Blood pressure may be predicted using new wearable off-the-shelf technology that can help give personalized advice to reduce the blood pressure based on the obtained data.
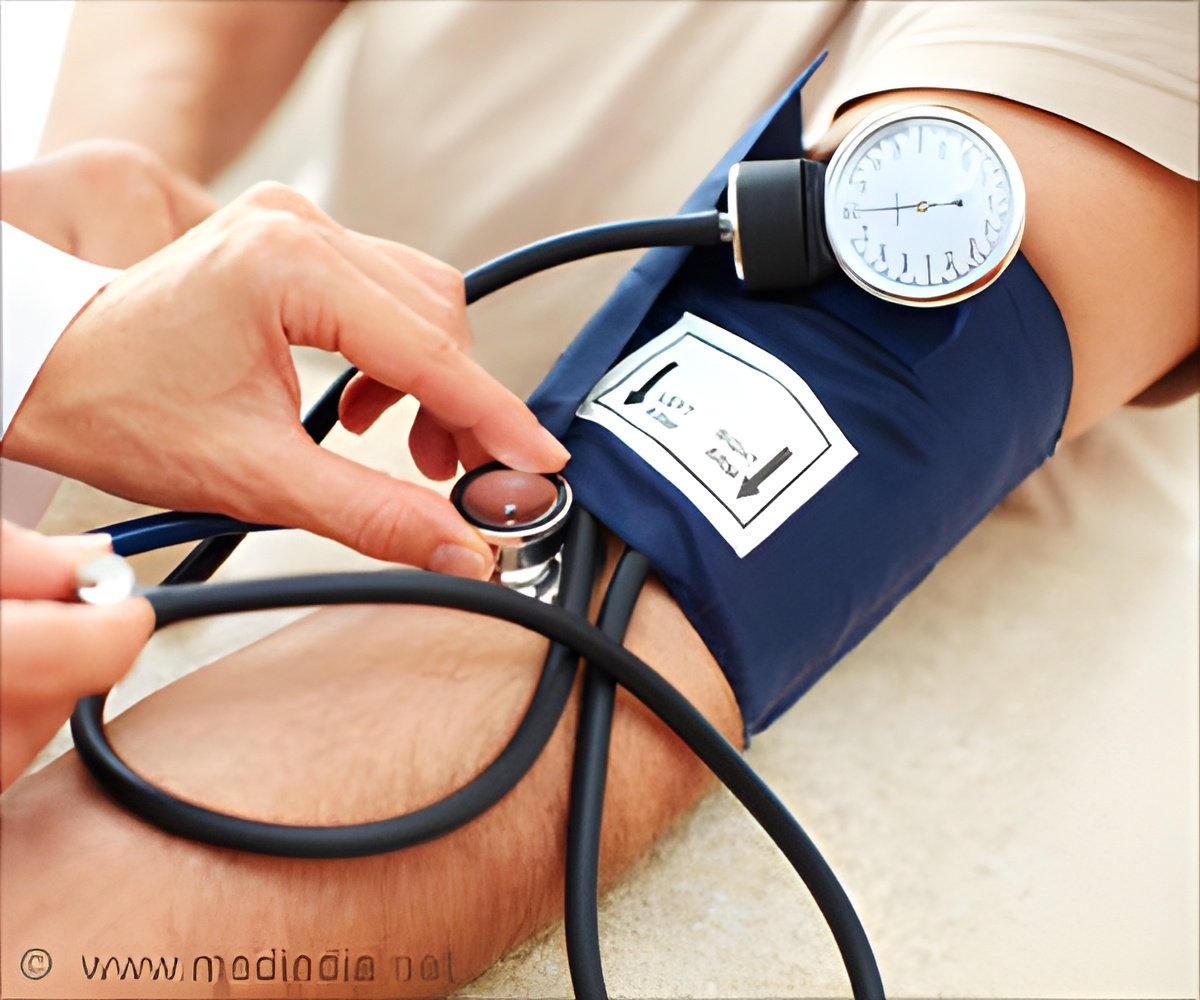
‘Blood pressure may be predicted using new wearable off-the-shelf technology that can help give personalized suggestions to decrease the blood pressure based on the obtained data.’
Read More..
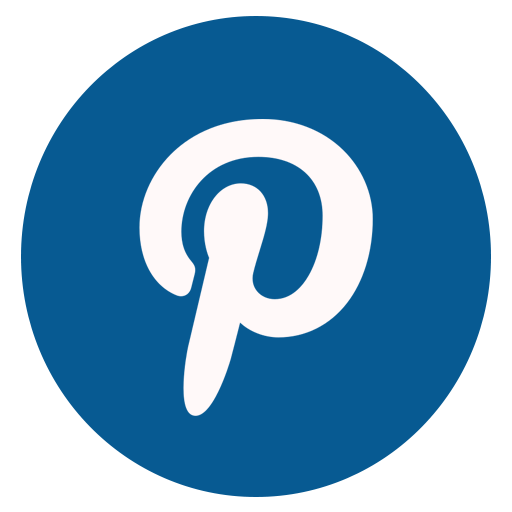
When doctors tell their patients to make a lot of significant lifestyle changes exercise more, sleep better, lower their salt intake, etc. it can be overwhelming, and compliance is not very high, said Sujit Dey, co-author of the paper and Director of the Center for Wireless Communications at UC San Diego's Jacobs School of Engineering where he's a professor in the Department of Electrical and Computer Engineering.Read More..
