Twitter users with anxiety and depression are more likely to post images with lower aesthetic values and less vivid colors, especially images in grayscale.
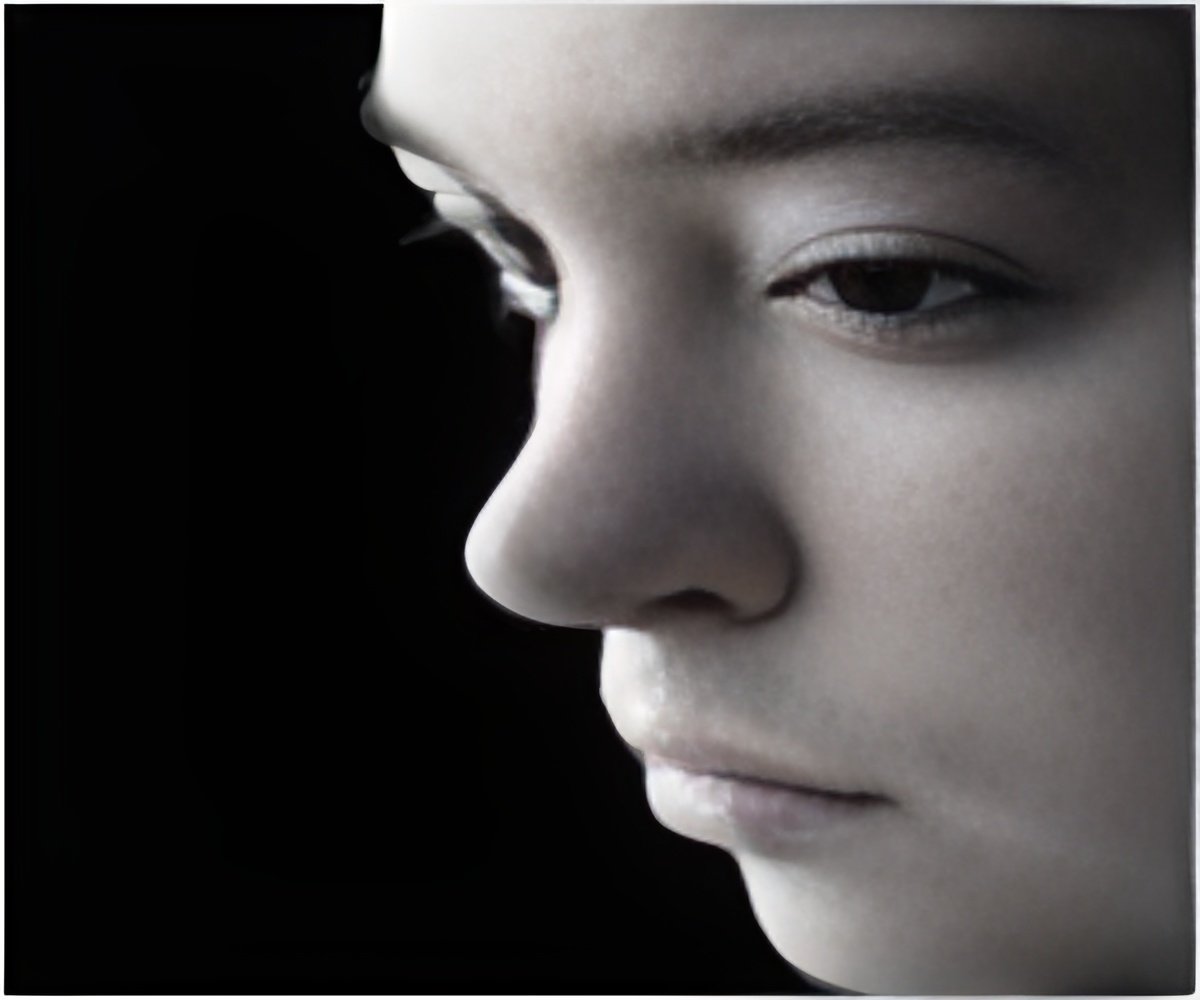
‘Photos posted by depressed Twitter users are mostly lower in aesthetic value and with less bright colors.’
