Scientists have revealed the use of smartwatch sensor and developing predictive models using machine learning to help prevent opioid relapse, overdose and dependency.
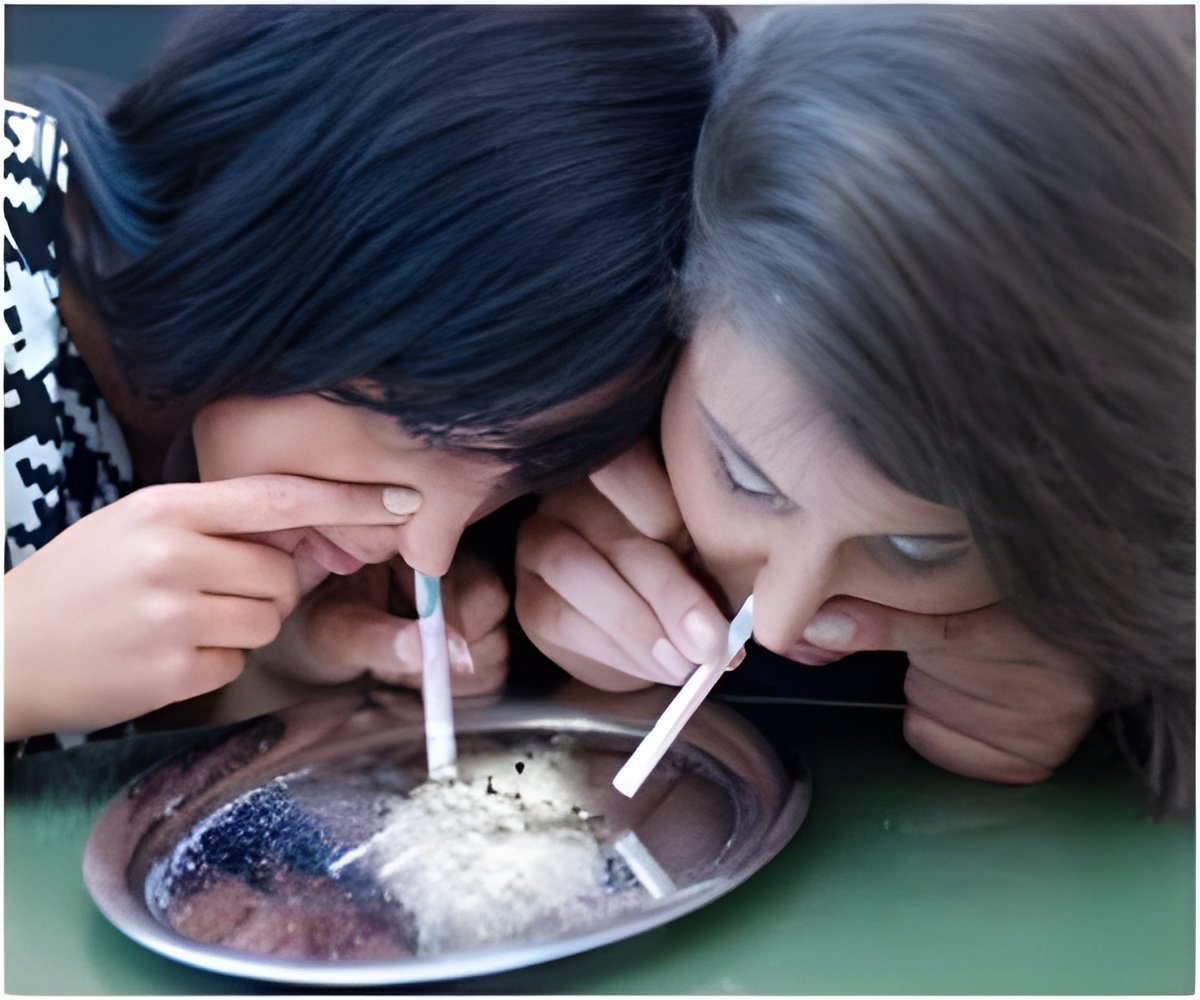
‘Successful execution of the research will begin to test the effectiveness of integrating passive sensing, adaptive artificial intelligence (AI) and mindfulness interventions on regulating drug craving.’
