New computational tool combines multiple types of genomic information to make stronger predictions about what genomic features are associated with specific drug responses; the tool is designed by University of Illinois and the Mayo Clinic.
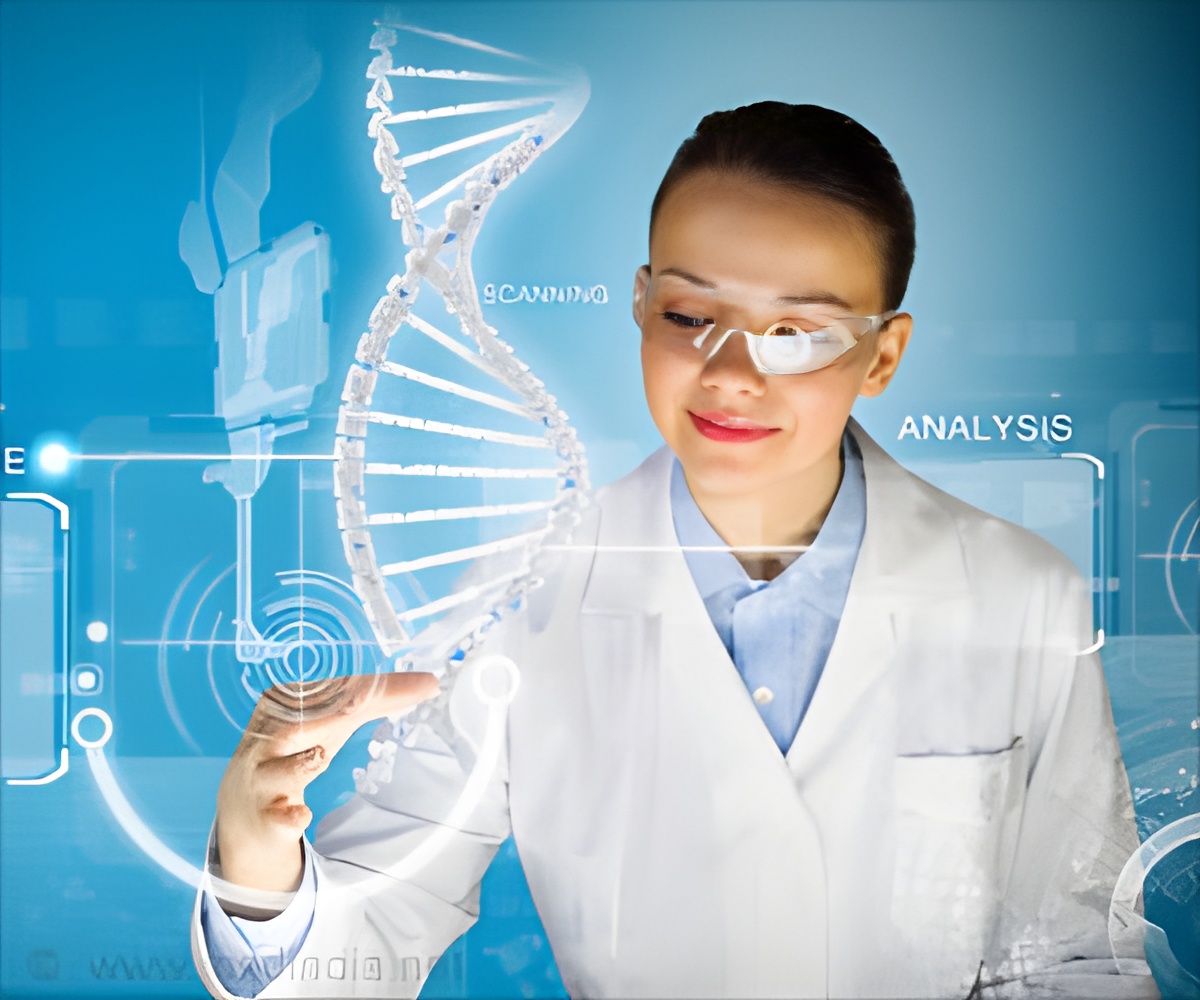
‘A new computational tool developed by the University of Illinois and the Mayo Clinic uses multiple types of genomic information to provide individualized responses to anticancer drugs.’
