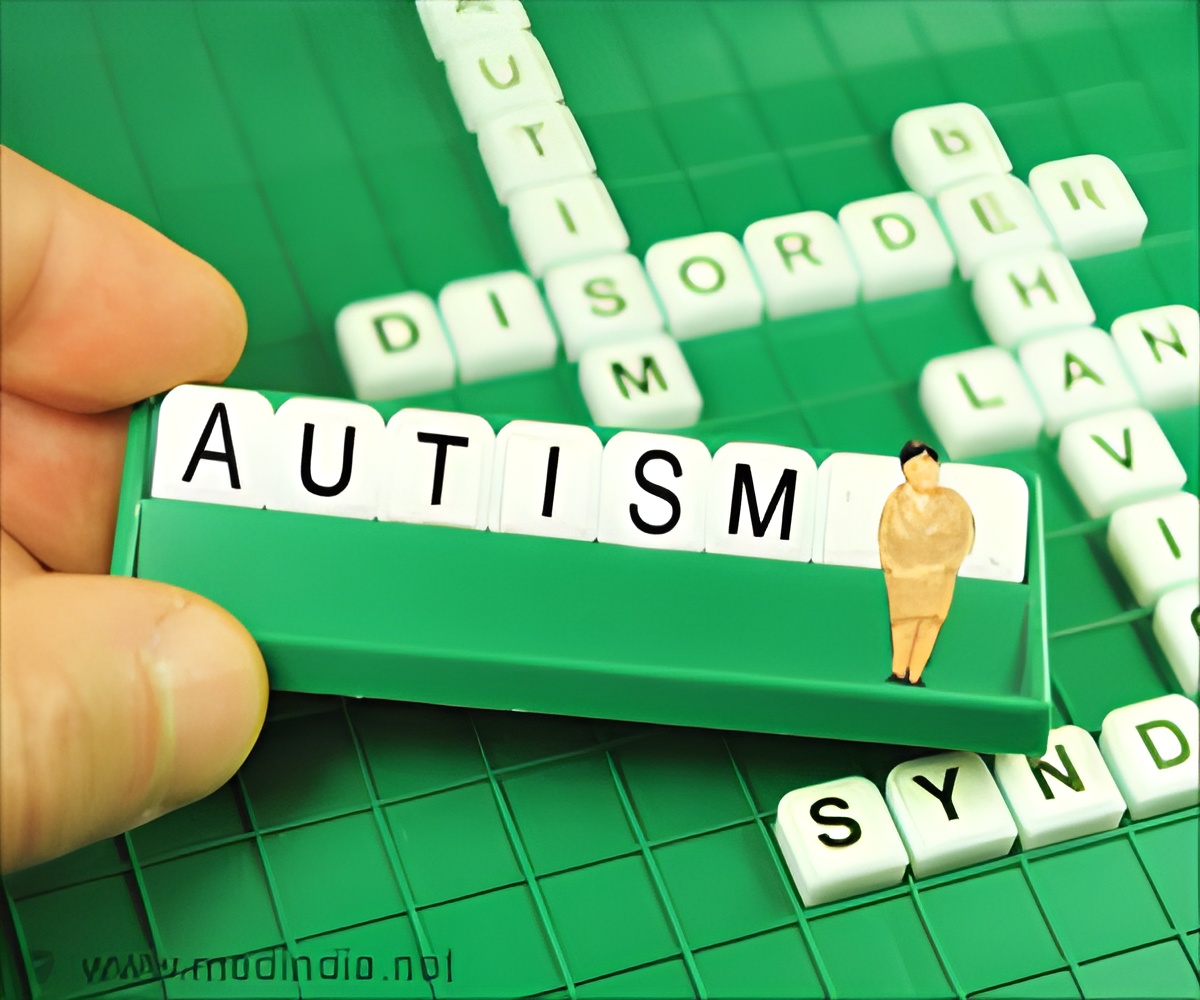
Machine Learning Prediction of Autism Spectrum Disorder From a Minimal Set of Medical and Background Information
Go to source).
