Discover how machine learning models are revolutionizing the diagnosis of occlusion myocardial infarction (OMI) in patients with acute chest pain.
- Machine learning models surpass clinicians and commercial systems, accurately diagnosing OMI through subtle ECG patterns
- Improved diagnostic accuracy leads to timely reperfusion therapy, reducing morbidity and mortality rates
- Implementation of machine learning models optimizes decision-making, streamlining patient care and resource allocation
Machine learning for ECG diagnosis and risk stratification of occlusion myocardial infarction
Go to source). The study utilized data from 7,313 consecutive patients with chest pain across multiple clinical sites. The intelligent model derived from this study outperformed practicing clinicians and other widely used commercial interpretation systems, significantly improving both precision and sensitivity. The study also introduced an OMI risk score that demonstrated enhanced rule-in and rule-out accuracy, enabling correct reclassification of one in three patients with chest pain. Additionally, the ECG features identified by the models were validated by clinical experts, establishing plausible mechanistic links to myocardial injury.
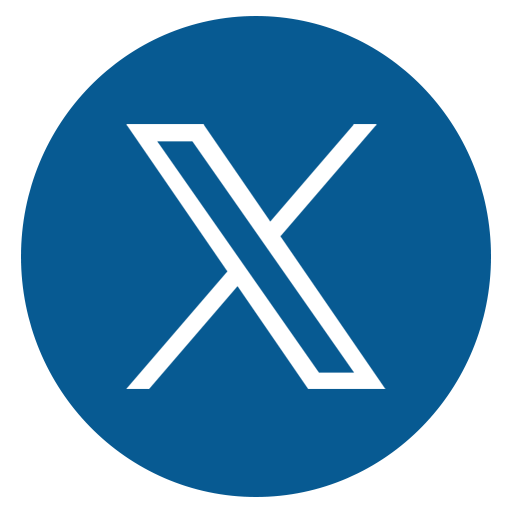
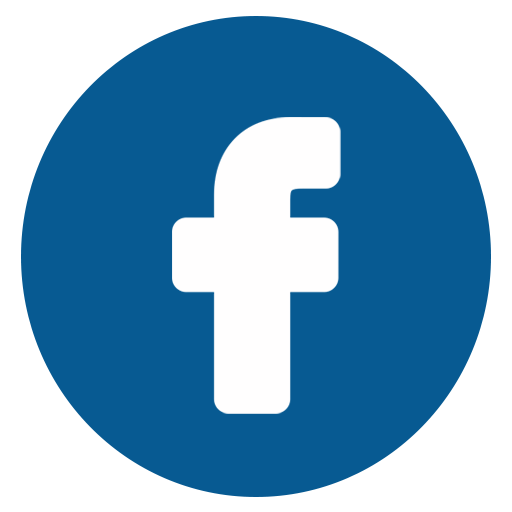
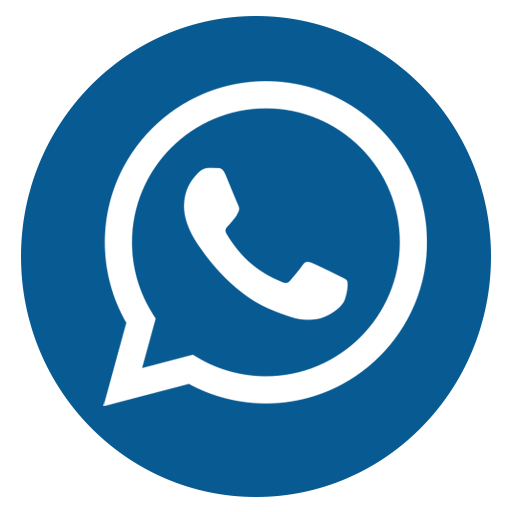
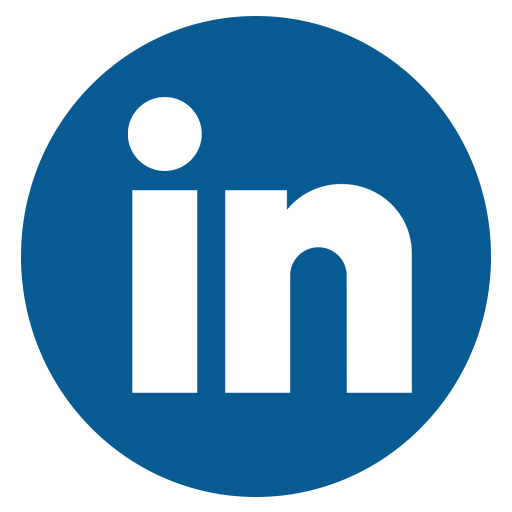
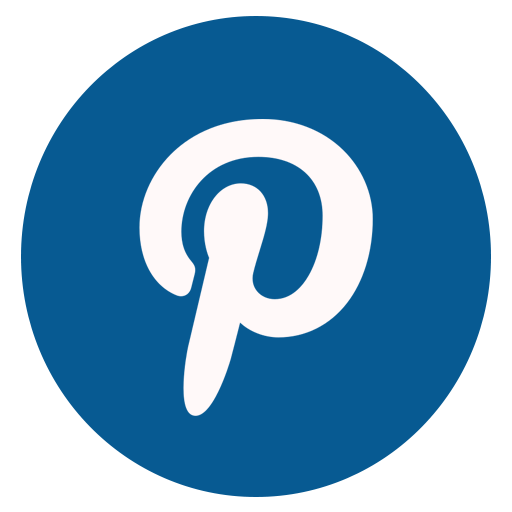
Challenges in Occlusion Myocardial Infarction (OMI) Diagnosis
Diagnosing OMI in patients without ST-elevation presents two significant limitations within the current diagnostic paradigm. Firstly, a considerable number of patients with non-STEMI have total coronary occlusion, and prompt identification of this vulnerable group is crucial for timely reperfusion therapy. However, the existing ECG signatures of OMI described in the literature are subtle and involve the entire QRST complex, making visual inspection by clinical experts suboptimal and leading to a high degree of variability in interpretation.The second limitation lies in the use of cardiac biomarkers, such as troponin, which cannot differentiate OMI until peak levels are reached. Consequently, many patients with OMI go undiagnosed or experience delays in treatment. Moreover, a significant proportion of acute myocardial infarction cases present with negative initial troponin results, further complicating the diagnosis. These diagnostic limitations contribute to inefficient clinical practice, where patients with chest pain are often over-monitored, while those with OMI face delayed diagnosis and treatment, leading to increased mortality rates.
Developing Machine Learning Models for ECG Diagnosis
Building upon previous work on AI-enabled ECG analysis, the present study utilized machine learning algorithms to develop an intelligent model for the ECG diagnosis and risk stratification of OMI in patients with acute chest pain but no ST-elevation. The study employed a multi-site, prospective, observational cohort design, enrolling 7,313 patients with chest pain from various clinical sites in the United States.The results of the study demonstrated the superiority of machine learning in detecting subtle ischemic ECG changes indicative of OMI, surpassing the performance of practicing clinicians and widely used commercial ECG interpretation software. The machine learning models identified important ECG features associated with OMI and provided an objective and quantifiable approach to evaluate these subtle patterns. These features included T wave characteristics, such as TpeakāTend interval prolongation, T wave flattening, and ventricular repolarization dispersion.
