New model predicts the risk of opioid overdose among Medicare beneficiaries with at least one opioid prescription.
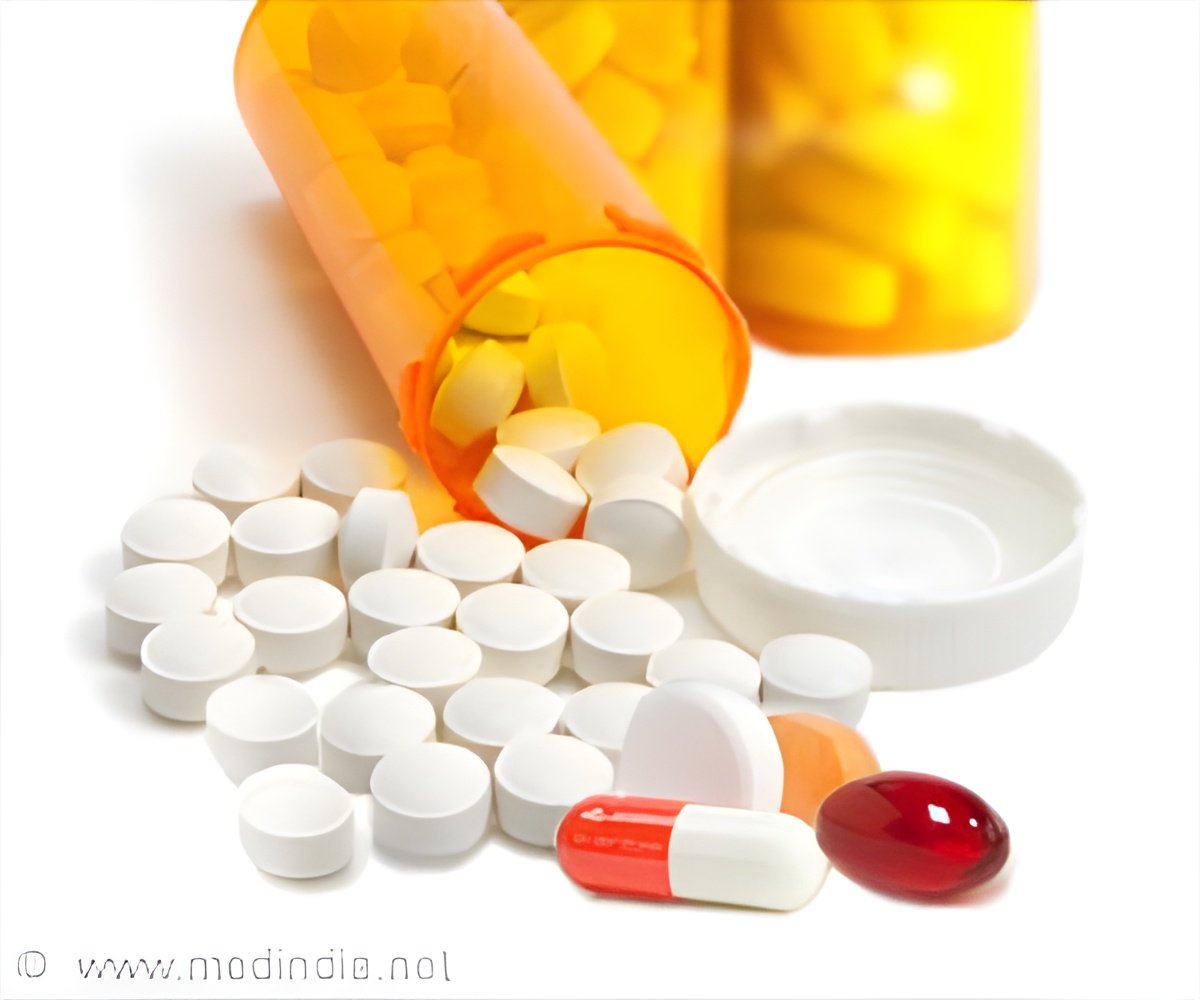
‘The machine-learning algorithms developed performed well in predicting risk of opioid overdose and identifying subgroups of patients at similar risk of overdosing. ’
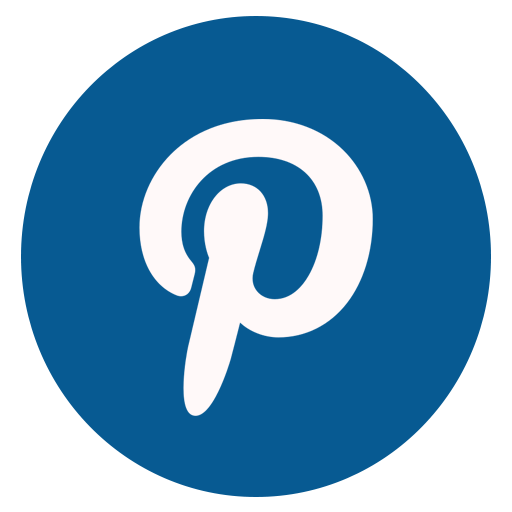
"Our model was effective in dividing the participants into three risk groups according to predicted risk score, with three-quarters in a low-risk group with a negligible rate of overdose, and more than 90 percent of individuals with overdose captured in the high- and medium-risk groups," explains Jeremy C. Weiss, assistant professor of health informatics at Carnegie Mellon University's Heinz College, who participated in the study. 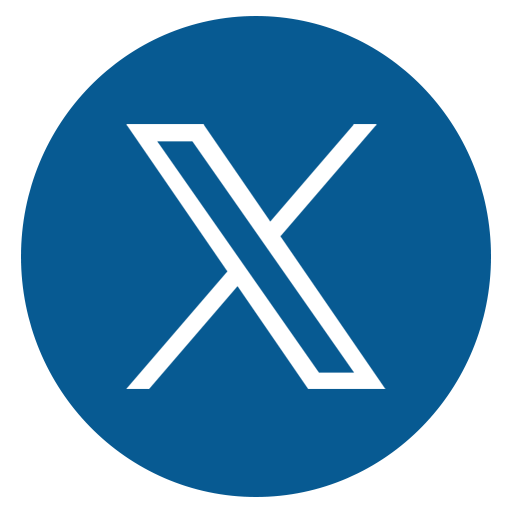
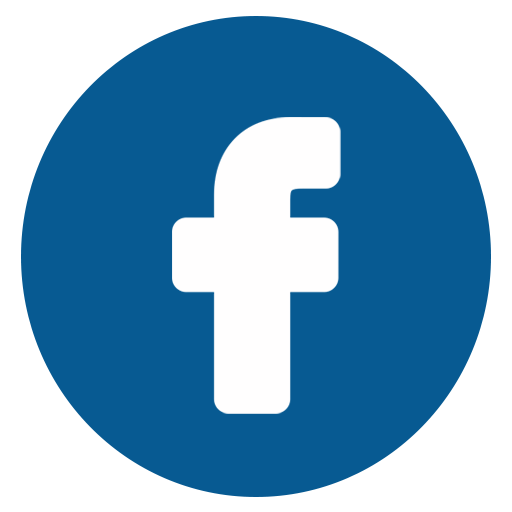
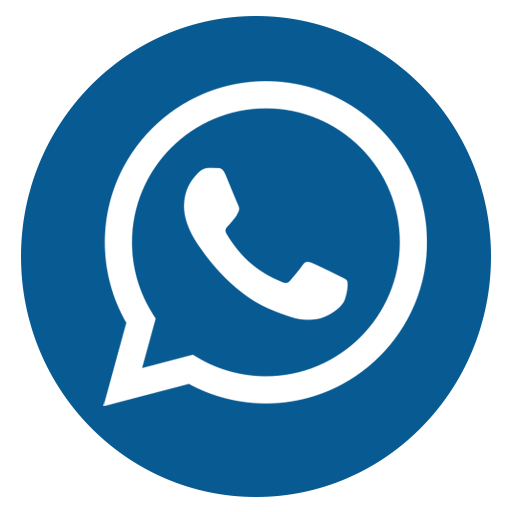
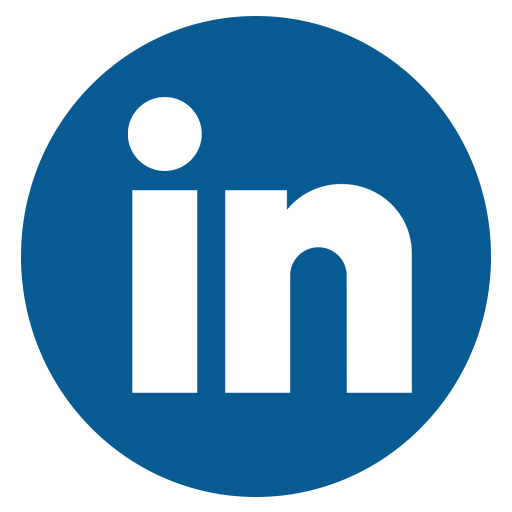
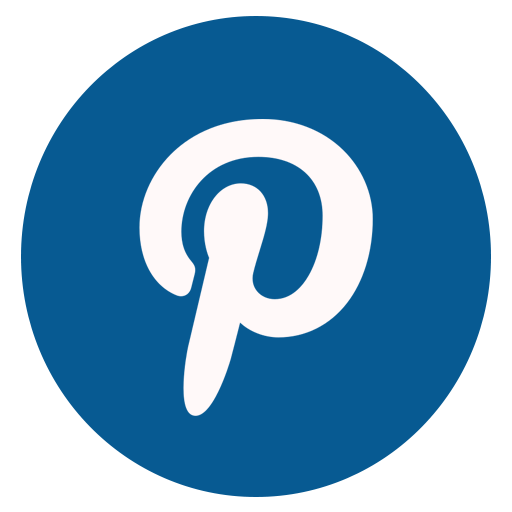
"The ability to identify such risk groups has important implications for policymakers and insurers who currently target interventions based on less accurate measures to identify patients at high risk," according to Wei-Hsuan "Jenny" Lo-Ciganic, assistant professor of pharmaceutical outcomes and policy at the University of Florida, who was lead author on the study.
In 2017-2018, researchers studied 560,057 fee-for-service Medicare beneficiaries without cancer who filled one or more prescriptions for opioids between 2011 and 2015. Those individuals were randomly assigned and equally divided into subgroups. Every three months, the researchers measured potential predictors of opioid overdose, including participants' socio-demographic characteristics (e.g., age, sex, disability status), health status, patterns of opioid use, and factors related to the participants' practitioners and the regions where they lived.
The researchers then identified opioid overdoses from inpatient and emergency room claims, and predicted risk of overdose in the three months after participants began treatment with the drugs. Machine learning is an alternative analytic approach to handling complex interactions in large data, discovering hidden patterns, and generating predictions in clinical settings. Based on their findings, the researchers concluded that their approach outperformed other methods for identifying risk, such as traditional statistical models.
The authors caution that their study looked only at patients who obtained opioids from medical settings, not those who got them from nonmedical settings, which are not captured in claims data. In addition, the study looked at overdoses in medical settings, not overdoses outside those settings.
Advertisement
Source-Eurekalert