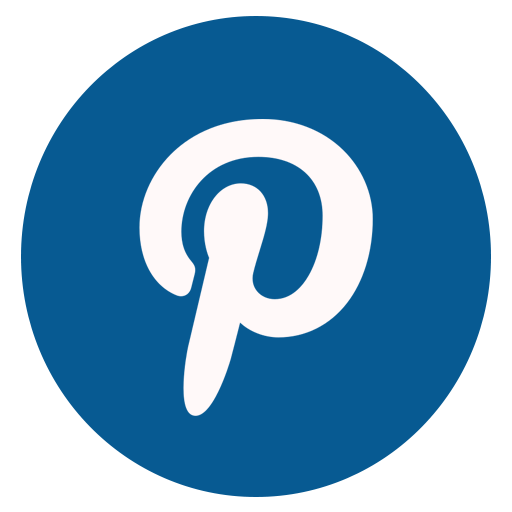
"We all know treatment outcomes for complex diseases like cancers vary dramatically among individuals, from lacking of efficacy resulting in disease recurring to severe toxicity resulting in noncompliance in patients who cannot tolerate these life-saving drugs," said Leiwei Wang, a professor of pharmacology at the Mayo Clinic. "Therefore, it is extremely important for us to understand better of how and why patients respond differently, so that we can truly individualize their therapies by choosing the right drug at the right dose." 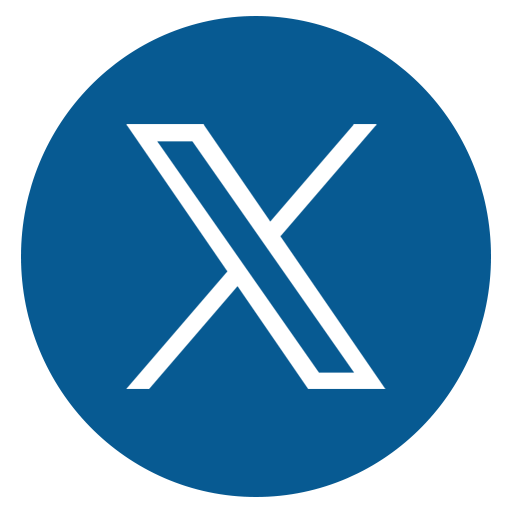
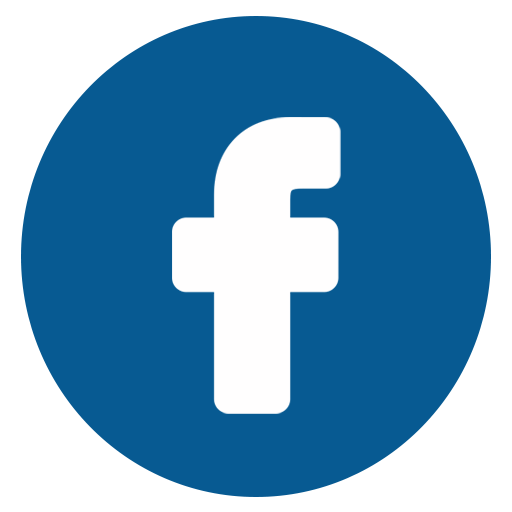
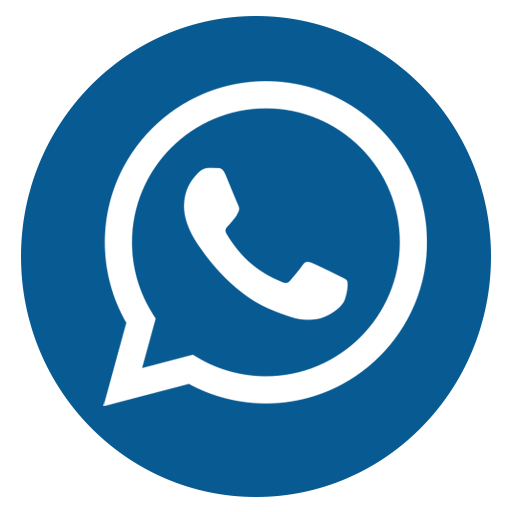
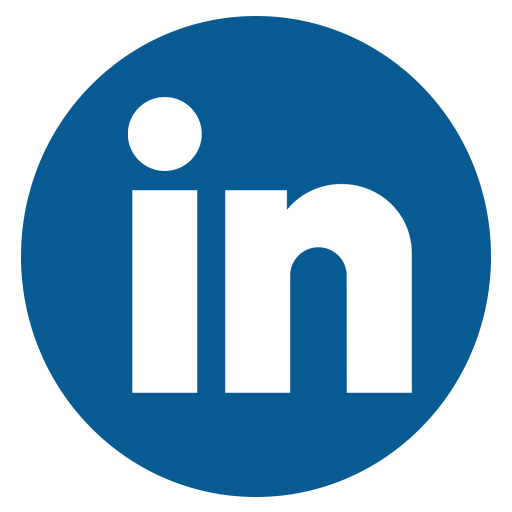
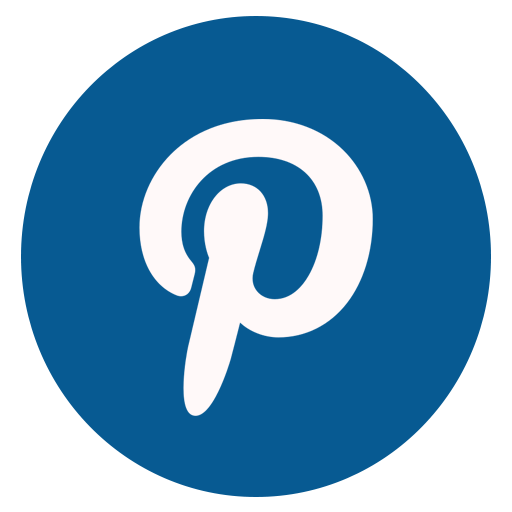
The researchers' first step toward this goal was a large-scale data collection effort. They assembled a panel of lab-reared tumor cells derived from a diverse set of individuals, and exposed samples of those cells to one of a set of common anticancer drugs. This allowed them to quantify the drug responses of different genetic backgrounds in a directly comparable way.
