Artificial intelligence-driven data analytics skills are underutilized. This slows the creation of advanced predictive systems that enable early risk identification for COVID-19.
- The use of artificial intelligence in healthcare could provide individualized and cost-effective holistic care to patients
- Although ground-breaking, artificial intelligence cannot provide a rapid answer for the systematized and deeply ingrained inequities in our healthcare system
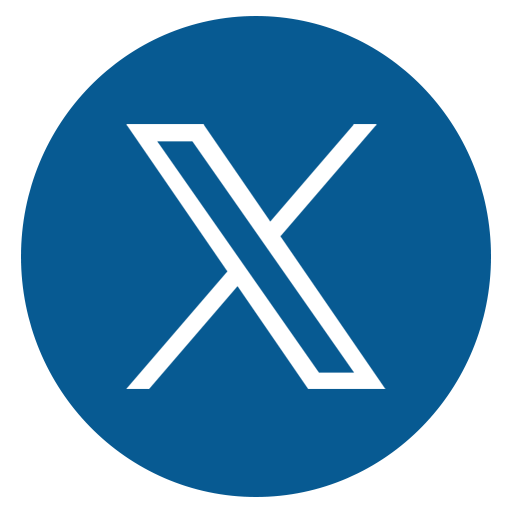
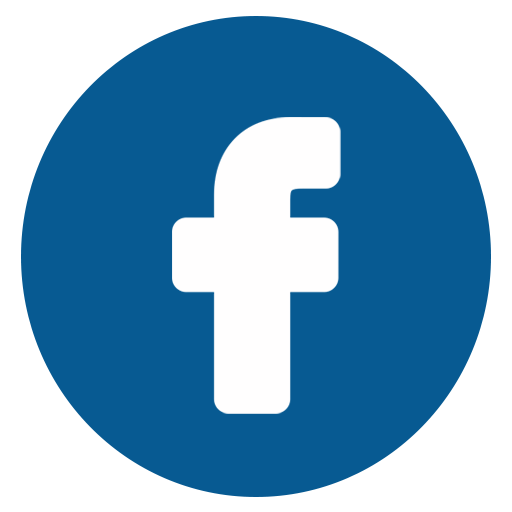
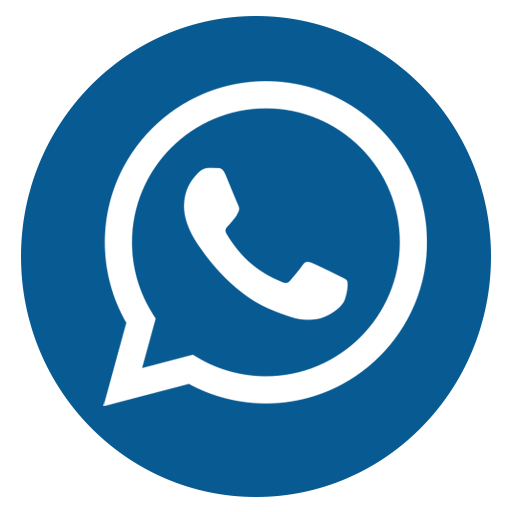
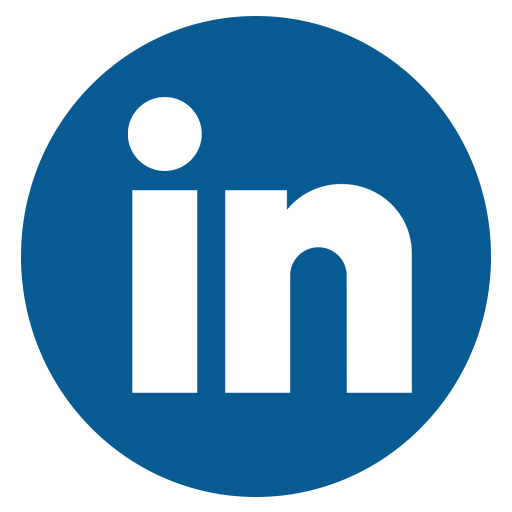
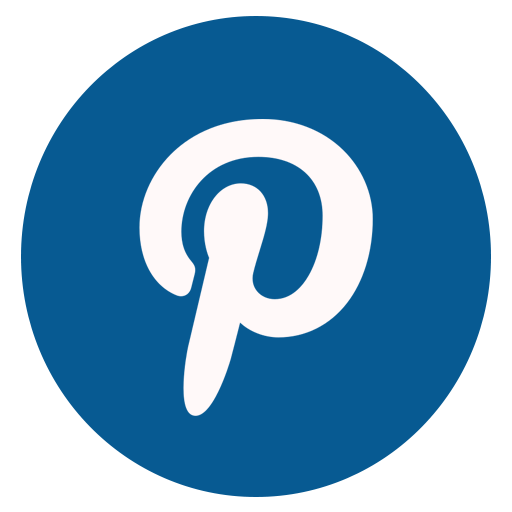
Significance of Avoiding Unnecessary Intubation
If intubation is avoided wherever possible, the danger of intubation and the side effects of mechanical ventilation, such as ventilator-induced lung injury, nosocomial infection, pressure injuries, and thrombosis, are reduced. Therefore, it is crucial to create technologies that can properly identify patients who are in danger of deterioration.From February 2020, complete data on critically ill patients with COVID-19 admitted to Australian intensive care units (ICUs) have been prospectively collected by the Short Period Incidence Study of Severe Acute Respiratory Infections (SPRINT-SARI) registry. The database’s strength comes from its consistency and dependability, which are used across more than 50 hospitals nationally thanks to thorough management and standardized collection processes. More than 100 demographic, clinical, and laboratory findings are included in the highly detailed data collected for each patient stay.
