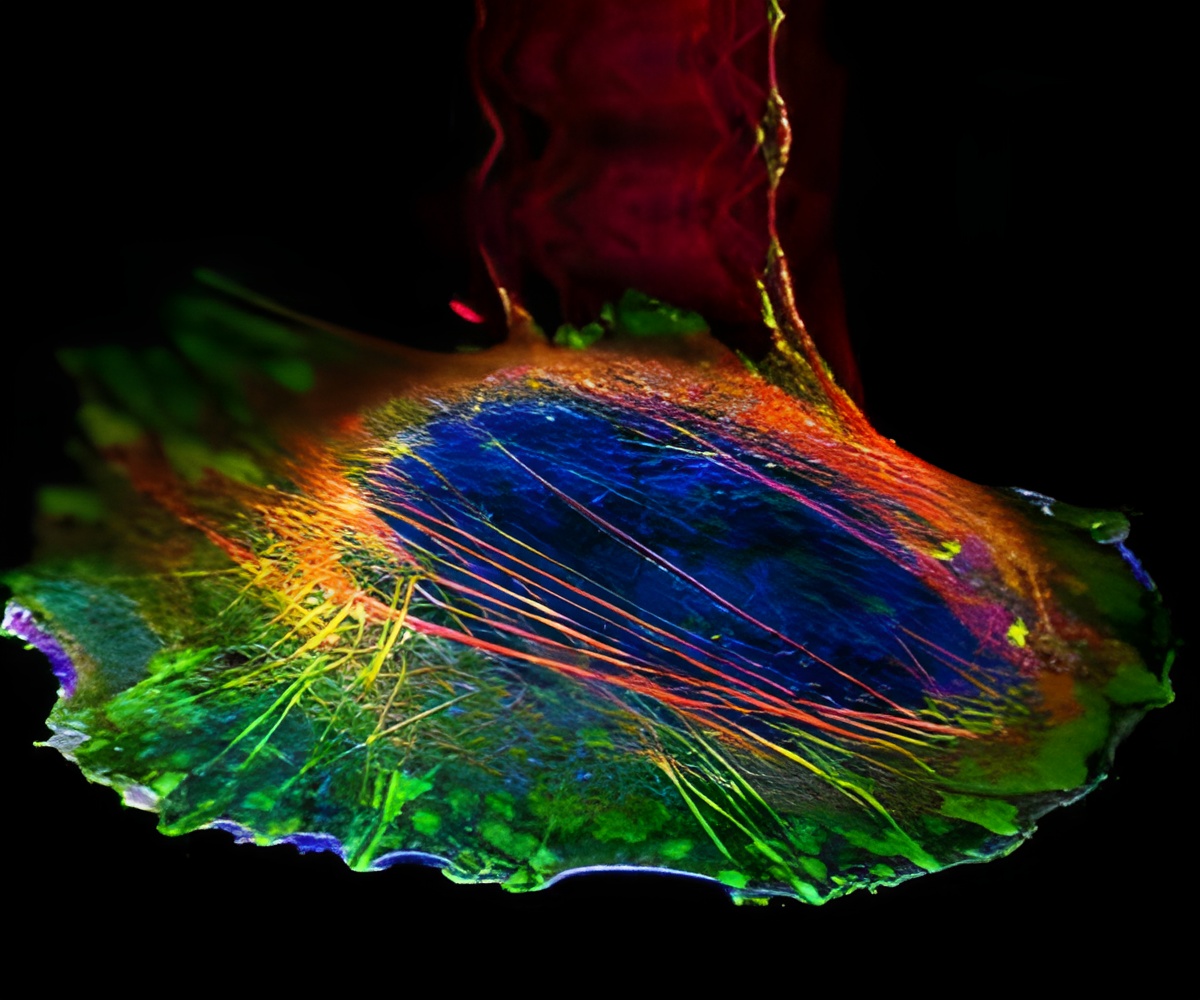
‘Cancer-causing proteins tended to have particular 'social' characteristics that differ from non-cancer proteins. This suggests that previously unexplored cancer proteins with similar characteristics could also make good drug targets.’
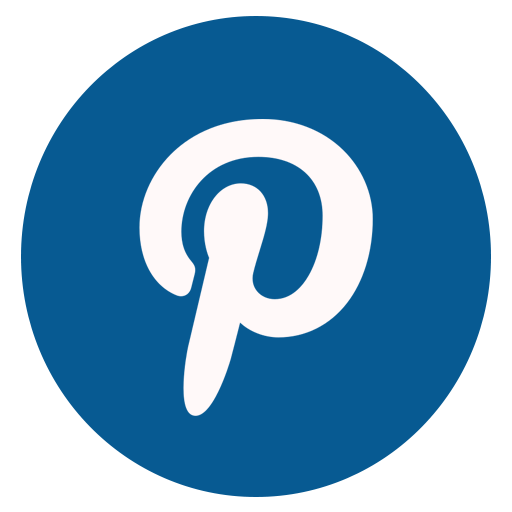
The researchers have made this map publicly available. It could provide drug discovery scientists with a shortcut to finding new drugs for many different types of cancer. The team found that there are many molecular pathways that interact to affect the development of cancer.