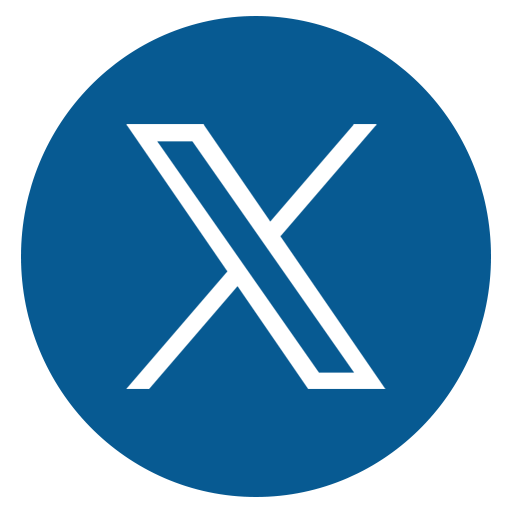
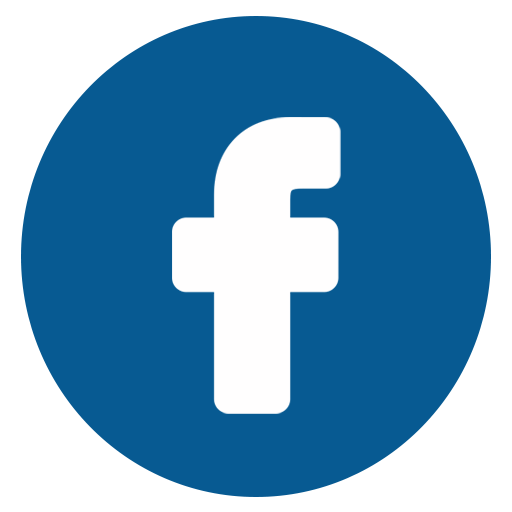
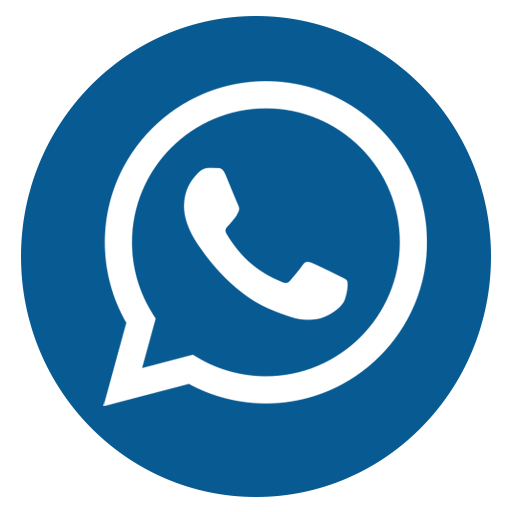
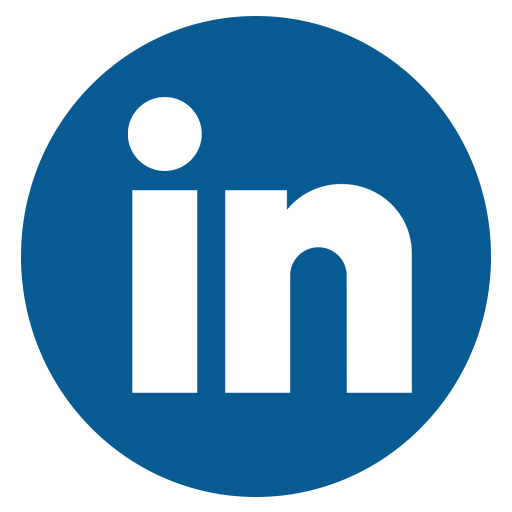
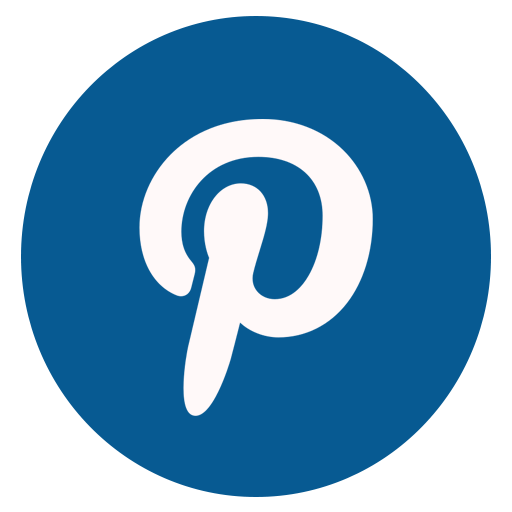
"What if we could pinpoint the one health behavior that most impacts an individual's blood pressure, and have them focus on that one goal, instead," Dey asked.
Dey and co-author Po-Han Chiang, a graduate student in the Mobile Systems Design Lab in the Department of Electrical and Computer Engineering at UC San Diego's Jacobs School of Engineering, collected sleep, exercise and blood pressure data from eight patients over 90 days using a FitBit Charge HR and Omron Evolv wireless blood pressure monitor. Using machine learning and this data from existing wearable devices, they developed an algorithm to predict the users' blood pressure and show which particular health behaviors affected it most.
This study affirmed the importance of personalized data over generalized information. While many health databases add large amounts of patient data into one model, considering all patients together to make health suggestions, the personalized information in this study was more effective. For example, one subject's blood pressure was most affected by the number of minutes they were sedentary throughout the day.
Changing that one factor had a significant impact, lowering their average systolic blood pressure by 15.4 percent and their diastolic blood pressure by 14.2 percent in one week. For another subject, the time they went to bed was the most important factor in lowering their blood pressure based on their historical data. When this subject went to bed a total of 58 minutes earlier over the week prior, they experienced a 3.6 percent drop systolic blood pressure and 6.6 percent decrease in their average diastolic blood pressure from the previous week.
Advertisement
"Instead of saying 'My blood pressure is high therefore I'll go to the doctor to get medicine,' giving patients and doctors access to this type of system can allow them to manage their symptoms on a continuous basis."
Advertisement
Source-Eurekalert