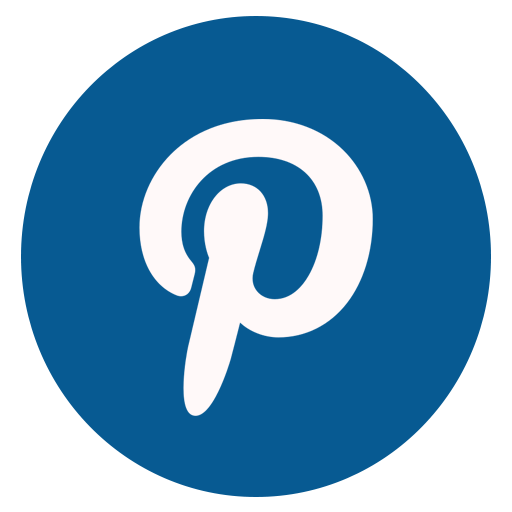
“We have the technology to detect these craving moments and incorporate an intervention to avoid scenarios of drug use,” says Rahman, who focuses on creating mobile, health-related sensors in the MOSAIC Lab he co-directs. 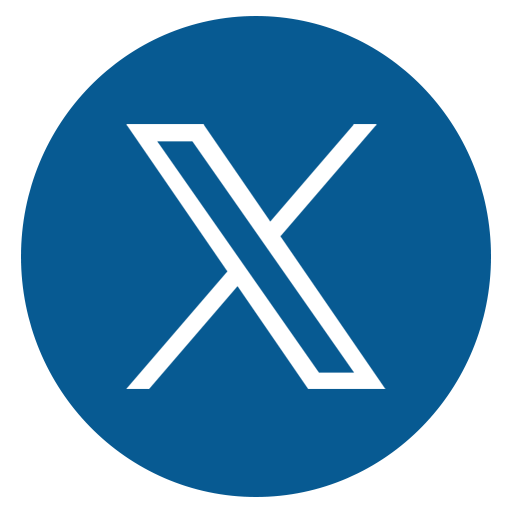
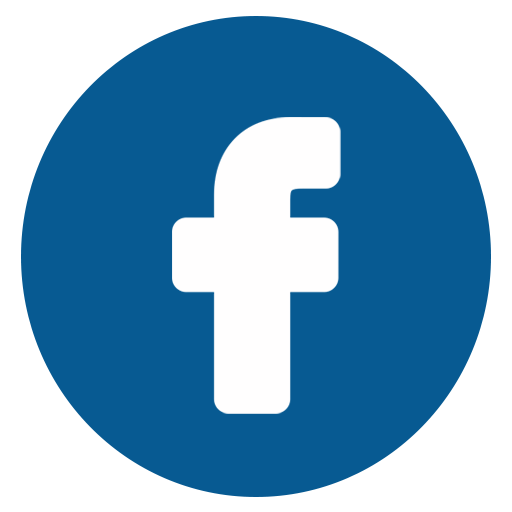
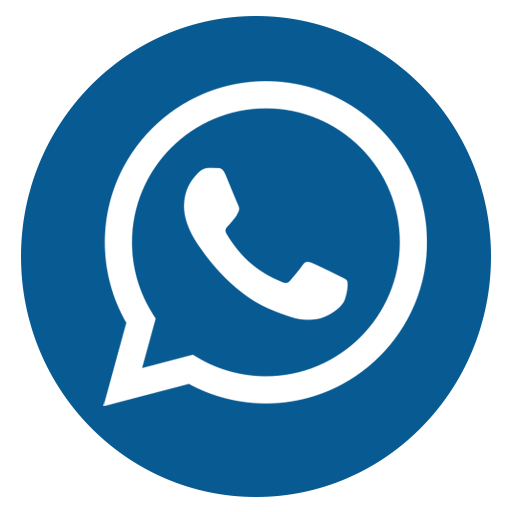
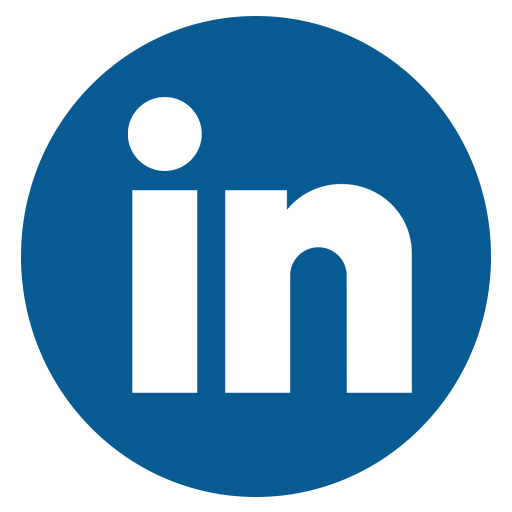
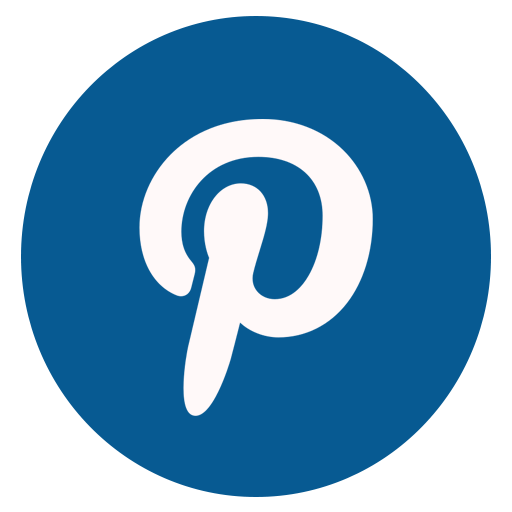
The tiny, wireless sensor uses machine learning to determine if the psychophysiological signs detected in real time by respiration and electrocardiogram (ECG) are consistent with opioid cravings. Such cravings are one of the primary causes of OUD relapse and fatal overdose following a period of abstinence.
Once a craving is detected, the sensor alerts the user with mindfulness-based interventions that ultimately could be personalized based on the user’s behaviors and input from their clinician.
“Nothing like this exists today,” Rahman says, “and we believe that mobile technologies can provide an effective mechanism for people with addiction to monitor their condition and manage their cravings better.”
The current project evolved from research published in 2019 by Rahman, lead author Bhanu Teja Gullapalli – a Ph.D. student working in Rahman’s lab – and others on the use of cardiac and respiratory signals to sense cocaine craving, euphoria and drug-seeking behavior.
Advertisement
The research can be expanded to include other substance use disorders, and the NSF-funded project includes a machine-learning course for clinicians and educational and awareness workshops for middle-school girls.
Advertisement
Source-Eurekalert