Artificial intelligence (AI) has shown why humans look older or younger. The PhotoAgeClock, based on AI technology can predict the age of a person by analyzing images of the skin at the corner of the eyes.
- An artificial intelligence (AI)-based technology called the PhotoAgeClock is capable of analyzing high-resolution images of the eyes, particularly the skin around the eyes
- The images may show wrinkles at the corner of the eyes and the extent of wrinkling can help predict the age of the person
- This AI-based technology is ideal for age prediction due to its non-invasive nature, ability to analyze anonymized data and high accuracy
In this regard, Anastasia Georgievskaya, CEO of Haut.AI, said: “We are very happy to collaborate with one of the most innovative, global, and ethical consumer companies committed to benefit consumers around the world on this important artificial intelligence project. The future of consumer business is in personalization and I hope that this study will lay the foundation for AI-powered consumer skincare and healthcare. Skin is our largest and one of the most important organs. Understanding the many biological processes in skin using AI may lead to the many breakthroughs down the road.”
The research findings have been published in Aging (Albany NY), a leading journal in the area of aging research.
Read More..
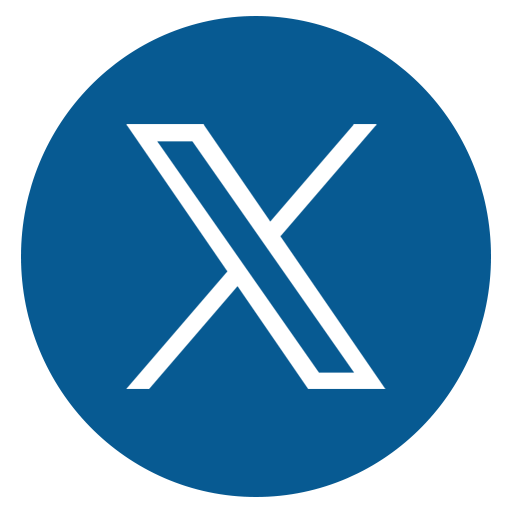
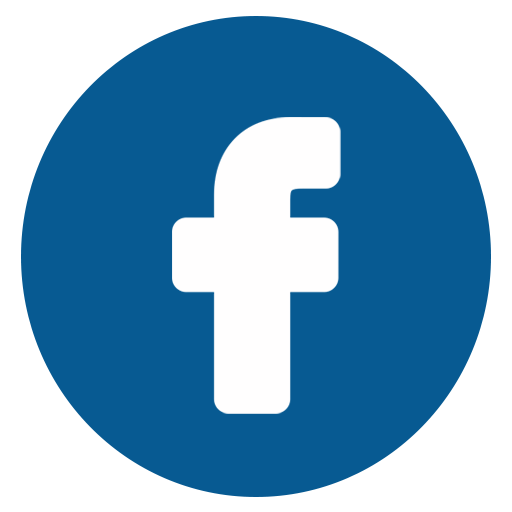
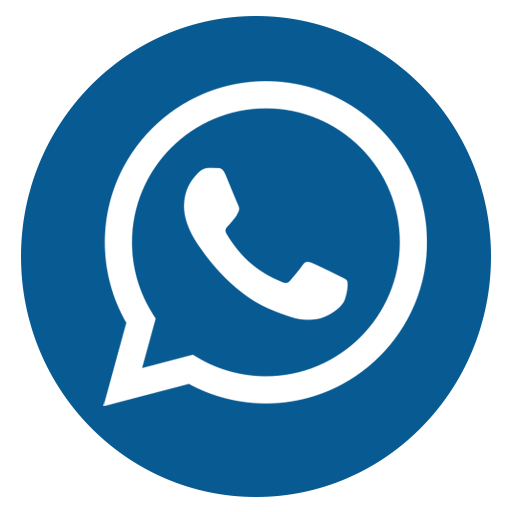
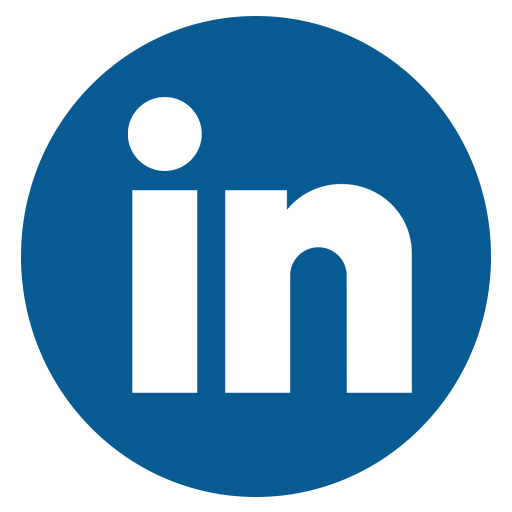
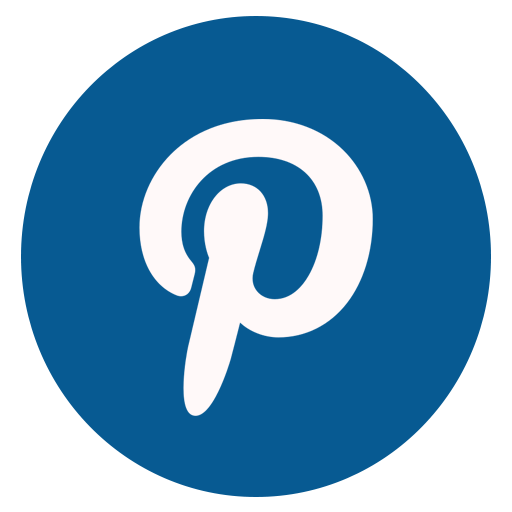
What is Artificial Intelligence (AI)?
