New computational tool named CopyKAT-based on from single-cell RNA-sequencing data, enables researchers to gain new insights when analyzing solid tumor samples.
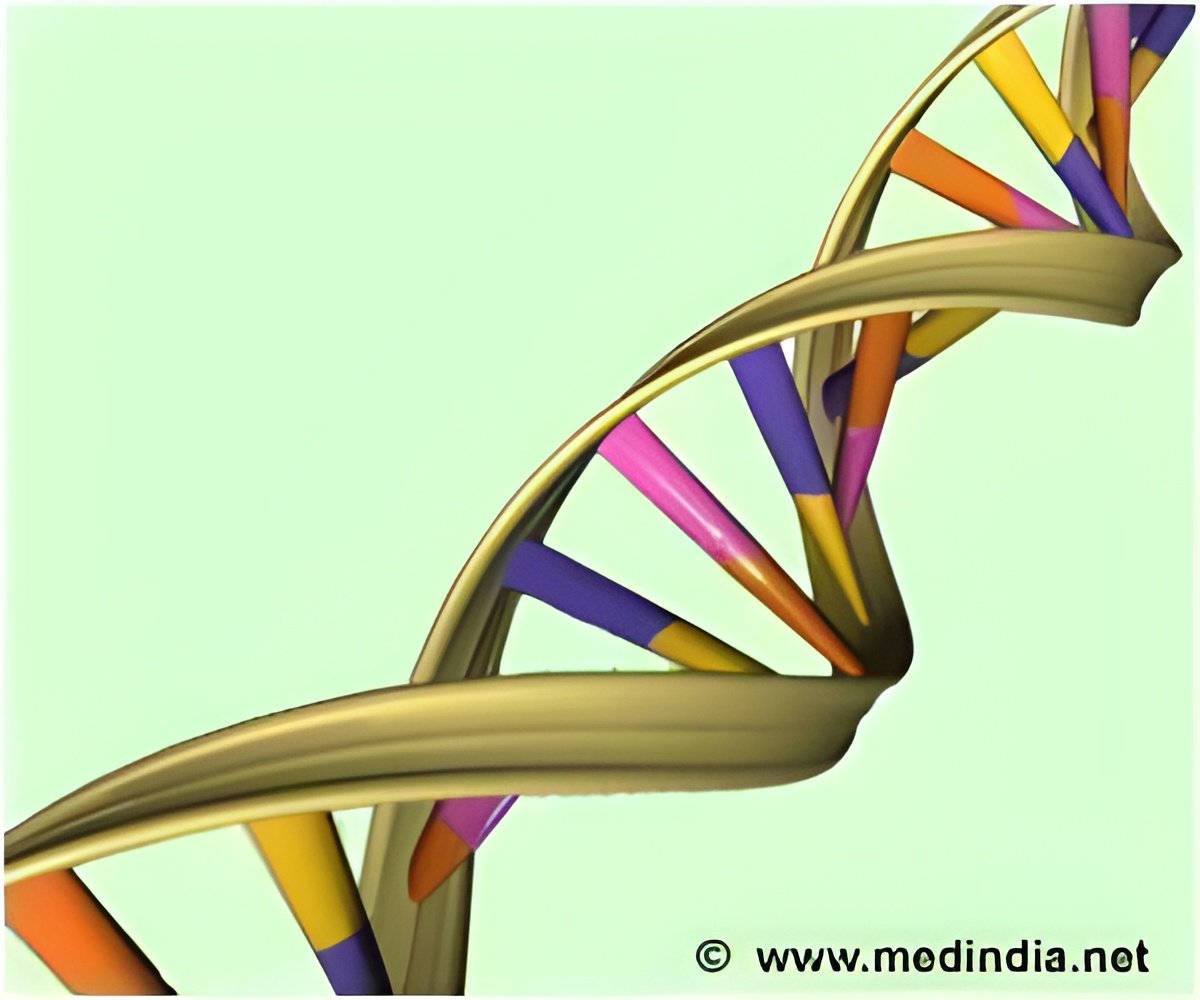
‘CopyKAT uses gene expression data to look for aneuploidy, or the presence of abnormal chromosome numbers, which is common in most cancers. It also helps to identify distinct subpopulations, or clones, within the cancer cells.’
