Identifying several predictive factors for scoring the Alzheimer’s Disease risk using machine learning models can prevent recurrence or mitigate their adverse effects.
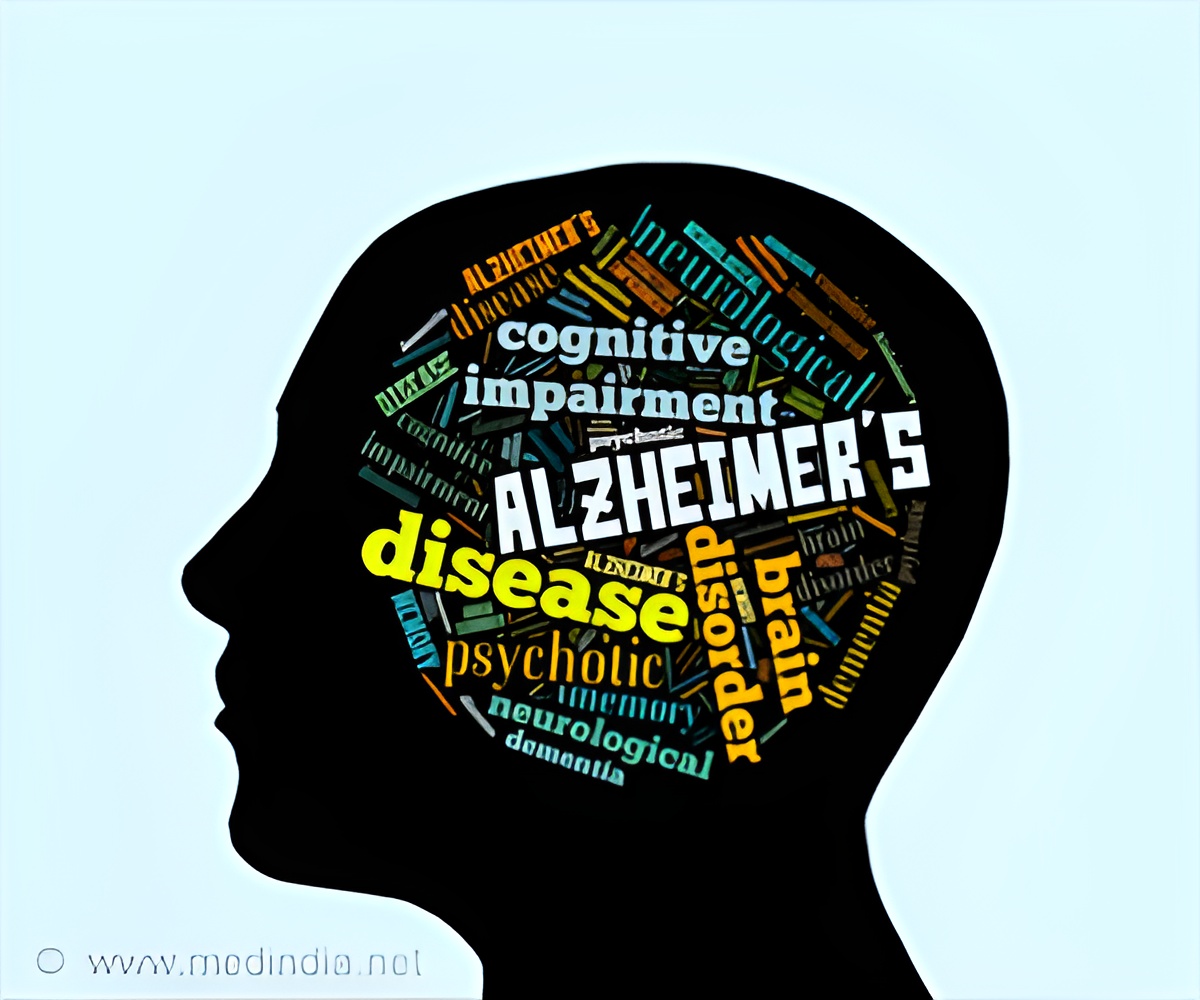
Artificial Intelligence for Alzheimer’s Disease: Promise or Challenge?