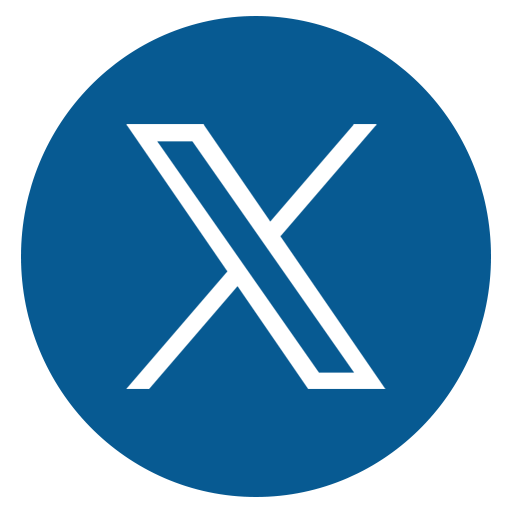
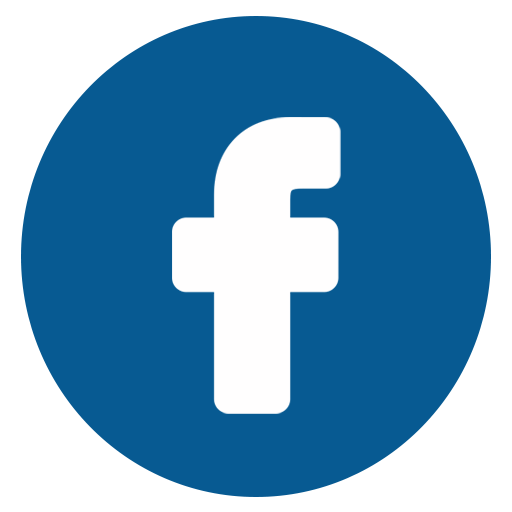
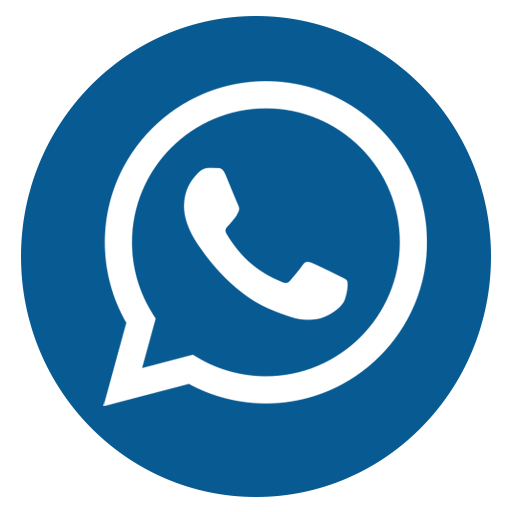
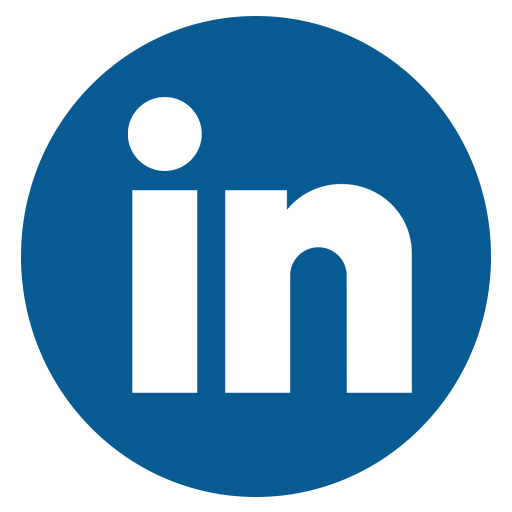
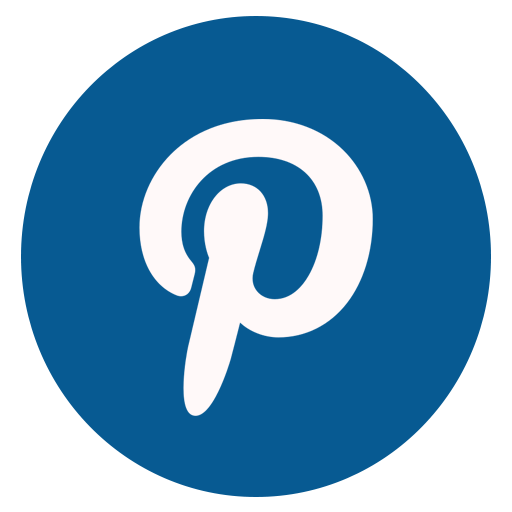
Cancer-causing proteins, which have already been successfully targeted with drugs, tended to have particular 'social' characteristics that differ from non-cancer proteins, suggesting that previously unexplored cancer proteins with similar characteristics could also make good drug targets. 'Hub-like' proteins which 'communicate' with lots of other proteins - like a super-Facebook user with thousands of friends - were more likely to cause cancer.
Study leader Bissan Al-Lazikani said, "This map will help researchers design better new drugs, more quickly, saving time and money. It also sheds light on how resistance to treatments may occur, and in just a few years could help doctors choose the best drug combinations to suit individual patients."
The study is published in PLOS Computational Biology.
Source-ANI