Read More..
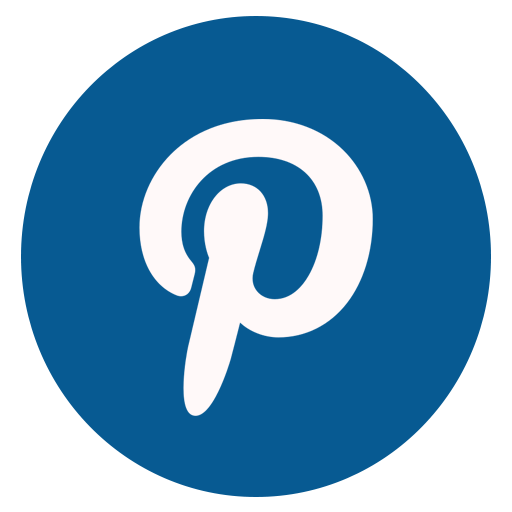
The study sought to utilize computer vision and artificial intelligence to determine what qualities of photos posted to and set as profile images on Twitter could be associated with depression and anxiety, with an eye toward using the platform as a method of screening for both. Read More..
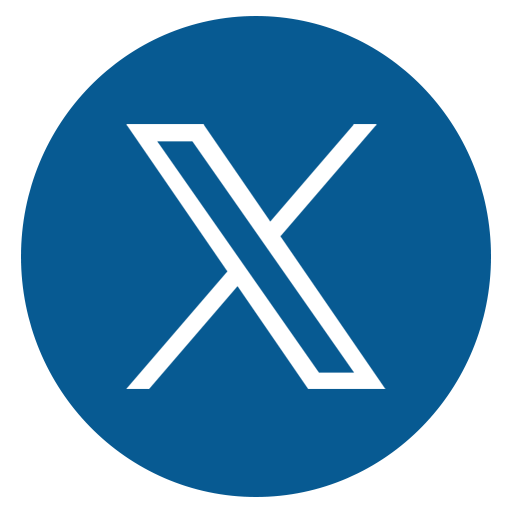
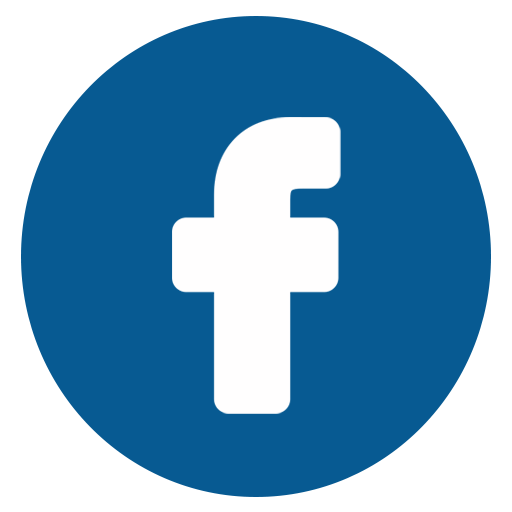
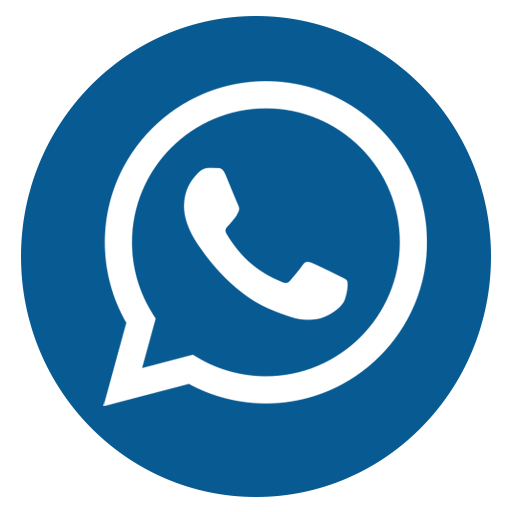
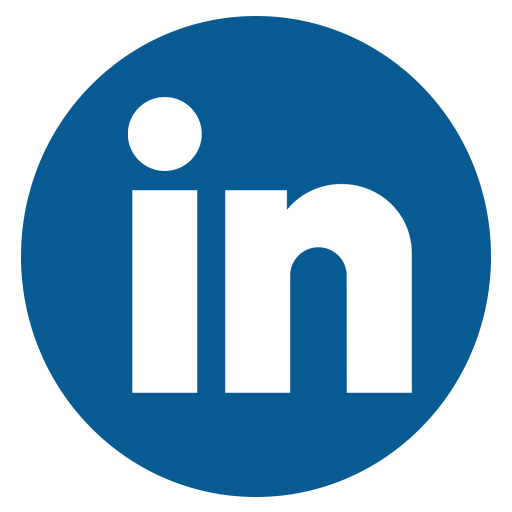
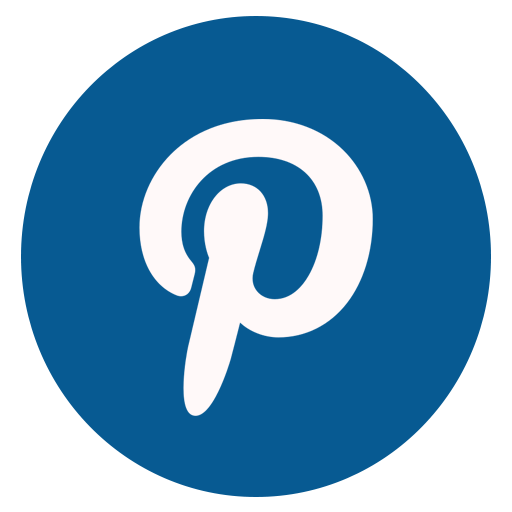
In 2018, Penn Medicine researchers found that depression could be predicted as much as three months before diagnosis by using artificial intelligence to identify keywords that flagged certain users. As social media is becoming increasingly image-focused more than half of all tweets, 3,000-plus of them per second, now contain an image the value in gaining clues on health conditions through image content will become increasingly valuable to medicine.
"While the association between depression and language-use patterns is well-studied, the visual aspects of depression has not been," said the study's lead author, Sharath Guntuku, Ph.D., a research scientist with Penn Medicine's Center for Digital Health.
"It is challenging to transform pixels that form the images to interpretable features, but with the advances in computer vision algorithms, we are now attempting to uncover another dimension of the condition as it manifests online."