Using these data, Mayo Clinic researchers wanted to ask what characteristics of cells from each individual helped determine its unique set of responses to the drugs tested. They collected data on the "expression" of every gene in the genome, how often each gene was being read by the cell and used to create the corresponding protein that gene carries the instructions for.
The team also wanted to look at where those differences in gene expression might come from. DNA sequence surrounding genes in the genome influence when genes are expressed. So do the actions of special proteins called transcription factors, which bind to DNA and make it easier or harder for genes to be read by cellular machinery. Finally, how different regions of the long DNA strands of the genome are coiled up, the "epigenetic state" of genomic DNA also helps determine how likely a gene is to be expressed.
The team decided to collect data on all of these characteristics of their lines of cells. They had built a comprehensive dataset, but lacked something vital, an analytical tool that could use it to full advantage.
Advertisement
Sinha and graduate student Casey Hanson developed an algorithm that takes in data on gene expression, genomic factors that help control gene expression, and resulting traits (such as drug response) and uses these to predict which genes are most important in determining the latter. They based their work on a tool they had previously developed named "Gene Expression in the Middle," or GENMi. Their new model, because of its ability to appropriately weight and integrate multiple sources of data, is named "probabilistic GENMi" or pGENMi.
Advertisement
Because this tool is the first of its kind, team had to get creative to assess how well it was working, they had no prior standard of performance for comparison, and the results generated by pGENMi are the basis for further experimental work, not an endpoint.
"Our end result was testable predictions . . . a ranking of what experiments to do and verify that this transcription factor indeed has a role in regulating the response to that drug," Sinha said.
"In a lot of computer science and bioinformatics papers, there is a gold standard database to validate predictions against - but we didn't have the luxury of that," Hanson said. "We had to search a vast literature to try to find, among the myriad ways of doing so and stating that one has done so, experiments that [could] confirm our hypothesis." The team's mix of computer science and biological knowledge was what made this task possible.
Hanson and his coauthors examined whether the predictions generated by the algorithm included associations that were already confirmed by the studies he identified. The literature searches revealed examples in which transcription factors highlighted by pGENMi had been experimentally manipulated, resulting in changes in drug responsiveness. Many of the predictions generated by pGENMi were supported by previous work, making it likely that those not supported by prior work are novel but real associations.
"For example . . . we found a paper in which rapamycin, an anticancer drug, decreased GATA1 [a transcription factor's] binding with DNA. Another paper, we found that . . . rapamycin increased expression of a gene, ERCC1," Hanson said. The same paper linked the transcription factor, GATA1, to ERCC1's expression. Hanson noted that "our own experiments showed that knocking down GATA1 changed the sensitivity of cells to rapamycin," in agreement with the previous work.
To test pGENMi's results even further, the group selected transcription factors predicted to impact drug responsiveness, as well as several predicted to have little impact, and reduced their function in lab-grown cancer cells. For the majority of the TFs examined, these experimental results were consistent with pGENMi's predictions.
Although in this initial project pGENMi was used to explore the factors that influence the response of cancer cells to therapeutic drugs, its flexibility would allow for a wide range of applications.
"We have generated tools that can be used broadly by the research community. These tools will be open to anyone who might have the right data sets to both help generate hypothesis and also to help refine the algorithms," Wang said. "This is a perfect example of how expertise in complementary research areas, in this case, computational science and pharmacoproteomics, come together to make a difference."
The complete study is published in Genome Research.
Source-Eurekalert