Medical hematologist and oncologist Aziz Nazha had developed a personalized prediction model for a type of bone marrow cancer which is effective than the existing prediction models.
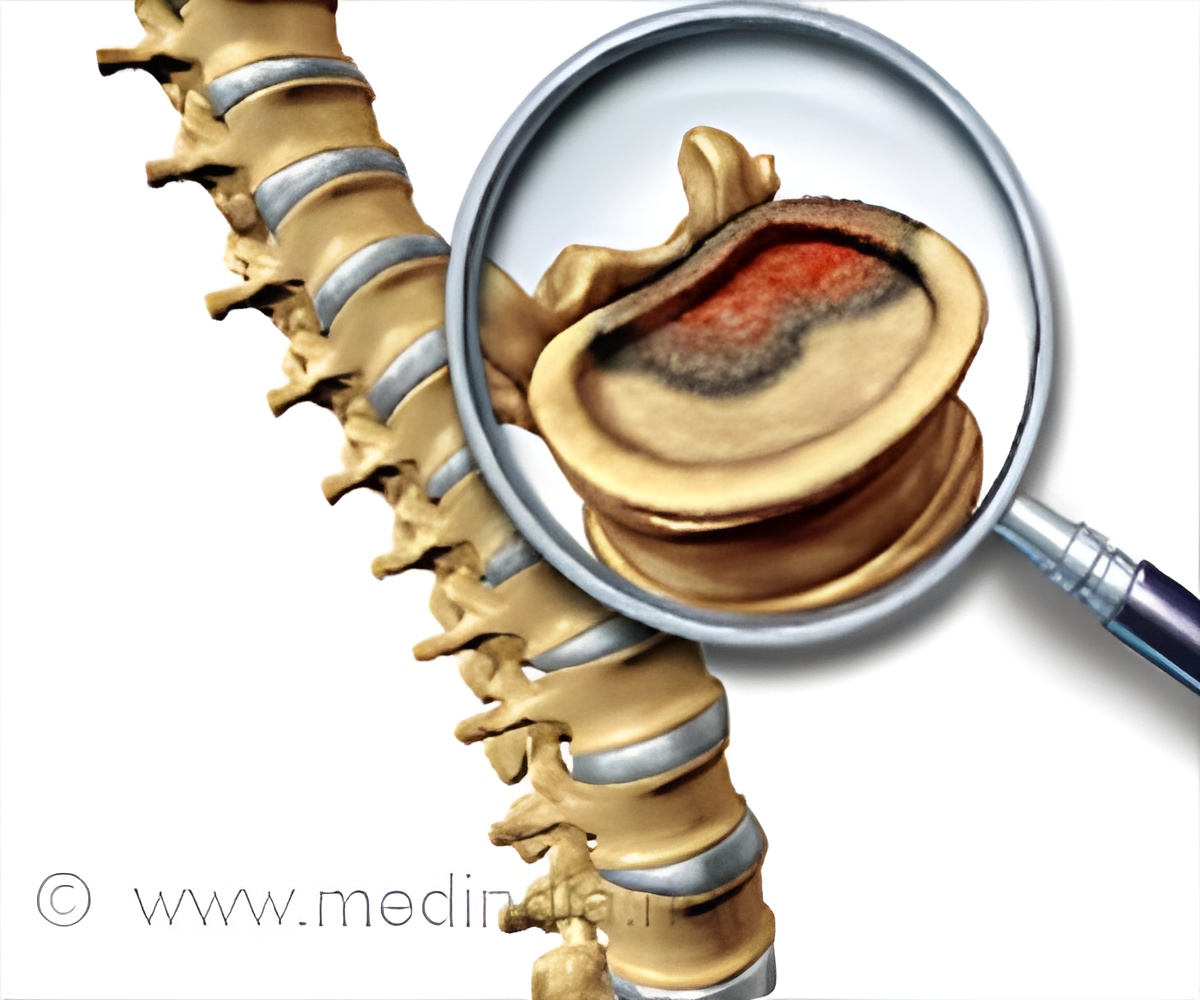
‘The personalized prediction tool will help in better and accurate prediction of the cancer patient's risk of mortality and the right amount of treatment can be given accordingly.’
