Cardiac imaging can be provided on the go using ultrasound-based wearable sensors according to a new study.
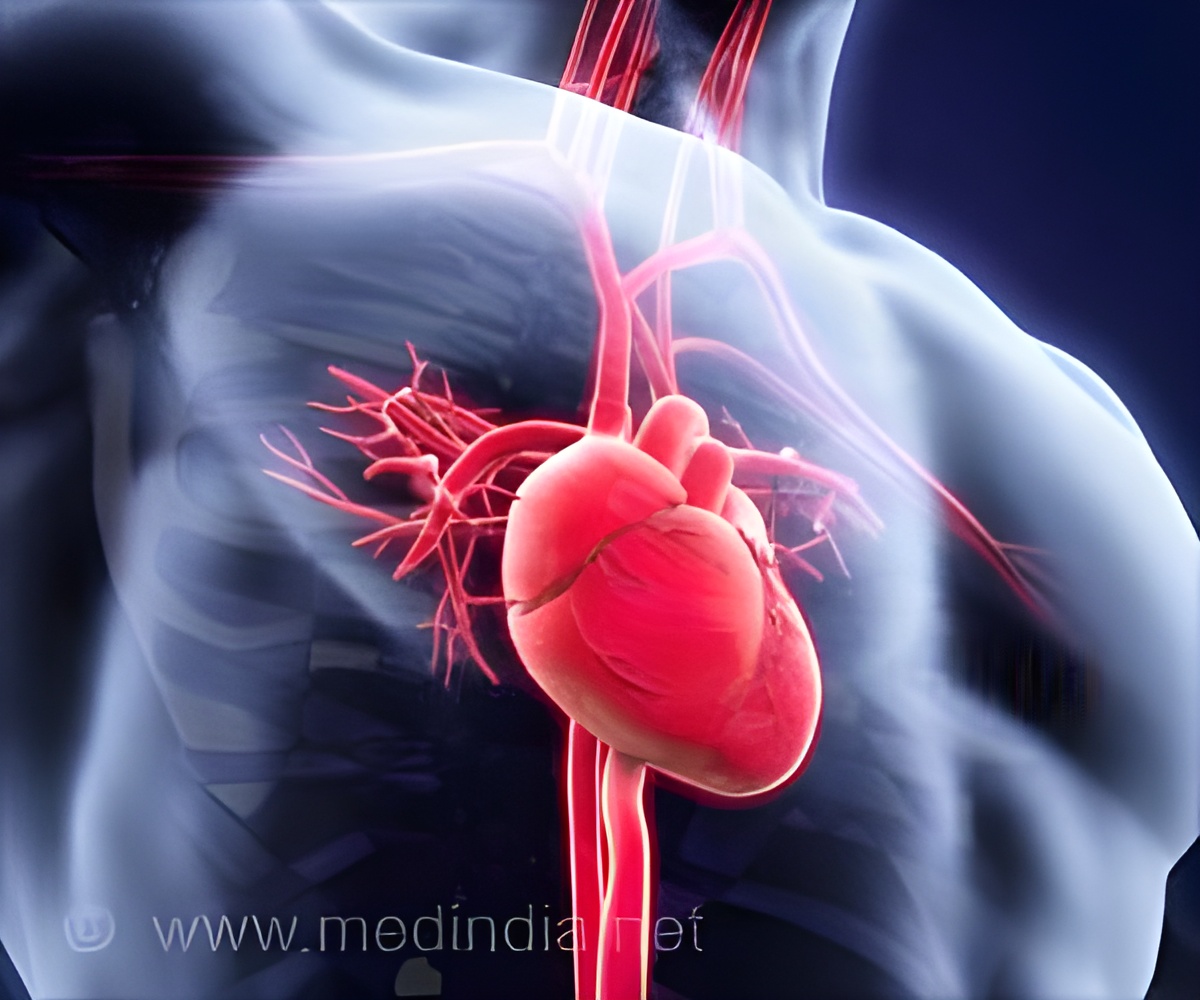
The goal is to make ultrasound more accessible to a larger population, said Sheng Xu, a professor of nanoengineering at the University of California San Diego, who is leading the project. Currently, echocardiograms– ultrasound examinations for the heart– require highly trained technicians and bulky devices.
