A new machine learning model developed by researchers helps predict the risk of mortality of intensive care unit patients according to their characteristics.
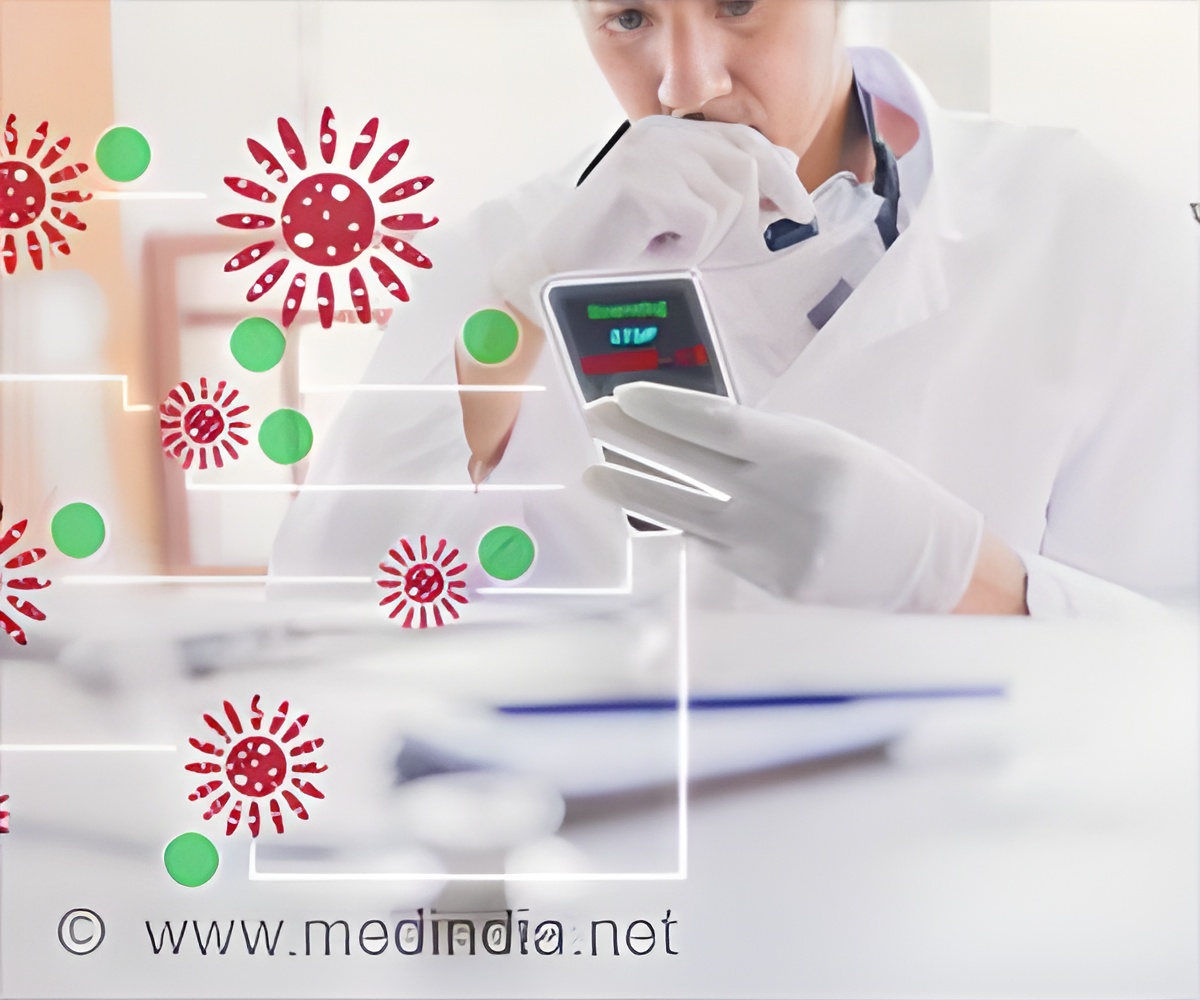
‘The hierarchical machine learning predictive prognosis model has a good predictive behavior, and it also allows studying which of the patient's traits are the most decisive, which can become risk factors, in assessing their risk of death.’