Artificial intelligence (AI), also called machine intelligence, is intelligence exhibited by machines, as opposed to natural intelligence displayed by human beings. AI involves simulation of processes involved in human intelligence by machines, particularly computers. AI involves the following processes:- Learning: The acquisition of information and learning how to use the information by following certain predefined rules.
- Reasoning: This involves interpretation of the accumulated information to reach an approximate or definitive conclusion by following a set of rules.
- Auto-correction: This involves checking and re-checking the data and automatically correcting any inconsistencies in the previous processes i.e. learning and reasoning so that the final result is absolutely correct and flawless.
Study Design
The study consisted of 8,414 anonymized high-resolution photos of the left and right eye corners of Caucasian females, along with the actual chronological age indicated on each photo. The age of the individuals ranged between 20-80 years. The age distribution was uneven – the age range of 40-65 years was higher than the other age groups in the study.In order to find the areas most sensitive for the age changes, the eyes were blocked with black spots of various sizes. The eye corner and eyelid areas were the most accurate in predicting the age. When blocked, the absence of these areas produced the highest relative error. This observation showed that the wrinkles under the eyes were the most important features for age prediction, indicating that these areas could be used for developing photographic biomarkers of aging.
Study Findings
The study findings indicate that high-resolution information about eye corner wrinkles can be utilized for accurately predicting the chronological age of a person.The ability of the PhotoAgeClock to predict the age is much more accurate in the age group 40-65 years than the age groups 20-40 years and above 65 years. This difference could be due to the fact that the latter two age groups were represented to a lesser extent in the dataset.
The predictive accuracy of the PhotoAgeClock was measured on the basis of the mean average error (MAE) and other statistical parameters. MAE is the difference between the age predicted by the PhotoAgeClock and the actual chronological age, which in this case was 2.3 years. Therefore, the PhotoAgeClock is ideal for age prediction due to its non-invasive nature, ability to analyze anonymized data and high accuracy.
Study Implications
The study has the following important implications:- Accurate estimation of age from facial images should use high-resolution images
- Accuracy of age prediction does not decrease significantly when the eye area is blocked
- PhotoAgeClock can be utilized to predict age on anonymized datasets of images
- Wrinkles and skin pigmentation serve as reliable non-invasive visual biomarkers of aging, which can provide valuable insight into the health status of the individual
- Personalized medical, lifestyle and cosmetic interventions for aging could be developed, based on individual health status, skin type, climate, geography and other parameters
- Deep learning systems trained on large numbers of human facial images could help doctors in predicting various age-related diseases
Study Limitations
The major limitations of the study were the following:- PhotoAgeClock is not an ideal biomarker of aging since it is not able to show the association with morbidity or mortality
- PhotoAgeClock works best on standardized high-resolution images of eye corners. Its potential for analysis of high-quality images generated by professional cameras and smartphones remains to be evaluated
Conclusion
With the current advances in deep learning and computer vision, it is quite evident that the AI-revolution with reference to the development of aging clocks is definitely underway. Importantly, the present research is likely to impact imaging data analysis in diverse areas like transcriptomics (the study of RNA molecules involved in transcription), proteomics (the large-scale study of proteins), data generated by sensors, as well as others.Future Plans
Since the study found that the images of the skin around the eyes were the most relevant for prediction of age, the researchers plan to carry out future studies to explore the information pattern generated, based on the condition of the human skin.Reference:
- PhotoAgeClock: deep learning algorithms for development of non-invasive visual biomarkers of aging - (https://doi.org/10.18632/aging.101629)
Source-Medindia