‘AutMedAI beats traditional methods, achieving 89.5% accuracy in early autism diagnosis #Autism #AI #ASD #medindia
’
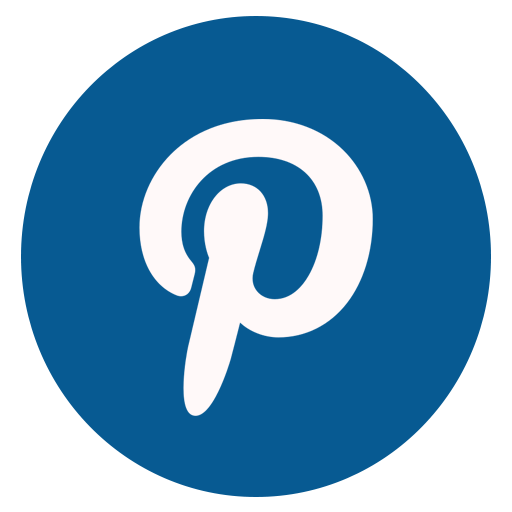
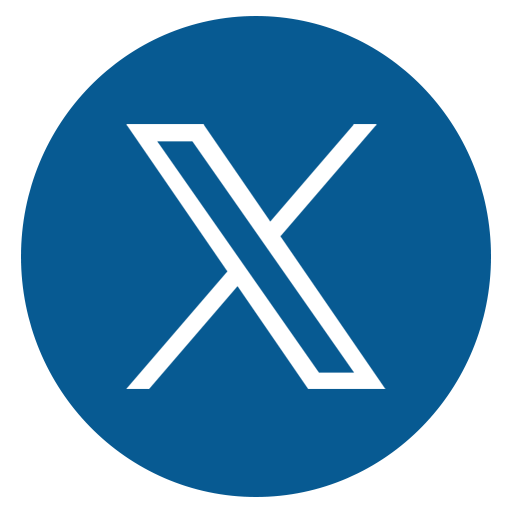
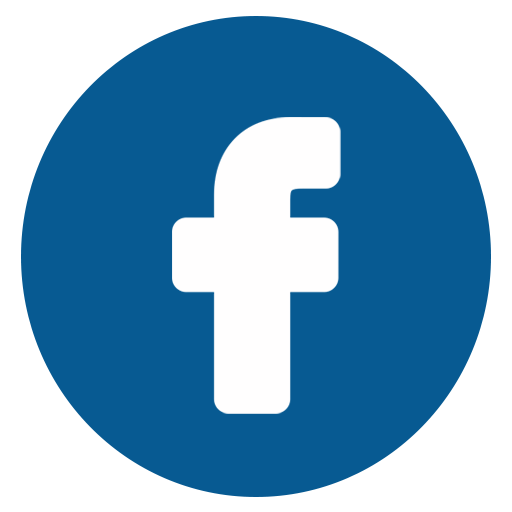
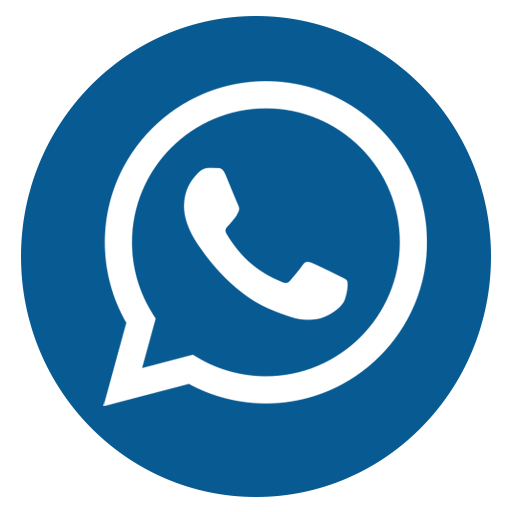
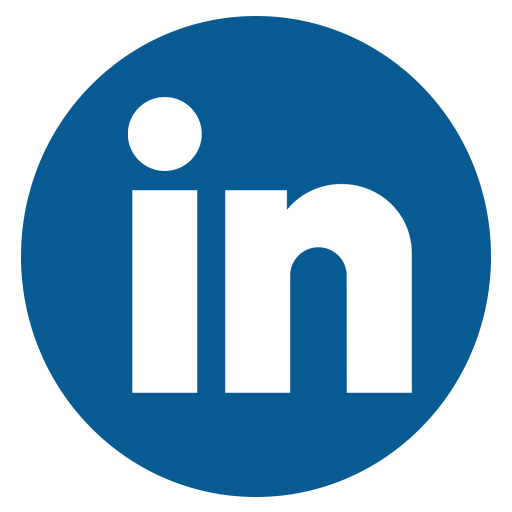
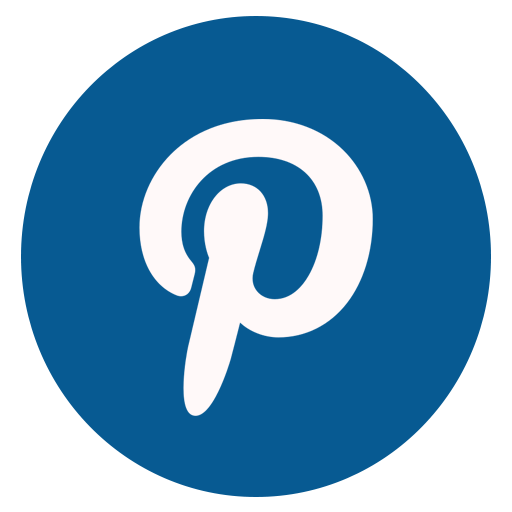
How AutMedAI Was Developed
The study was conducted in Sweden with the approval from Swedish Ethical Committee by following the Transparent Reporting of a Multivariable Prediction Model for Individual Prognosis or Diagnosis (TRIPOD) reporting guidelines.All patients were consented and the data collection was approved by the ethical committee for the SPARK and SSC projects involving 30,660 individuals (15,330 with ASD and 15,330 without ASD) for analysis from 31 university-affiliated research clinics and online in 26 US states. 28 basic medical screening and background information before 24 months of age was collected and utilized for the development of the model.
The model was developed using four different ML algorithms—logistic regression, decision tree, random forest, and eXtreme Gradient Boosting (XGBoost). Performance Metrics- Accuracy, AUROC, sensitivity, specificity, positive predictive value (PPV), and F1 score were used.
AutMedAI’s Fabulous Results
Among the four algorithms, XGBoost model, later named as AutMedAI, showed high accuracy in diagnosing ASD and the model was tested on new datasets, giving good results across different age groups and both sexes.High performance was observed for AutMedAI model with an average area under the receiver operating characteristics curve (AUROC) of 0.895 indicating high accuracy in differentiating children with ASD from the non-ASD children.
Advertisement
The positive outcomes of AutMedAI prove the possibility to use its algorithm for the early diagnosis of ASD in children as a non-invasive technique. It signifies that ASD can be predicted with a small amount of information, allowing for earlier diagnosis, and early interventions which are so vital for the developmental outcomes.
Advertisement
Reference:
- Machine Learning Prediction of Autism Spectrum Disorder From a Minimal Set of Medical and Background Information - (https://jamanetwork.com/journals/jamanetworkopen/fullarticle/2822394?)
Source-Medindia