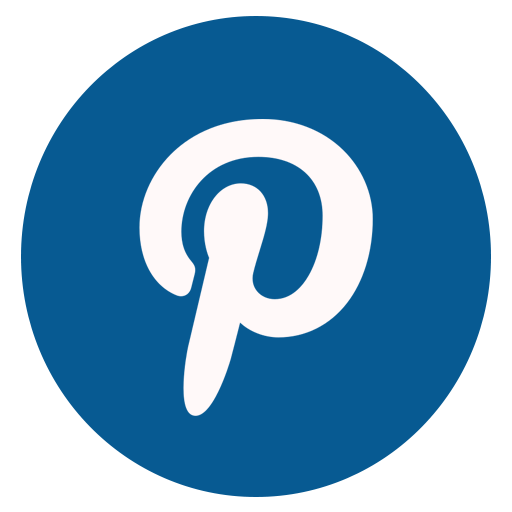
This process is complex and comes at a high cost, and depends on the inherent variability of the opinion of specialists, based on their experience and instinct. Therefore, to improve the quality of care in the ICUs, it is important to set down protocols based on objective data and on an accurate prediction of a patient's risk of mortality according to their characteristics. In this sense, machine learning tools may be of great help to medical experts. 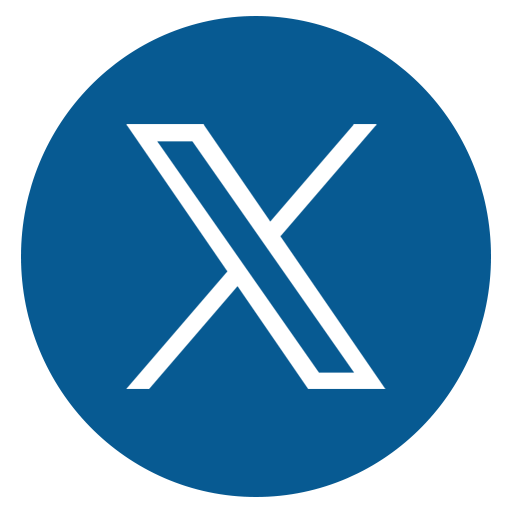
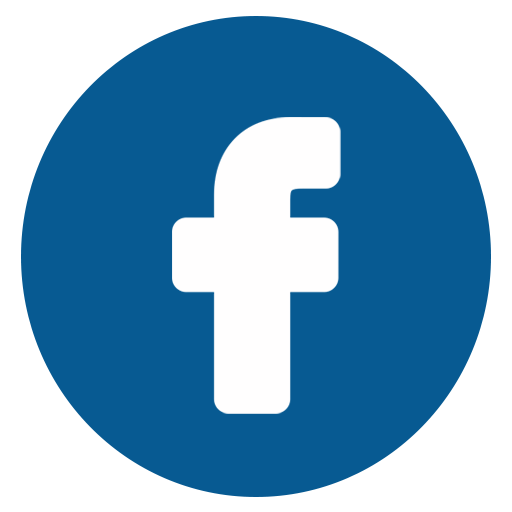
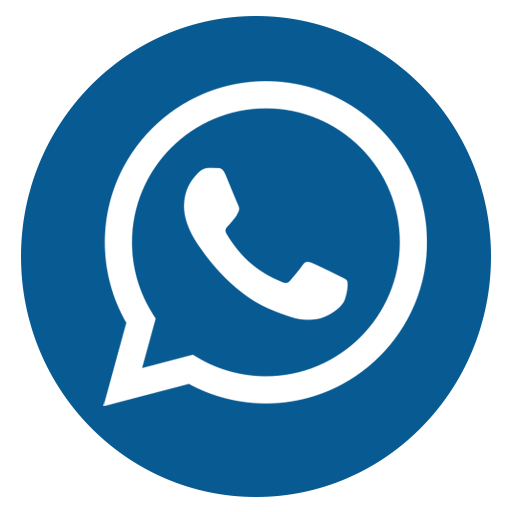
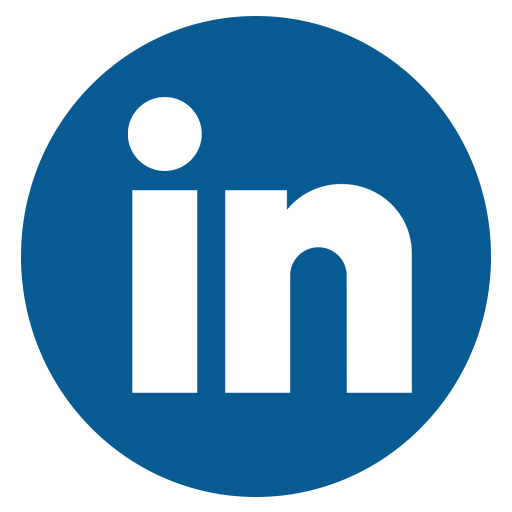
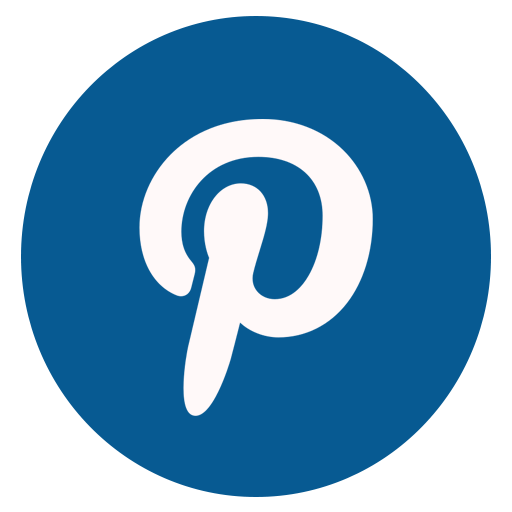
A group of researchers led by Dr Rosario Delgado from the Department of Mathematics of the UAB, in collaboration with Head of the ICU at Hospital de Mataró Dr Juan Carlos Yébenes, UAB associate lecturer Àngel Lavado from the Information Management Unit of the Maresme Health Consortium, and José David Núñez-González, PhD student of the UAB Department of Mathematics, used machine learning tools to create a model capable of predicting the risk of mortality of ICU patients, based on a real database which also served to validate the model. The model will aid in the decision-making process of healthcare workers by improving the prediction of premature deaths, making medical decisions about high-risk patients more efficient, evaluating the effectiveness of new treatments and detecting changes in clinical practices.
The use of this model represents a clear improvement in traditional approaches, consistent with predicting the risk of mortality based on the Acute Physiology And Chronic Health Evaluation (APACHE) score - a questionnaire widely used to assess a person's state of health with the help of different indicators. The new model makes use of an estimated logistical regression that was validated in previous groups of patients. Researchers were able to demonstrate experimentally that the new model they created overcomes the weak points of traditional approaches, offering good results and presenting itself as a better alternative.
The predictive self-learning prognosis model created by researchers consists in a set of Bayesian classifiers used by assigning a life prognosis label (live or die) to each individual, according to traits such as demography, gender and age; the Charlson comorbidity index; their place of origin; the cause of admission; the presence or lack of sepsis; severity reached in the first 24 hours after aadmission; and the APACHE II score.
Researchers improved the model's prediction through a combination of individual predictions of each classifier designed in a way that the faults of some predictions could be compensated with other correct predictions, and taking into account the imbalance represented by a low proportion of patients dying in the ICUs. The model predicts the cause of death of patients at a high risk, as well as the outcome of patients at a low risk of dying. This type of model is known as a hierarchical predictive model, given that there are two stages of prediction.
Advertisement
Source-Eurekalert