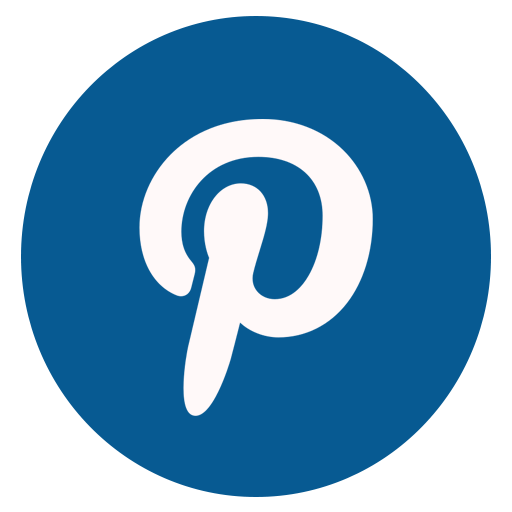
Patients with MDS have survival outcomes that can range from months to decades. Although several prognostic scoring systems have been developed to risk stratify MDS patients, survival varies even within distinct categories, which may lead to over- or under-treatment. Researchers hypothesized that discrepancies may be due to analytic approaches or lack of incorporation of molecular data. 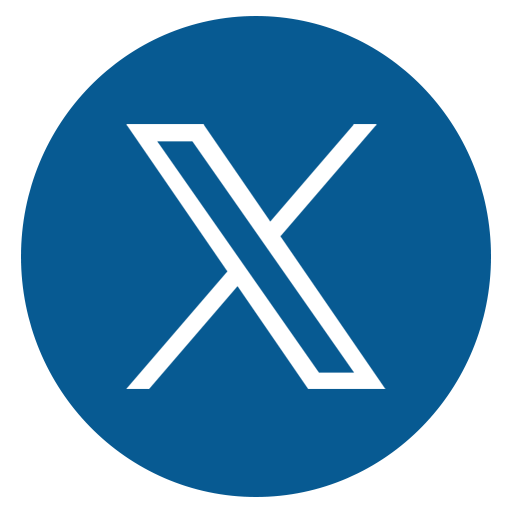
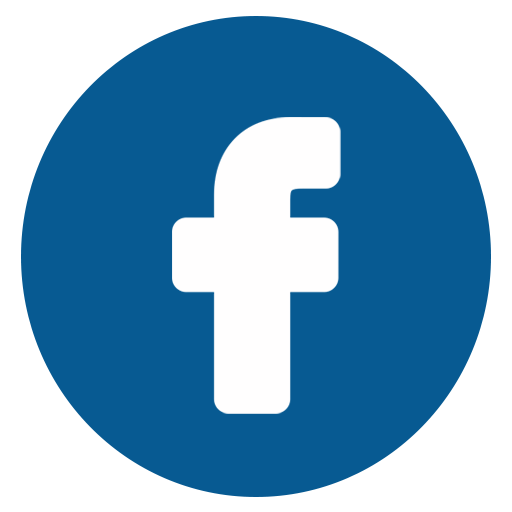
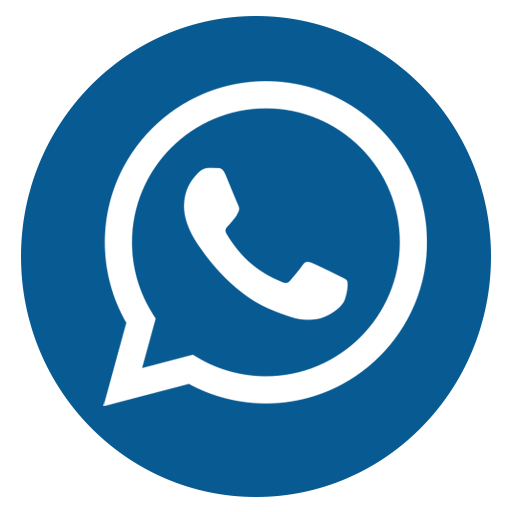
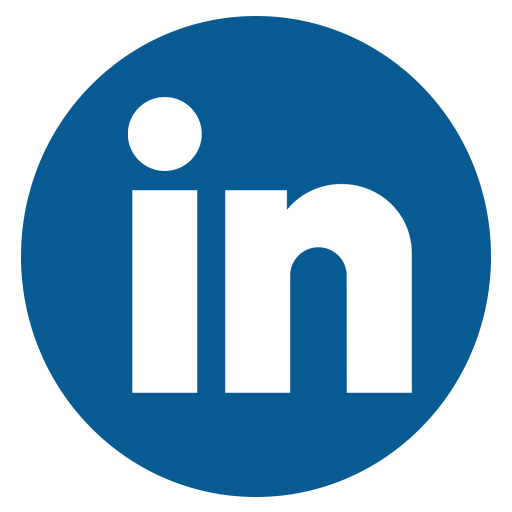
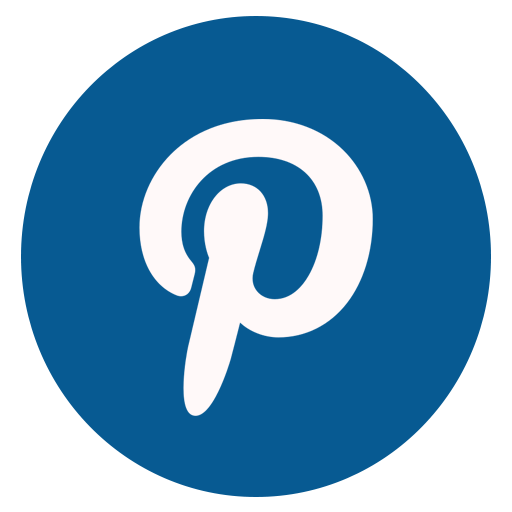
"Determining prognosis in oncology is one of the most important parts of our job. All oncology patients want to know how long they are going to live," said Dr. Nazha. "Too often we find a significant gap between what we predicted, based on existing prediction models, and what actually happened to our patients."
The new model designed by Dr. Nazha and team incorporates individual patient genomic and clinical data using a machine-learning algorithm to better predict survival probabilities and outcomes. Patients' clinical and mutational variables are entered into a web application that runs the personalized prediction model and provides overall survival and AML transformation probabilities at different time points that are specific for a patient. Patient data used to test the model came from Cleveland Clinic and Munich Leukemia Laboratory and was validated in a separate collection of patient data from Moffitt Cancer Center.