“The technology enables anybody to use ultrasound imaging on the go,” Xu said.
Thanks to custom AI algorithms, the device is capable of measuring how much blood the heart is pumping. This is important because the heart not pumping enough blood is at the root of most cardiovascular diseases. And issues with heart function often manifest only when the body is in motion.
‘Development of a powerful new ultrasound sensor system for cardiac imaging that even works during a workout has been now designed by scientists.
’
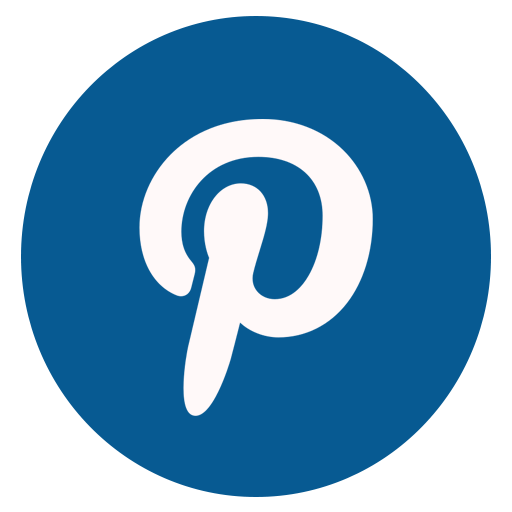
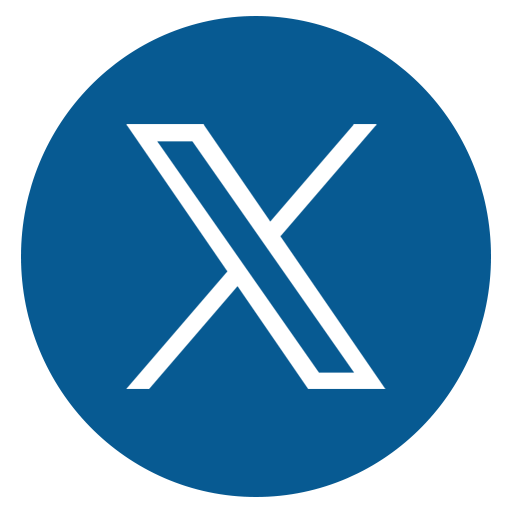
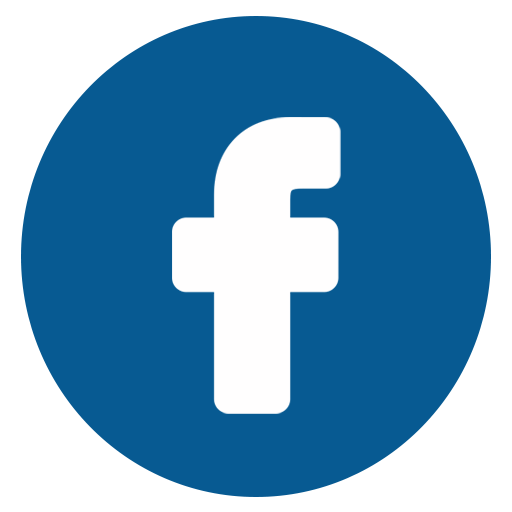
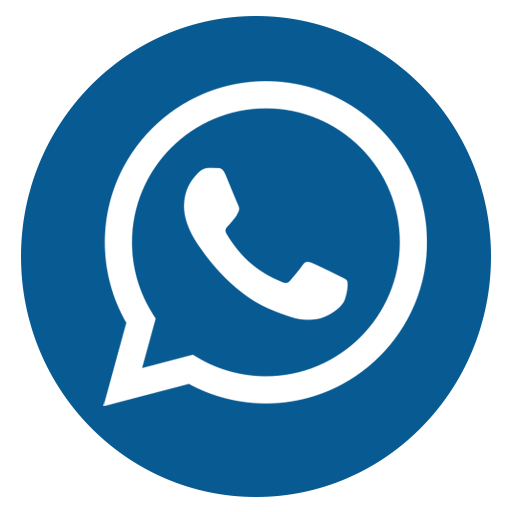
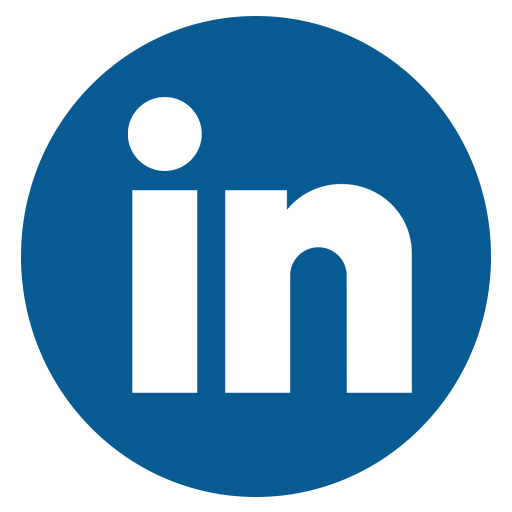
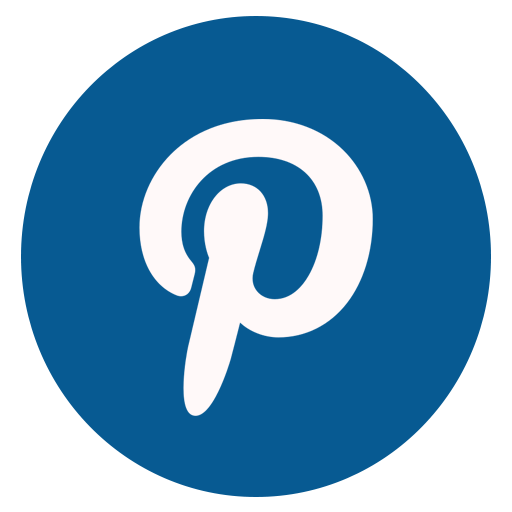
Advertisement
Cardiac Imaging for Heart Monitoring
Cardiac imaging is an essential clinical tool to assess long-term heart health, detect problems as they arise, and care for critically ill patients. This new wearable, non-invasive heart monitor for humans provides real-time, automated insights on the difficult-to-capture pumping activity of the heart, even when a person is exercising.The wearable heart monitoring system uses ultrasound to continuously capture images of the four chambers of the heart from different angles, and analyze a clinically relevant subset of the images in real-time using custom-built AI technology. The project builds on the team's previous advances in wearable imaging technologies for deep tissues.
“The increasing risk of heart diseases calls for more advanced and inclusive monitoring procedures,” Xu said. “By providing patients and doctors with more thorough details, continuous and real-time cardiac image monitoring is poised to fundamentally optimize and reshape the paradigm of cardiac diagnoses.”
In comparison, existing non-invasive methods have limited sampling capabilities and provide limited data. The wearable technology developed by Xu’s team enables safe, non-invasive, and high-quality cardiac imaging, resulting in images with high spatial resolution, temporal resolution, and contrast.
“It also minimizes patient discomfort and overcomes some limitations of noninvasive technologies such as CT and PET, which could expose patients to radiation,” said Hao Huang, a PhD student in the Xu group at UC San Diego.
The unique design of the sensor makes it ideal for bodies in motion. “The device can be attached to the chest with minimal constraint to the subjects’ movement, even providing a continuous recording of cardiac activities before, during and after exercise,” said Xiaoxiang Gao, a postdoctoral researcher in the Xu group at UC San Diego.