Artificial Intelligence Predicts Which Patients Requires Ventilation
The first study using artificial intelligence/machine learning to detect publicly available clinical risk factors for mechanical ventilation in patients with COVID-19 admitted to the ICU was published in October 2022 by the SPRINT-SARI collaboration of investigators. The study used Australian data. The ‘grey area’ cohort of patients, who are ill enough to warrant admission to the ICU but not yet in need of mechanical ventilatory assistance, was the study’s main emphasis.The early detection of patients at risk of invasive ventilation within three days of ICU admission was made possible by the high sensitivity (81%) of machine learning algorithms developed in the investigation, providing a potent tool to support clinical decision-making and more efficient resource allocation at the organizational level.
What is Machine Learning
A growing body of research shows that machine learning may effectively characterize and predict complicated biological processes for which there are no prior theories. It’s capacity to negotiate non-linear interactions within high dimensional data, producing a mechanism via which more precise predictions can be formed, gives it an edge over conventional (typically linear) statistical methods.Machine learning has proven to be very successful in ‘personalised prediction’, as demonstrated by the algorithm used by Netflix to tailor television show and movie suggestions or by Facebook (now Meta) to deliver tailored advertisements.
FDA-approved Algorithms in Ophthalmology and Radiology
With notable accomplishments like a detection system for diabetic retinopathy in the field of ophthalmology that was approved by the US Food and Drug Administration and automated image analysis for hospital workflow management in radiology, these potent algorithms have been implemented gradually but steadily in patient care.Machine learning technologies offer two major benefits in a health system under constant demand from staff shortages, growing prices, and protracted wait times: scalability and efficient resource allocation. Machine learning algorithms can now be installed on cloud servers, making them accessible from any location with an internet connection.
This means that by expanding internet connection in Australia, we may work toward achieving fair access to artificial intelligence-based medical technology. Additionally, the idea of integrating telehealth services and machine learning-driven decision assistance is promising. Over 95 000 practitioners countrywide provided 118.2 million telehealth services to 18 million patients between 13 March 2020 and 31 July 2022.
In the future, seamless artificial intelligence integration into the backend of telehealth services may complement prompt diagnosis and early action. Artificial intelligence integrated into the electronic medical record may help with resource allocation by speeding up the triaging of patients for primary and tertiary care. To predict the progression of conditions like diabetes to chronic kidney disease, for instance, established, precise models based on huge datasets (>50 000 individuals) already exist.
These are useful in making sure high-risk patients are given priority and aren’t ‘lost’ in the long waiting lists for specialized care, especially in rural areas.
Resistance to Adopting Artificial Intelligence in Healthcare
Despite the obvious benefits of artificial intelligence, resistance to its use in clinical settings still exists, most notably because of the complexity of machine learning algorithms. The term ‘black box’ still permeates the discourse surrounding the use of artificial intelligence in healthcare, encapsulating the widespread skepticism against a system whose inner workings are hidden from the clinicians who use it. There is concern that handing up decision-making authority to an unknowable ally may breach the clinician’s basic duty to safeguard the welfare of patients and expose them to medico-legal risk.Patients are also wary because the ability of ‘non-human’ computers to decide how to manage them may make them uncomfortable. The trade-off between model complexity and explicability further complicates matters. The most challenging algorithms to comprehend are frequently those that are complicated and best suited to extract and exploit data trends.
In addition, third-party explanation systems that are as inventive and sophisticated as the algorithms are being developed, which is an important step in closing this trust gap. A unique explanatory modelling framework for enhanced non-linear machine learning model interpretability was developed in 2020, and it utilized a game theory-based methodology to rationalize the contributions made by various input features to the predictions being made.
The SPRINT-SARI group used this technique to establish which characteristics were most helpful in their artificial intelligence system for foretelling invasive ventilation in critically ill COVID-19 patients. It was intended to give doctors a better understanding of the reasoning behind the algorithm to increase clinician trust and adoption. In the future, there is hope for a better working connection between medical experts and artificial intelligence-based solutions used in hospitals thanks to the algorithm transparency attained by this unique method.
Predictive algorithms’ utility is severely constrained by the demographic and disease-related characteristics of the cohort in question because, in the end, they are only as good as the data used to train them.
Source-Medindia