In a comparison using medical records, the new model predicted a patient's likelihood of surviving for a given period of time 74 percent of the time, compared to IPSS-R accuracy of 67 percent. The model accurately predicted a patient's risk of AML 80 percent of the time, compared to IPSS-R accuracy of 73 percent.
Dr. Nazha's next step is to build a website where clinicians can input a patient's clinical and genetic characteristics and get back the patient's probability of surviving at different time points such as six months, 12 months, and 18 months.
Advertisement
Dr. Nazha also presented "A Personalized Prediction Model for Outcomes after Allogeneic Hematopoietic Stem Cell Transplant in Patients with MDS: On Behalf of the CIBMTR Chronic Leukemia Committee" at ASH on Saturday, Dec. 1.
Advertisement
Investigators used data from MDS patients enrolled in the Center for International Blood and Marrow Transplant Research Registry and built a web application where patients' genomic and clinical data are computed to predict survival probability after HCT at 6,12 and 24 months. The application identified several clinical and molecular variables that impacted overall survival and the hazard of relapse. This information may aid physicians and patients in their decision prior to HCT.
"Because of the risks of transplant-related mortality and relapse, identifying patients who may or may not benefit from HCT is clinically important," said Dr. Nazha. "Understanding survival probability at different points in time may aid physicians and patients in their approach, prior to HCT."
Source-Eurekalert