Deep learning, a sophisticated type of AI in which the computer can be trained to recognize subtle patterns, has the potential to improve chest X-ray interpretation, said scientists.
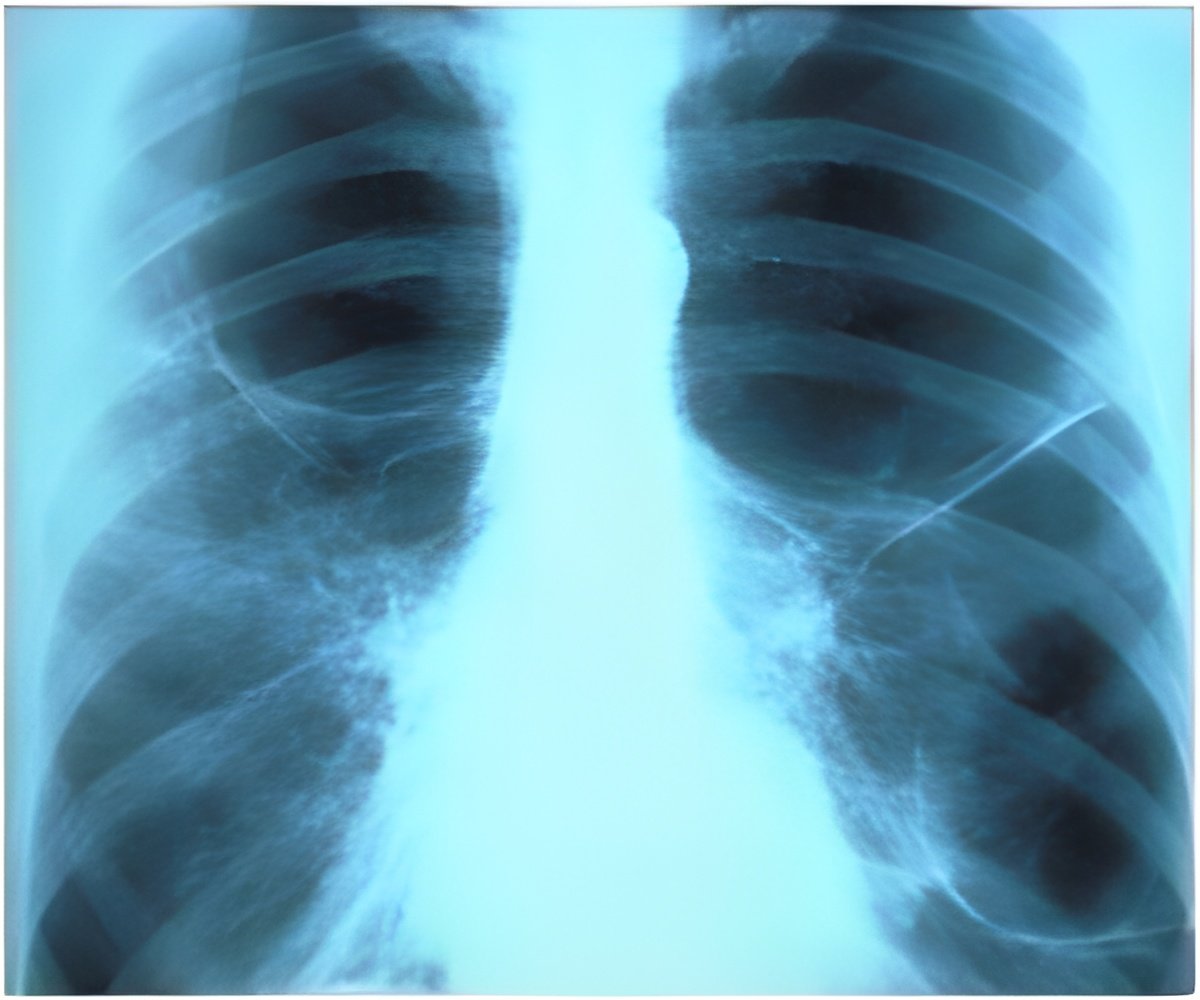
‘Deep learning models for chest X-ray interpretation have been developed by Google Health researchers.’