In this study, researchers used the machine learning models to rank predictive risk factors for two populations from the UK Biobank: White individuals aged 40 and older, and a subset of those adults who were 65 or older.‘The machine learning model improved the accuracy of Alzheimer’s disease risk prediction by exploring various predictors and new feature patterns.’
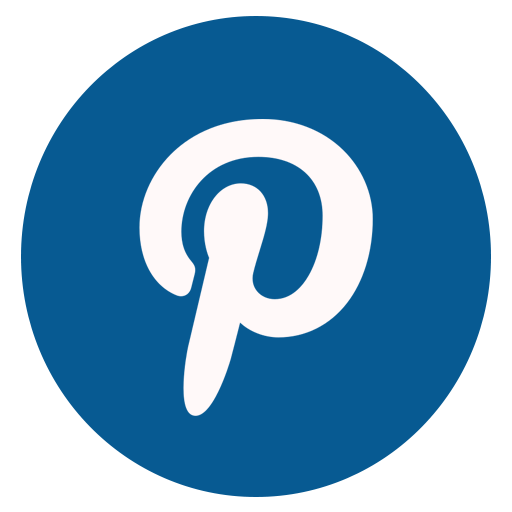
The results showed that age – which constitutes one-third of total risk by age 85, according to the Alzheimer’s Association – was the biggest risk factor for Alzheimer’s in the entire population, but for the older adults, genetic risk as determined by a polygenic risk score was more predictive.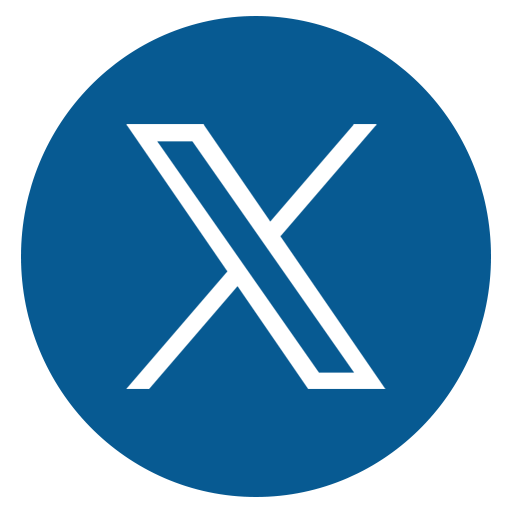
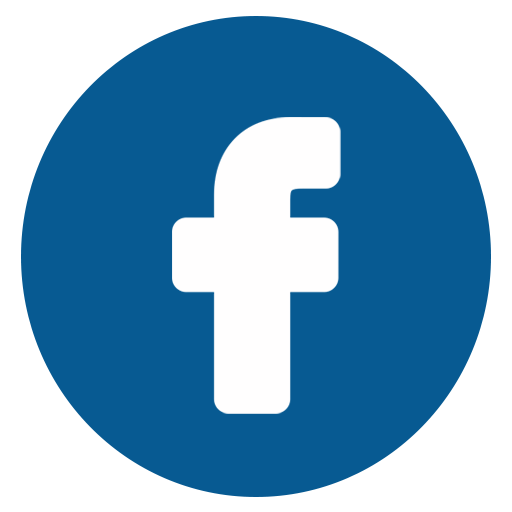
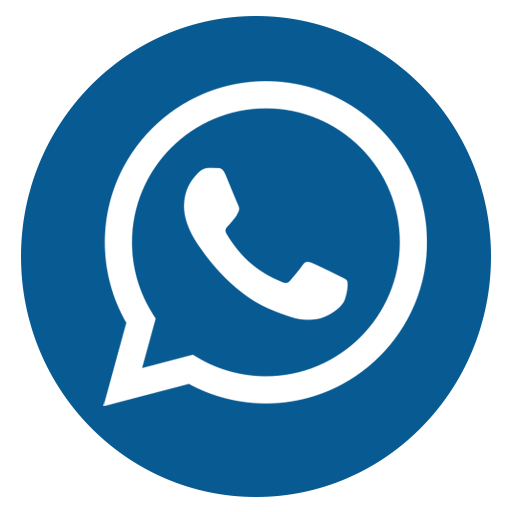
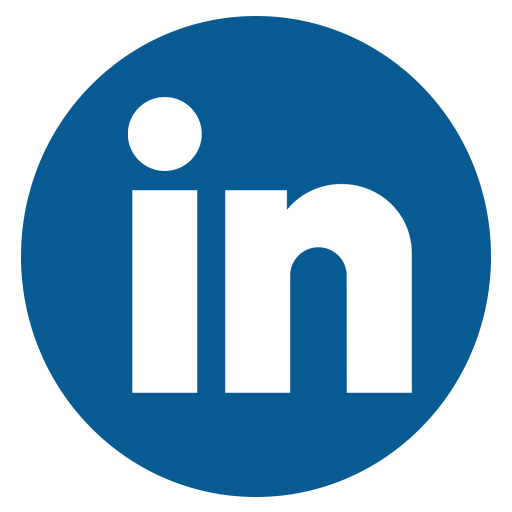
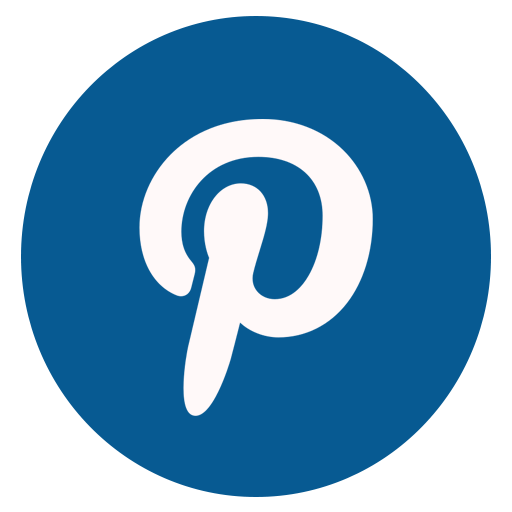
A low household income also emerged as an important risk factor, ranking either third or fourth after the effects of age and genetics. The finding related to income was very interesting. Income can be such an important factor to decide what you can afford to eat, where you can afford to live, your education level, and access to care. All of these possibly contribute to Alzheimer’s disease.
Of the 457,936 UK Biobank participants in the sample, 2,177 individuals had developed Alzheimer’s disease and 455,759 had not, and 88,309 were 65 or older. A few non-genetic risk factors that differed between people with and without Alzheimer’s disease (AD) stood out.
Results showed that in people with AD, higher systolic and lower diastolic blood pressure was more common, diabetes was more prevalent, household income and education were lower, and recent falls, hearing difficulty, and a mother’s history of having AD were higher.
Ranking the Alzheimer’s Disease Risk Factors
The top-20 list of risk factors for the full sample of adults also included diagnoses of high blood pressure, urinary tract infection, depressive episodes, fainting, unspecified chest pain, disorientation, and abnormal weight loss.Other risk factors in the top 20 for people 65 and older included high cholesterol and gait abnormalities. These findings showed the power of adding condition codes from electronic health records to the models.
Advertisement
The separate collections of variants were used to establish two polygenic risk scores, which aggregate genetic effects across the genome into a single measure of risk for each individual.
Advertisement
Being born with a genetic risk for disease already established, the information about how other health and socioeconomic factors affect our risk gives us the power to take preventive measures. constructing models to make these predictions could also help with drug development and effective and low-cost screening programs.
Source-Eurekalert