Read More..
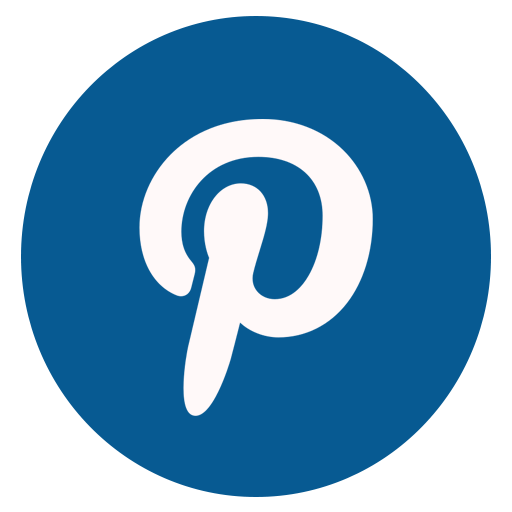
CopyKAT uses that gene expression data to look for aneuploidy, or the presence of abnormal chromosome numbers, which is common in most cancers, said study senior author Nicholas Navin, Ph.D., associate professor of Genetics and Bioinformatics & Computational Biology. The tool also helps to identify distinct subpopulations, or clones, within the cancer cells.Read More..
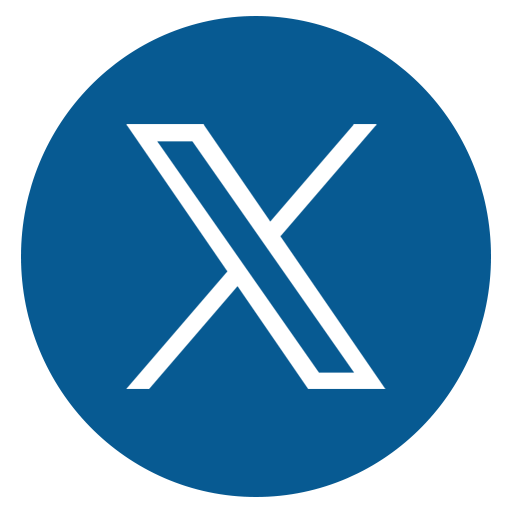
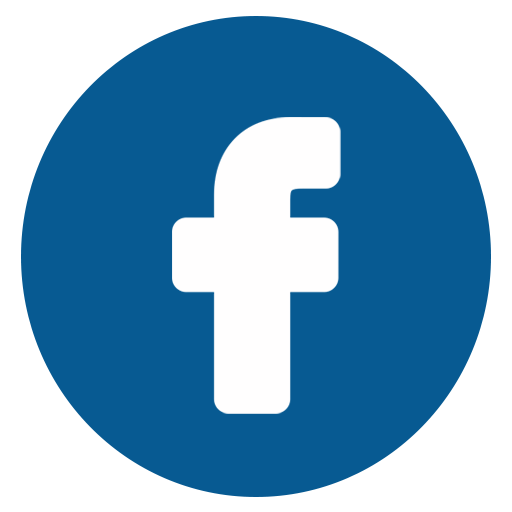
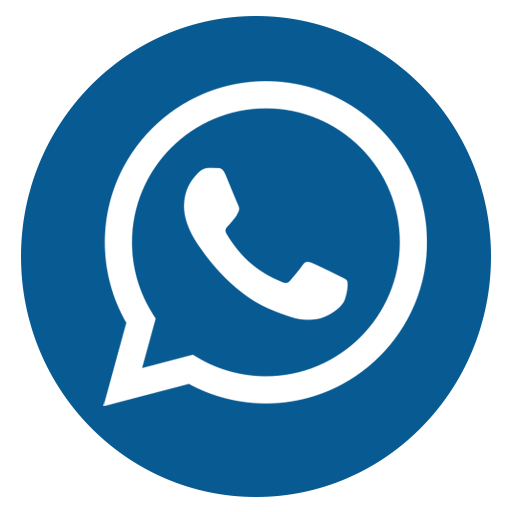
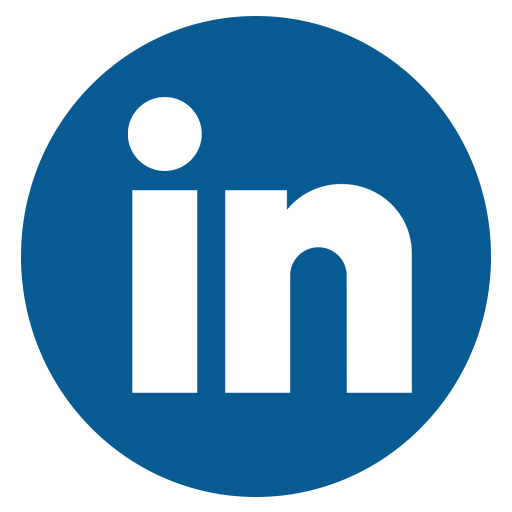
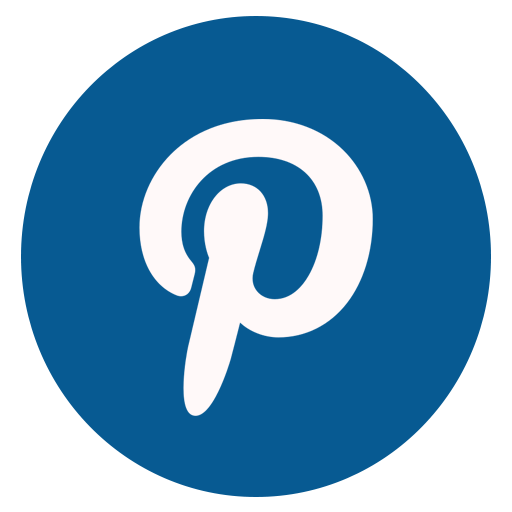
"We developed CopyKAT as a tool to infer genetic information from the transcriptome data. By applying this tool to several datasets, we showed that we could unambiguously identify, with about 99% accuracy, tumor cells versus the other immune or stromal cells present in a mixed tumor sample," Navin said. "We could then go one step further to discover the subclones present and understand their genetic differences."
Historically, tumors have been studied as a mixture of all cells present, many of which are not cancerous. The advent of single-cell RNA sequencing in recent years has enabled researchers to analyze tumors in much greater resolution, examining the gene expression of each individual cell to develop a picture of the tumor landscape, including the surrounding microenvironment.
However, it's not easy to distinguish between cancer cells and normal cells without a reliable computational approach, Navin explained. Former postdoctoral fellow Ruli Gao, Ph.D., now assistant professor of Cardiovascular Sciences at Houston Methodist Research Institute, developed the CopyKAT algorithms, which improve upon older techniques by increasing accuracy and adjusting for the newest generation of single-cell RNA-sequencing data.
The team first benchmarked its tool by comparing results to whole-genome sequencing data, which showed high accuracy in predicting copy number changes. In three additional datasets from pancreatic cancer, triple-negative breast cancer and anaplastic thyroid cancer, the researchers showed that CopyKAT was accurate in distinguishing between tumor cells and normal cells in mixed samples.
Advertisement
In analyzing these samples, the researchers also showed the tool is effective in identifying subpopulations of cancer cells within the tumor based on copy number differences, as confirmed by experiments in triple-negative breast cancers.
Advertisement
The tool is freely available to researchers here. The authors note that the tool is not applicable to the study of all cancer types. Aneuploidy, for example, is relatively rare in pediatric and hematologic cancers.
Source-Eurekalert