The derived OMI risk score proved to be an accurate tool for rule-in and rule-out of OMI, outperforming the traditional HEART score. Combining the OMI risk score with the clinical judgment of trained emergency personnel, one in three patients with chest pain could be correctly reclassified. This improved diagnostic accuracy could have significant implications for patient outcomes, resource allocation, and decision-making in emergency departments.
Benefits and Implications of Machine Learning Models for ECG Interpretation
The implementation of machine learning models for OMI diagnosis has several potential benefits and implications for clinical practice. By providing more accurate and timely identification of patients with OMI, these models can improve triage decisions, enabling appropriate and timely reperfusion therapy. This, in turn, may lead to reduced morbidity and mortality rates in this vulnerable patient population.Moreover, the enhanced diagnostic capabilities offered by machine learning models can inform various aspects of clinical decision-making, including pre-hospital interventions, activation of catheterization laboratories, administration of anti-ischemic therapies, hospital destination choices, the need for medical consults, referrals for diagnostic testing, and early discharge decisions. Implementing these models in routine clinical practice could streamline and optimize the care pathway for patients with acute chest pain, reducing unnecessary investigations and hospitalizations while ensuring appropriate treatment for those at high risk.
Furthermore, identifying specific ECG features associated with OMI through machine learning models can provide valuable mechanistic insights into myocardial injury. These findings can contribute to our understanding of the pathophysiological mechanisms underlying OMI and potentially inform the development of targeted interventions.
Machine learning models offer a promising solution for enhancing the ECG diagnosis of OMI in patients with acute chest pain but no ST-elevation. These models surpass the accuracy of practicing clinicians and existing commercial interpretation systems, providing an objective and quantifiable approach to identifying subtle ECG patterns associated with OMI. The introduction of an OMI risk score further enhances diagnostic accuracy, aiding in the correct reclassification of patients and improving clinical decision-making. By implementing these models in clinical practice, clinicians can make more informed decisions regarding patient management, leading to improved outcomes and resource allocation. Prospective trials are warranted to evaluate the clinical effectiveness of this diagnostic approach.
Reference:
- Machine learning for ECG diagnosis and risk stratification of occlusion myocardial infarction - (https://www.nature.com/articles/s41591-023-02396-3)
Source-Medindia