Algorithms that were found to identify patients who are at risk of acquiring HIV and may benefit from preventive care developed.
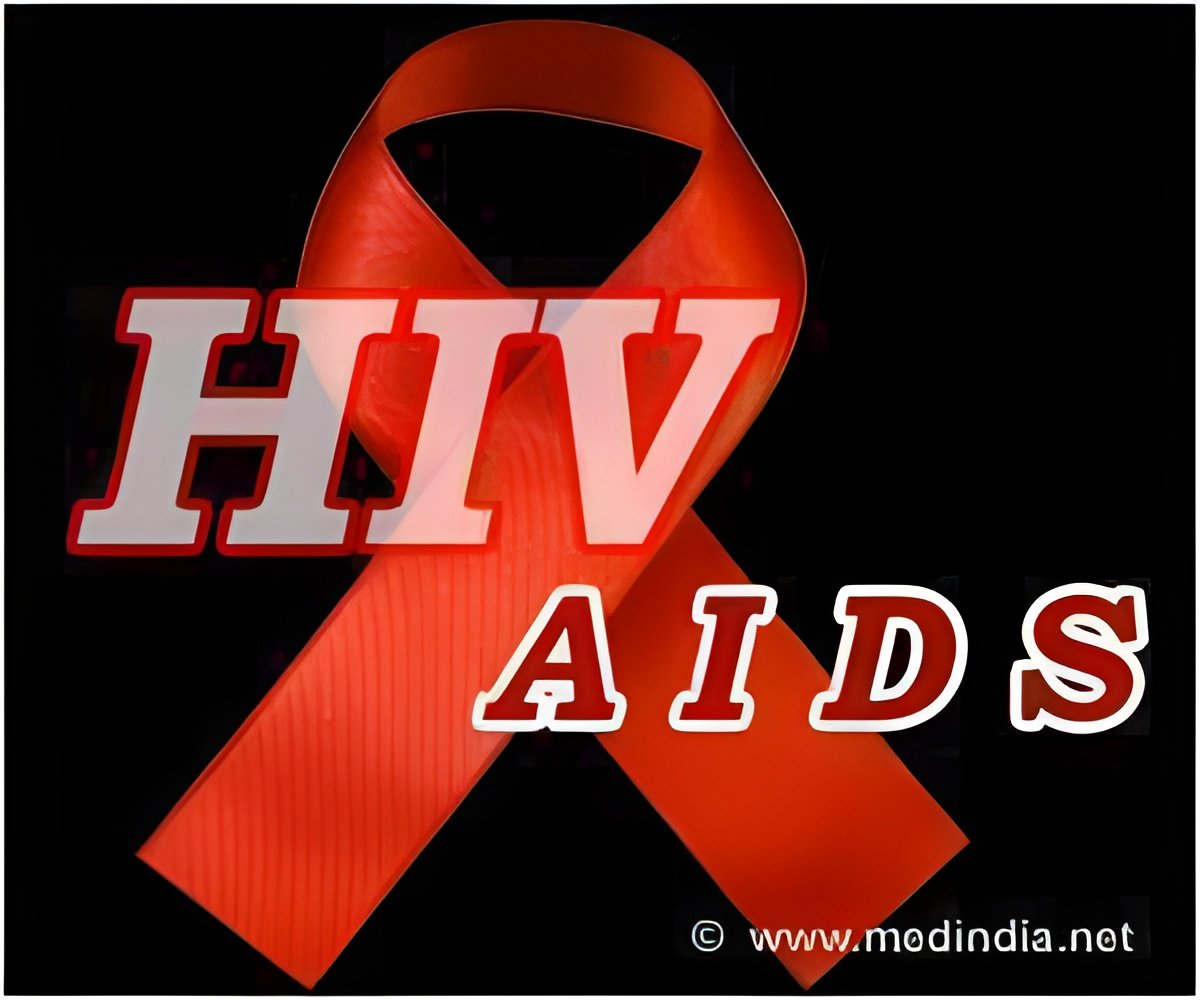
One barrier to use is the difficulty for providers in identifying patients who are at high risk of HIV acquisition. Providers often have limited time, may have limited knowledge about PrEP, and may lack training in how to talk to patients about sex or substance use. Risk prediction tools, a form of electronic clinical decision support using the data in patients' electronic health records (EHRs), are often used in other areas of medicine.
Researchers from both studies, one using a patient population in California and the other in Massachusetts, built HIV risk prediction models that could be used in EHRs as automated screening tools for PrEP.
The two studies looked back at the medical records of millions of patients who were HIV-uninfected and had not yet used PrEP. Researchers extracted demographic and clinical data from these patients' EHRs on numerous potential predictors of HIV risk. A machine-learning algorithm automatically selected important HIV risk-related variables for the final models.
In the California-based study, which used medical record data of 3.7 million patients at Kaiser Permanente Northern California, the final risk prediction model included such variables as sex, race, living in a neighborhood with high HIV incidence, use of medications for erectile dysfunction, and sexually-transmitted infection (STI) testing and positivity. The model flagged 2% of the general patient population as potential PrEP candidates and identified 46% of male HIV cases, but none among females.
"Although risk prediction tools are imperfect and cannot replace the clinical judgement of skilled providers, our algorithms can help prompt discussions about PrEP with the patients who are most likely to benefit from it," said Julia Marcus, PhD, MPH, lead author of the California-based study and Assistant Professor of Population Medicine at the Harvard Pilgrim Health Care Institute and Harvard Medical School.
Advertisement
Advertisement
Jonathan Volk, MD, senior author of the California-based study and an infectious disease physician at Kaiser Permanente San Francisco Medical Center added that "a recent publication by the U.S. Preventive Services Task Force in JAMA cites the lack of effective prediction models as a major gap in research that is critical to improving PrEP delivery. Our model helps fill that gap."
Source-Eurekalert