Researchers have always puzzled as to how immune cells manage to sort through vast numbers of similar-looking proteins within the body to detect foreign invaders and fight infections.
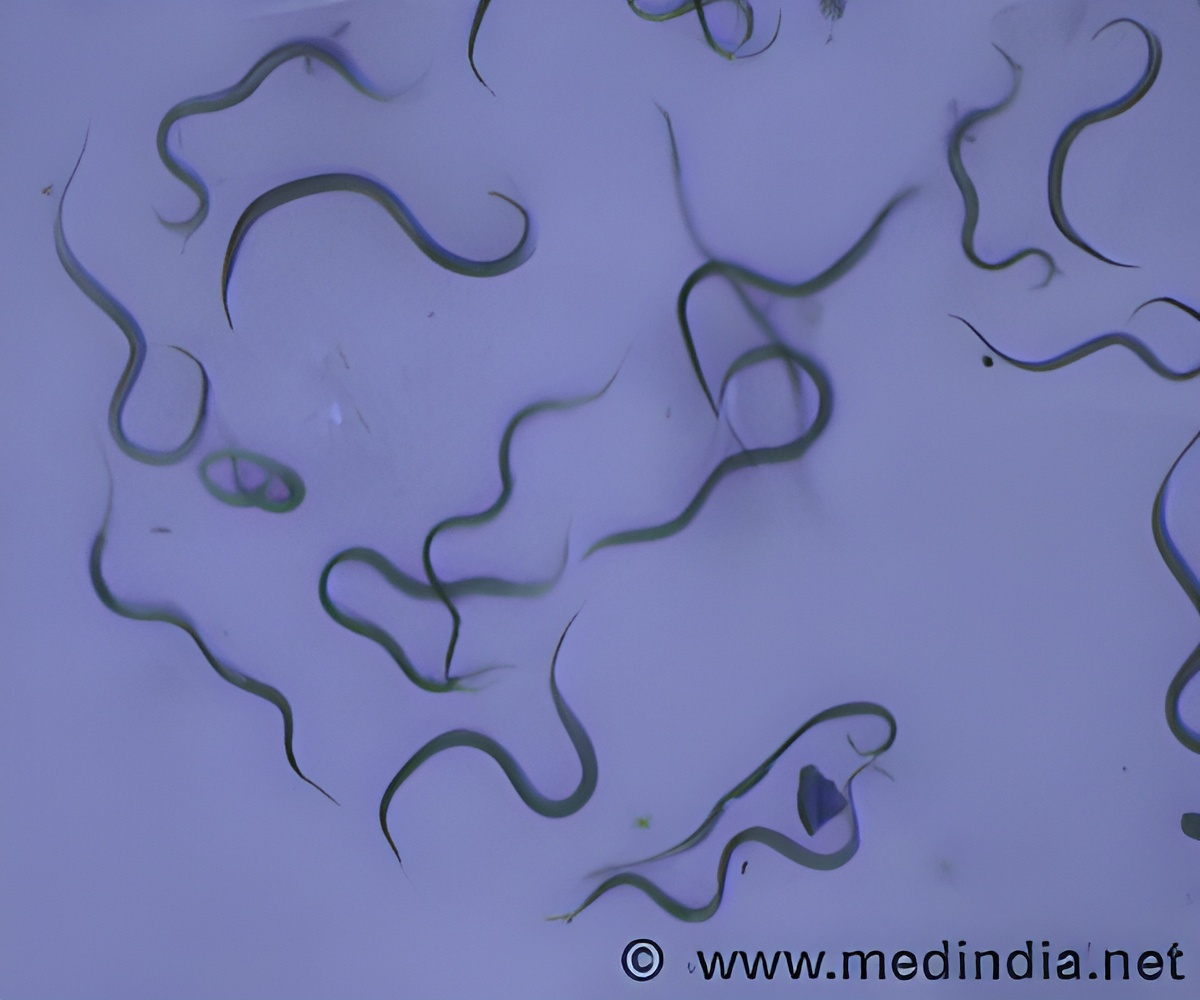
Understanding how immune cells tackle this formidable challenge is important, because it could provide crucial insights into the understanding of immune diseases, from AIDS to auto-immune disorders.
In a study published May 21 in the journal Physical Review Lettters, François and McGill graduate student Jean-Benoît Lalanne used computational tools to examine what kind of solutions immune systems may use to detect small concentrations of foreign antigens (characteristic of potentially harmful infections) in a sea of "self-antigens" normally present at the surface of cells.
The researchers' computer simulations yielded a surprisingly simple solution related to the well-known phenomenon of biochemical adaptation – a general biochemical mechanism that enable organisms to cope with varying environmental conditions.
To find solutions, the computer uses an algorithm inspired by Darwinian evolution. This algorithm, designed previously within the François research group, randomly generates mathematical models of biochemical networks. It then scores them by comparing properties of these networks to predefined properties of the immune system. Networks with best scores are duplicated in the next generation and mutated, and the process is iterated over many simulated "generations" until networks reach a perfect score.
In this case, almost all solutions found were very similar, sharing a common core structure or motif.
Advertisement
Advertisement