Advertisement
The Importance of Cardiac Imaging
Cardiac diseases are the leading cause of death among the elderly and are also becoming more prevalent among the young due to lifestyle factors. The signs of cardiac diseases are transient and unpredictable, making them hard to spot.This has upped the demand for more advanced, inclusive, non-invasive, and cost-effective monitoring technologies such as long-term cardiac imaging, which this wearable device facilitates.
Cardiac imaging is one of the most powerful tools for screening and diagnosing cardiac issues before they become problems. “The heart undergoes all kinds of different pathologies,” said Hongjie Hu, a postdoctoral researcher in the Xu lab at UC San Diego. “Cardiac imaging will disclose the true story underneath. Whether it be that a strong but normal contraction of heart chambers leads to the fluctuation of volumes, or that a cardiac morphological problem has occurred as an emergency, real-time image monitoring on the heart tells the whole picture in vivid detail and visual effect.”
Advertisement
How it Works in Detail
The new system gathers information through a wearable patch as soft as human skin, designed for optimal adherence. The patch measures 1.9 cm (L) x 2.2 cm (W) x 0.09 cm (T), about the size of a postage stamp.It sends and receives the ultrasound waves which are used to generate a constant stream of images of the structure of the heart in real-time. This ultrasound patch is soft and stretchable, and it adheres well to human skin, even during exercise.
The system can examine the left ventricle of the heart in separate bi-plane views using ultrasound, generating more clinically useful images than were previously available. As a use case, the team demonstrated imaging of the heart during exercise, which is not possible with the rigid, cumbersome equipment used in clinical settings.
The performance of the heart is characterized by three factors: stroke volume (the volume of blood the heart pumps out each beat), ejection fraction (the percentage of blood pumped out of the left ventricle of the heart every beat), and cardiac output (the volume of blood the heart pumps out every minute).
Xu’s team developed an algorithm to facilitate continuous, AI-assisted automatic processing.
“A deep learning model automatically segments the shape of the left ventricle from the continuous image recording, extracting its volume frame-by-frame and yielding waveforms to measure stroke volume, cardiac output and ejection fraction,” said Mohan Li, a master’s student in the Xu group at UC San Diego.
“Specifically, the AI component involves a deep learning model for image segmentation, an algorithm for heart volume calculation, and a data imputation algorithm,” said Ruixiang Qi, a master’s student in the Xu group at UC San Diego.
“We use this machine learning model to calculate the heart volume based on the shape and area of the left ventricle segmentation. The imaging-segmentation deep learning model is the first to be functionalized in wearable ultrasound devices. It enables the device to provide accurate and continuous waveforms of key cardiac indices in different physical states, including static and after exercise, which has never been achieved before.”
Thus, this technology can generate curves of these three indices continuously and noninvasively, as the AI component processes the continuous stream of images to generate numbers and curves.
To create the platform, the team faced some technical challenges that required careful decision-making. To produce the wearable device itself, the researchers used a piezoelectric 1-3 composite bonded with Ag-epoxy backing as the material for transducers in the ultrasound imager, reducing risk and improving efficiency over previous methods.
When choosing the transmission configuration of the transducer array, they achieved superior results through wide-beam compounding transmission. They also selected nine popular models for machine-learning-based image segmentation, landing on FCN-32, which achieved the highest possible accuracy.
In the current iteration, the patch is connected through cables to a computer, which can download the data automatically while the patch is still on. The team has developed a wireless circuit for the patch, which will be covered in a forthcoming publication.
Next Steps
Xu plans to commercialize this technology through Softsonics, a company spun off from UC San Diego that he cofounded with engineer Shu Xiang. He also encourages others in his scientific community to follow his lead and work on areas of this research that warrant further exploration.To follow up on these results, Xu recommends four immediate next steps:
- B-mode imaging, which allows more diagnostic capabilities involving different organs
- The design of the soft imager, which allows researchers to fabricate large transducer probes that cover multiple positions simultaneously
- Miniaturization of the back-end system that powers the soft imager
- Working toward a general machine learning model that fits more subjects
Source-Eurekalert