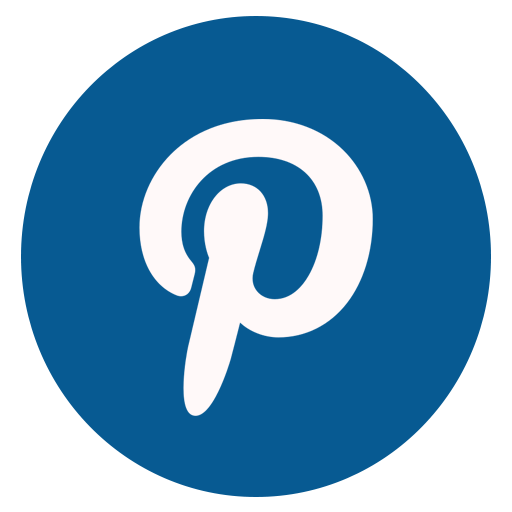
"We've found that there is a lot of subjectivity in chest X-ray interpretation," said study co-author Shravya Shetty, an engineering lead at Google Health in Palo Alto, California. "Significant inter-reader variability and suboptimal sensitivity for the detection of important clinical findings can limit its effectiveness." 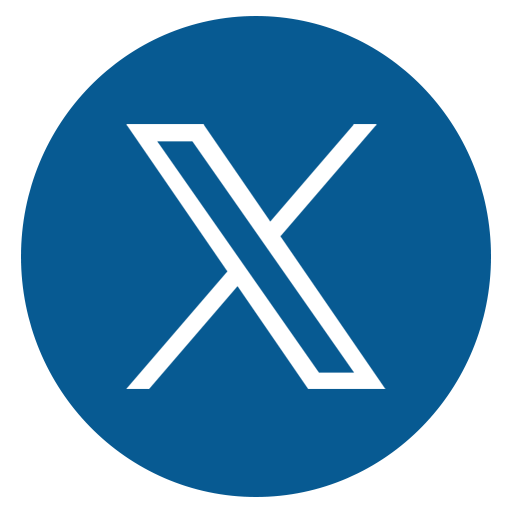
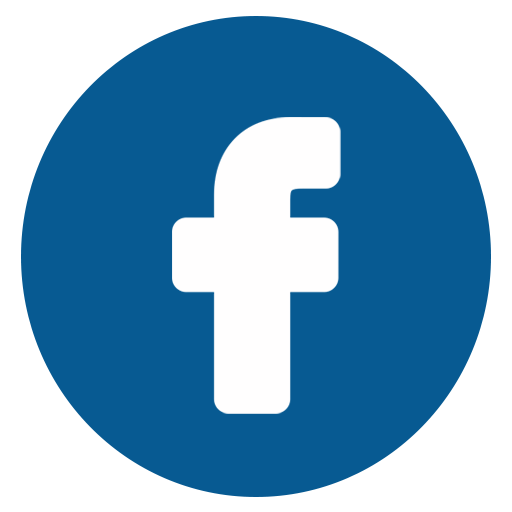
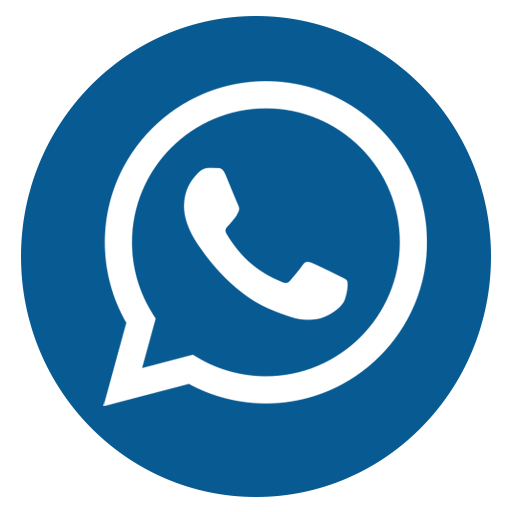
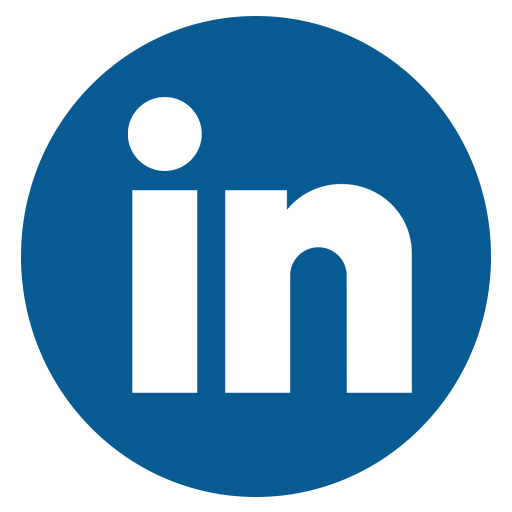
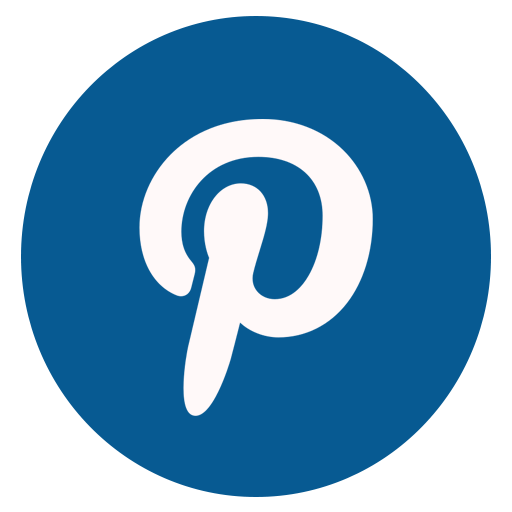
Deep learning, a sophisticated type of AI in which the computer can be trained to recognize subtle patterns, has the potential to improve chest X-ray interpretation, but it too has limitations. For instance, results derived from one group of patients cannot always be generalized to the population at large.
They used two large datasets to develop, train and test the models. The first dataset consisted of more than 750,000 images from five hospitals in India, while the second set included 112,120 images made publicly available by the National Institutes of Health (NIH).
A panel of radiologists convened to create the reference standards for certain abnormalities visible on chest X-rays used to train the models.
"Chest X-ray interpretation is often a qualitative assessment, which is problematic from deep learning standpoint," said Daniel Tse, M.D., product manager at Google Health. "By using a large, diverse set of chest X-ray data and panel-based adjudication, we were able to produce more reliable evaluation for the models."
Advertisement
Radiologist adjudication led to increased expert consensus of the labels used for model tuning and performance evaluation. The overall consensus increased from just over 41 percent after the initial read to more than almost 97 percent after adjudication.
"We believe the data sampling used in this work helps to more accurately represent the incidence for these conditions," Dr. Tse said. "Moving forward, deep learning can provide a useful resource to facilitate the continued development of clinically useful AI models for chest radiography." The research team has made the expert-adjudicated labels for thousands of NIH images available for use by other researchers at the following link:
https://cloud.google.com/healthcare/docs/resources/public-datasets/nih-chest#additional_labels. "The NIH database is a very important resource, but the current labels are noisy, and this makes it hard to interpret the results published on this data," Shetty said. "We hope that the release of our labels will help further research in this field."
Source-Eurekalert