The researchers used algorithms to extract features such as colors, facial expressions, and different aesthetic measures (such as depth of field, symmetry, and lighting) from images posted by more than 4,000 Twitter users who consented to be a part of the study. To quickly categorize their depression and anxiety scores, they analyzed each person's last 3,200 tweets. Meanwhile, 887 users also completed a traditional survey to obtain depression and anxiety scores. Then, image features were correlated with users' depression and anxiety scores. From this, several significant relationships emerged.
Advertisement
"Depression is often accompanied by 'flat affect,' which is characterized by reduced expression and displays of emotion," Guntuku said. "The condition also takes a toll on one's appeal toward hobbies or games activities in general that are otherwise usually enjoyed."
Advertisement
"This tool is far from perfect to be used as a diagnostic tool. However, an automated machine learning tool could be a low-cost method for clinicians, with permission from their patients, to monitor their accounts and potentially detect elevated depression or anxiety levels, Guntuku explained. "The clinicians could then refer patients who were flagged by the tool for more formal screening methods."
The study's senior author, Lyle Ungar, Ph.D., a professor of Genomics and Computational Biology and Psychology, said that there are great possibilities beyond just Twitter, and anxiety and depression, too.
"Something like this could be applied to Instagram and text messaging, too," Ungar said. "We hope this may give some insights into the different facets of depression. And we're also looking at a variety of other conditions, from loneliness to ADHD."
Source-Eurekalert